Online multiple instance boosting for object detection
Neurocomputing(2011)
摘要
Semi-supervised or unsupervised, incremental learning approaches based on online boosting are very popular for object detection. However, in the course of online learning, since the positive examples labelled by the current classifier may actually not be ''correct'', the optimal weak classifier is unlikely to be selected by previous approaches. This would directly lead to a decline in algorithm performance. In this paper, we present an improved online multiple instance learning algorithm based on boosting (called OMILBoost) for object detection. It can pick out the real correct image patch around labelled example with high possibility and thus, avoid drifting problem effectively. Furthermore, our method shows high performance when dealing with partial occlusions. Effectiveness is experimentally demonstrated on six representative video sequences.
更多查看译文
关键词
online boosting,multiple instance learning,high possibility,online learning,algorithm performance,object detection,improved online multiple instance,incremental learning,labelled example,high performance,current classifier,online multiple instance,optimal weak classifier
AI 理解论文
溯源树
样例
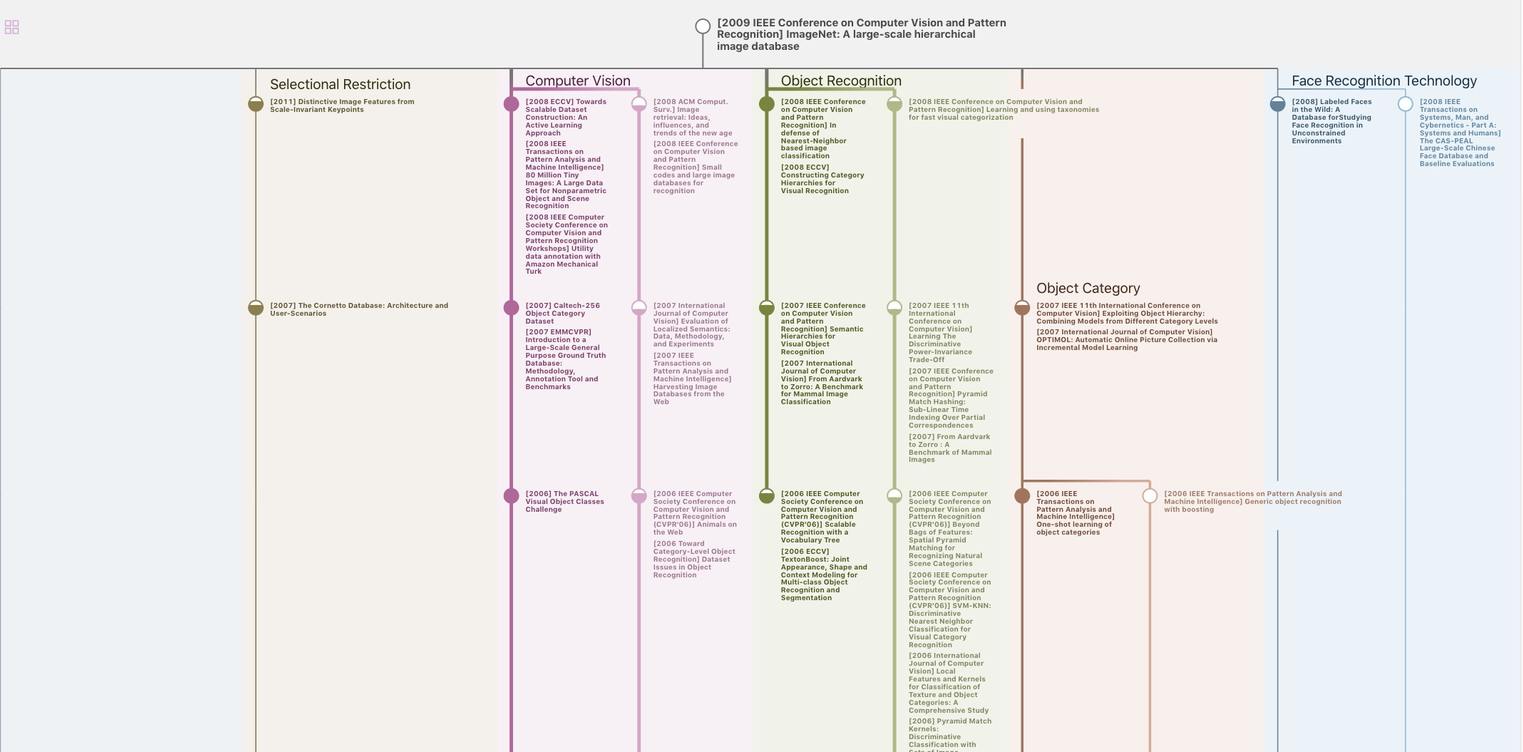
生成溯源树,研究论文发展脉络
Chat Paper
正在生成论文摘要