Vector Quantization Mappings for Speaker Verification
Pattern Recognition(2010)
摘要
In speaker verification several techniques have emerged to map variable length utterances into a fixed dimensional space for classification. One popular approach uses Maximum A-Posteriori (MAP) adaptation of a Gaussian Mixture Model (GMM) to create a super-vector. This paper investigates using Vector Quantisation (VQ) as the global model to provide a similar mapping. This less computationally complex mapping gives comparable results to its GMM counterpart while also providing the ability for an efficient iterative update enabling media files to be scanned with a fixed length window.
更多查看译文
关键词
Gaussian processes,computational complexity,speaker recognition,vector quantisation,GMM,Gaussian mixture model,MAP adaptation,computationally complex mapping,fixed dimensional space,fixed length window,maximum a-posteriori adaptation,speaker verification several techniques,variable length utterances,vector quantisation,vector quantization mappings
AI 理解论文
溯源树
样例
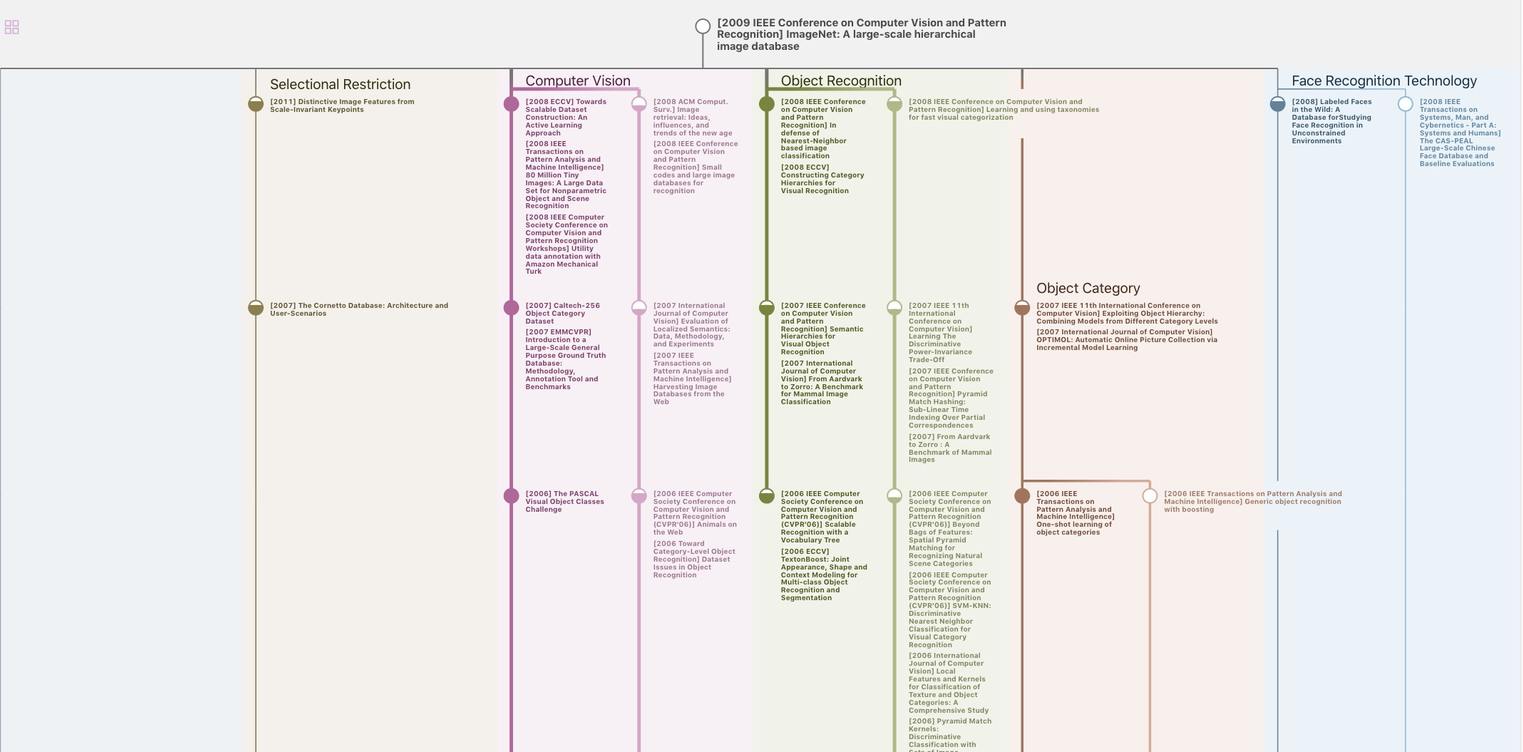
生成溯源树,研究论文发展脉络
Chat Paper
正在生成论文摘要