Vehicle Detection Using Partial Least Squares
IEEE Transactions on Pattern Analysis and Machine Intelligence(2011)
摘要
Detecting vehicles in aerial images has a wide range of applications, from urban planning to visual surveillance. We describe a vehicle detector that improves upon previous approaches by incorporating a very large and rich set of image descriptors. A new feature set called Color Probability Maps is used to capture the color statistics of vehicles and their surroundings, along with the Histograms of Oriented Gradients feature and a simple yet powerful image descriptor that captures the structural characteristics of objects named Pairs of Pixels. The combination of these features leads to an extremely high-dimensional feature set (approximately 70,000 elements). Partial Least Squares is first used to project the data onto a much lower dimensional sub-space. Then, a powerful feature selection analysis is employed to improve the performance while vastly reducing the number of features that must be calculated. We compare our system to previous approaches on two challenging data sets and show superior performance.
更多查看译文
关键词
gradient methods,image colour analysis,least squares approximations,road vehicles,video surveillance,aerial images,color probability maps,oriented gradients feature,partial least squares,powerful feature selection analysis,urban planning,vehicle detection,visual surveillance,Vehicle detection,feature selection.,partial least squares
AI 理解论文
溯源树
样例
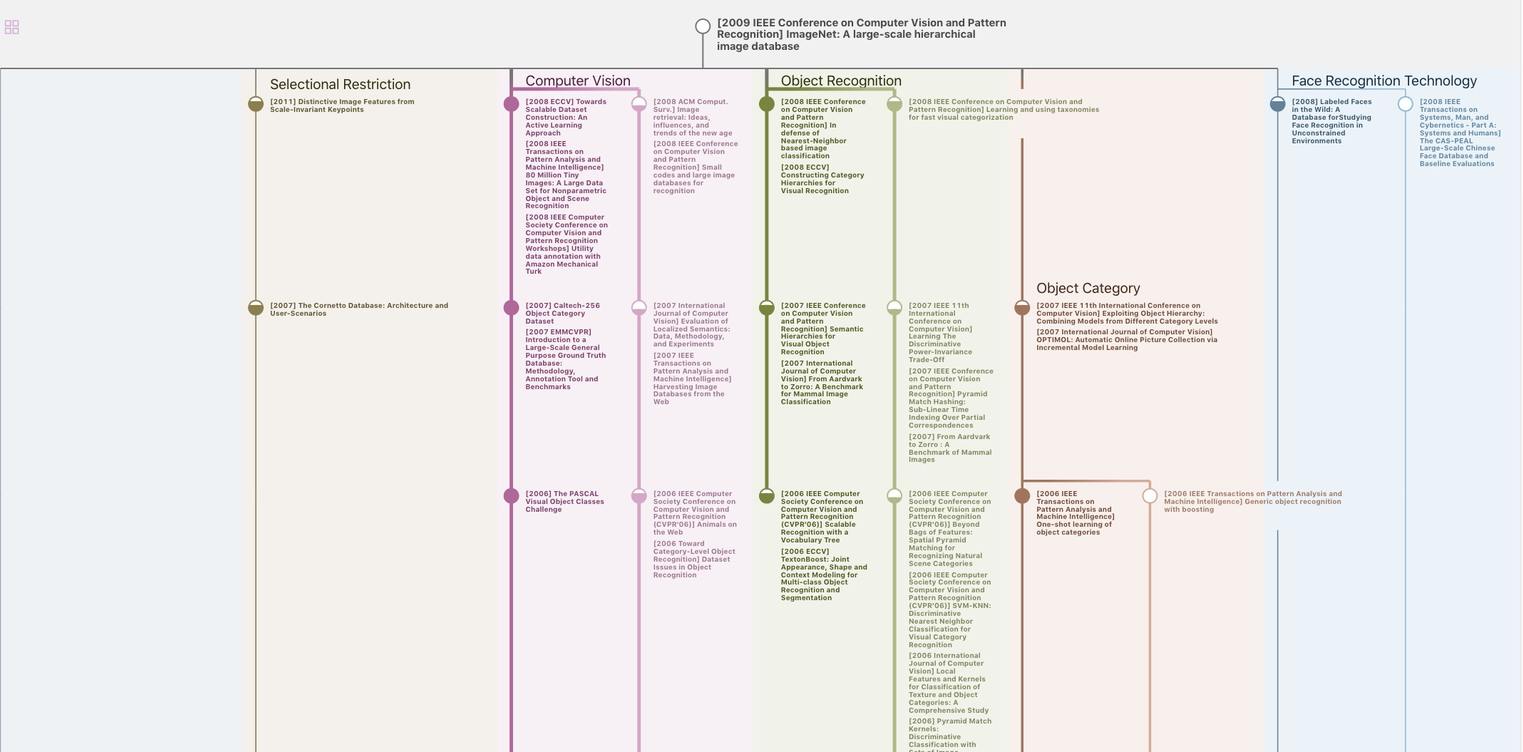
生成溯源树,研究论文发展脉络
Chat Paper
正在生成论文摘要