Knowledge-Based Weak Supervision for Information Extraction of Overlapping Relations.
HLT '11 Proceedings of the 49th Annual Meeting of the Association for Computational Linguistics: Human Language Technologies - Volume 1(2011)
摘要
Information extraction (IE) holds the promise of generating a large-scale knowledge base from the Web's natural language text. Knowledge-based weak supervision, using structured data to heuristically label a training corpus, works towards this goal by enabling the automated learning of a potentially unbounded number of relation extractors. Recently, researchers have developed multi-instance learning algorithms to combat the noisy training data that can come from heuristic labeling, but their models assume relations are disjoint --- for example they cannot extract the pair Founded(Jobs, Apple) and CEO-of(Jobs, Apple). This paper presents a novel approach for multi-instance learning with overlapping relations that combines a sentence-level extraction model with a simple, corpus-level component for aggregating the individual facts. We apply our model to learn extractors for NY Times text using weak supervision from Free-base. Experiments show that the approach runs quickly and yields surprising gains in accuracy, at both the aggregate and sentence level.
更多查看译文
关键词
automated learning,multi-instance learning,Knowledge-based weak supervision,NY Times text,information extraction,natural language text,noisy training data,novel approach,sentence-level extraction model,structured data,overlapping relation
AI 理解论文
溯源树
样例
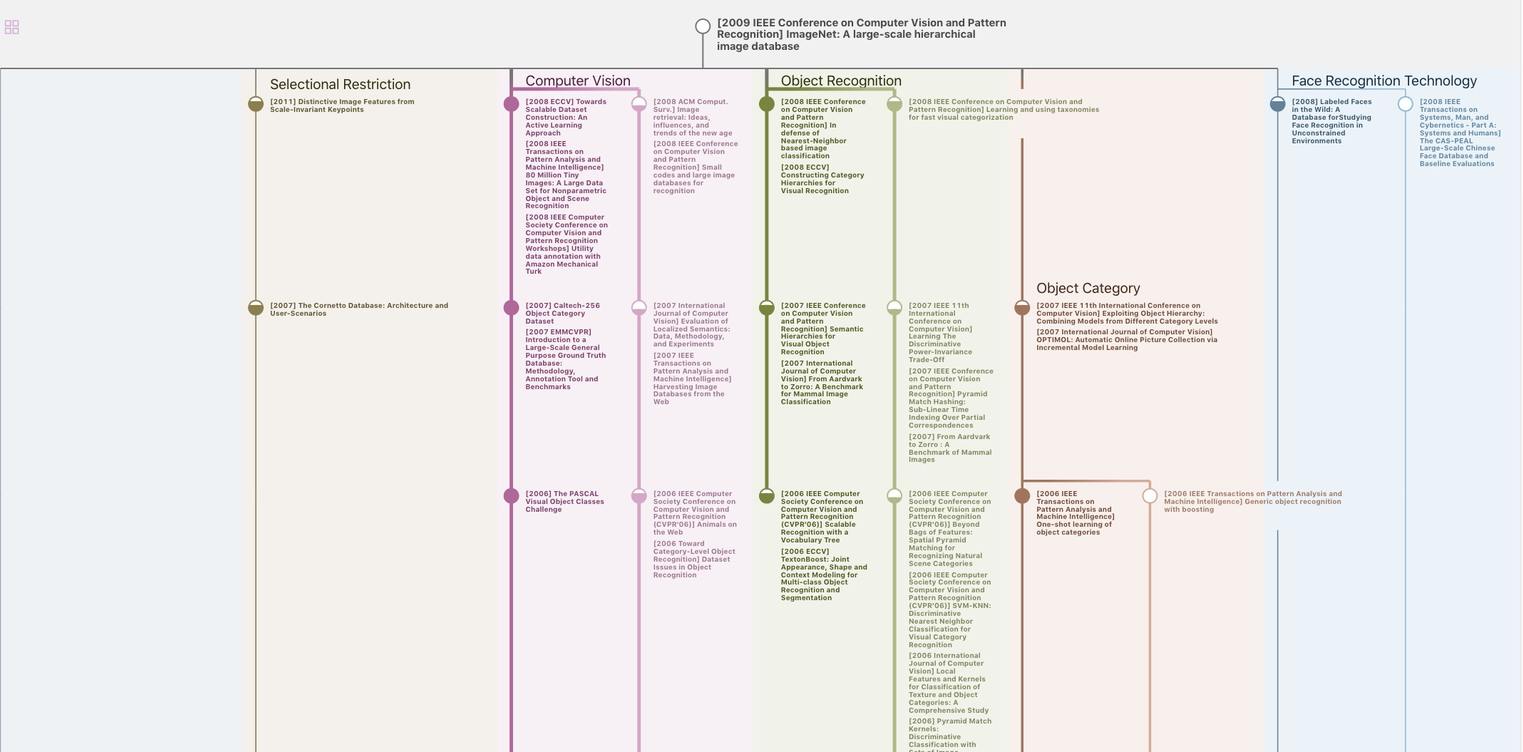
生成溯源树,研究论文发展脉络
Chat Paper
正在生成论文摘要