An Empirical Comparison of Platt Calibration and Inductive Confidence Machines for Predictions in Drug Discovery
Data Mining Workshops(2010)
摘要
During the early phase of drug discovery, machine learning methods are often utilized to select compounds to send for experimental screening. In order to accomplish this goal, any method that can provide estimates of error rate for a given set of predictions is an extremely valuable tool. In this paper we compare Platt Calibration Algorithm and recently introduced Conformal Algorithm to control the error rate in the sense of precision while preserving the ability to identify as many compounds as possible (recall) that are highly likely to be bio-active in a certain context. We empirically evaluate and compare the performance of Platt’s Calibration and offline Mondrian ICM in the context of SVM-based classification on 75 distinct classification problems. We perform this evaluation in the real world setting where the true class labels of compounds are unknown at the time of prediction and are only revealed after the biological experiment is completed. Our empirical results show that under this setting, offline Mondrian ICM and Platt Calibration are not able to bound precision rates very well on an absolute basis. Comparatively, Mondrian ICM, even though not theoretically designed to control precision directly, compares favorably with Platt Calibration for this task.
更多查看译文
关键词
calibration,chemical engineering computing,drugs,pattern classification,support vector machines,Piatt calibration,SVM-based classification,conformal algorithm,drug discovery,inductive confidence machines,machine learning,precision control,Drug Discovery,Mondrian ICM,Platt Calibration
AI 理解论文
溯源树
样例
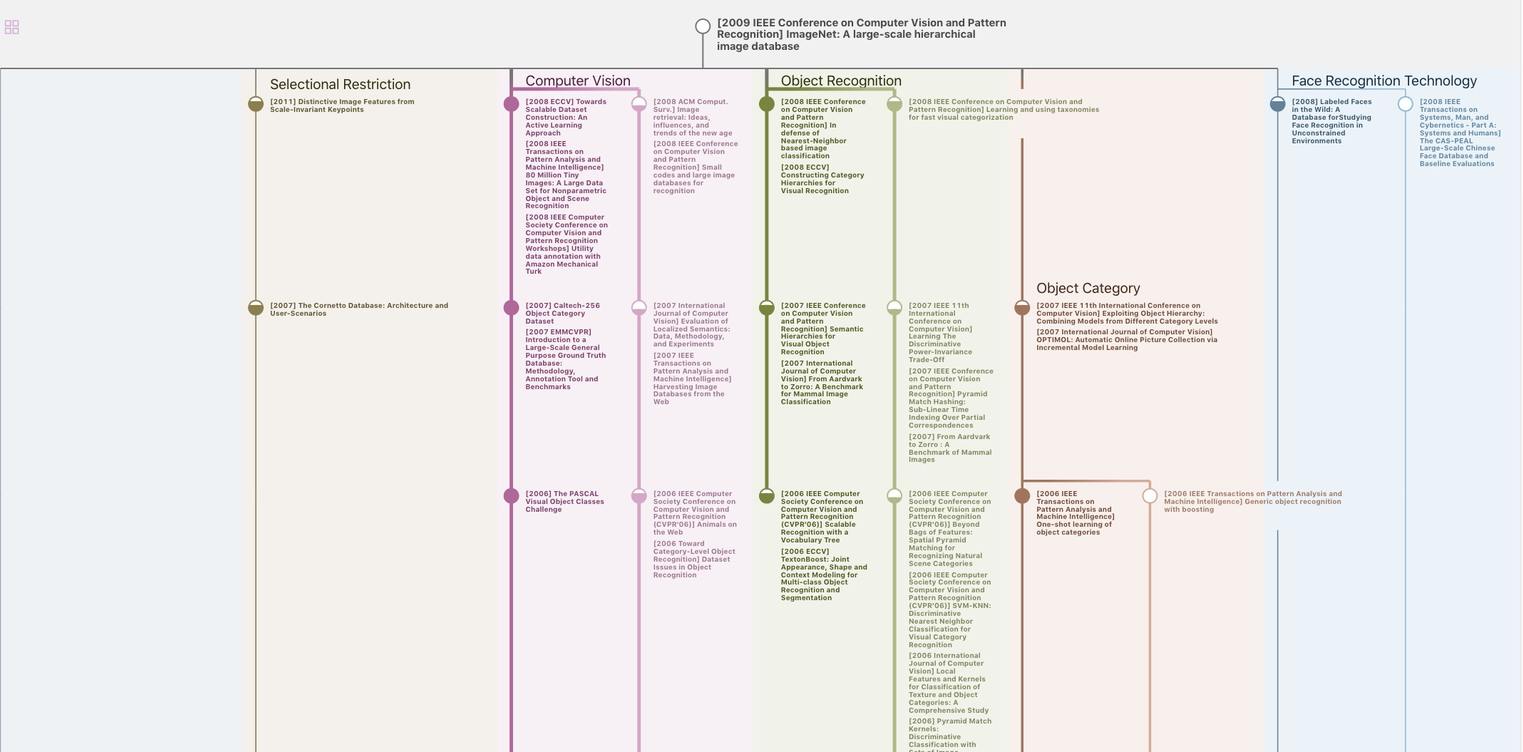
生成溯源树,研究论文发展脉络
Chat Paper
正在生成论文摘要