Improving Classification Accuracy by Comparing Local Features through Canonical Correlations
Pattern Recognition(2010)
摘要
Classifying images using features extracted from densely sampled local patches has enjoyed significant success in many detection and recognition tasks. It is also well known that generally more than one type of feature is needed to achieve robust classification performance. Previous works using multiple features have addressed this issue either through simple concatenation of feature vectors or through combining feature specific kernels at the classifier level. In this work we introduce a novel approach for combining features at the feature level by projecting two types of features onto two respective subspaces in which they are maximally correlated. We use their correlation as an augmented feature and demonstrate improvement in classification accuracy over simple combination through concatenation in a pedestrian detection framework.
更多查看译文
关键词
robust classification performance,classifier level,simple combination,improving classification accuracy,classification accuracy,local features,feature specific kernel,feature vector,feature level,multiple feature,canonical correlations,pedestrian detection framework,augmented feature,detectors,computer vision,histograms,correlation,canonical correlation,image classification,pixel,feature extraction
AI 理解论文
溯源树
样例
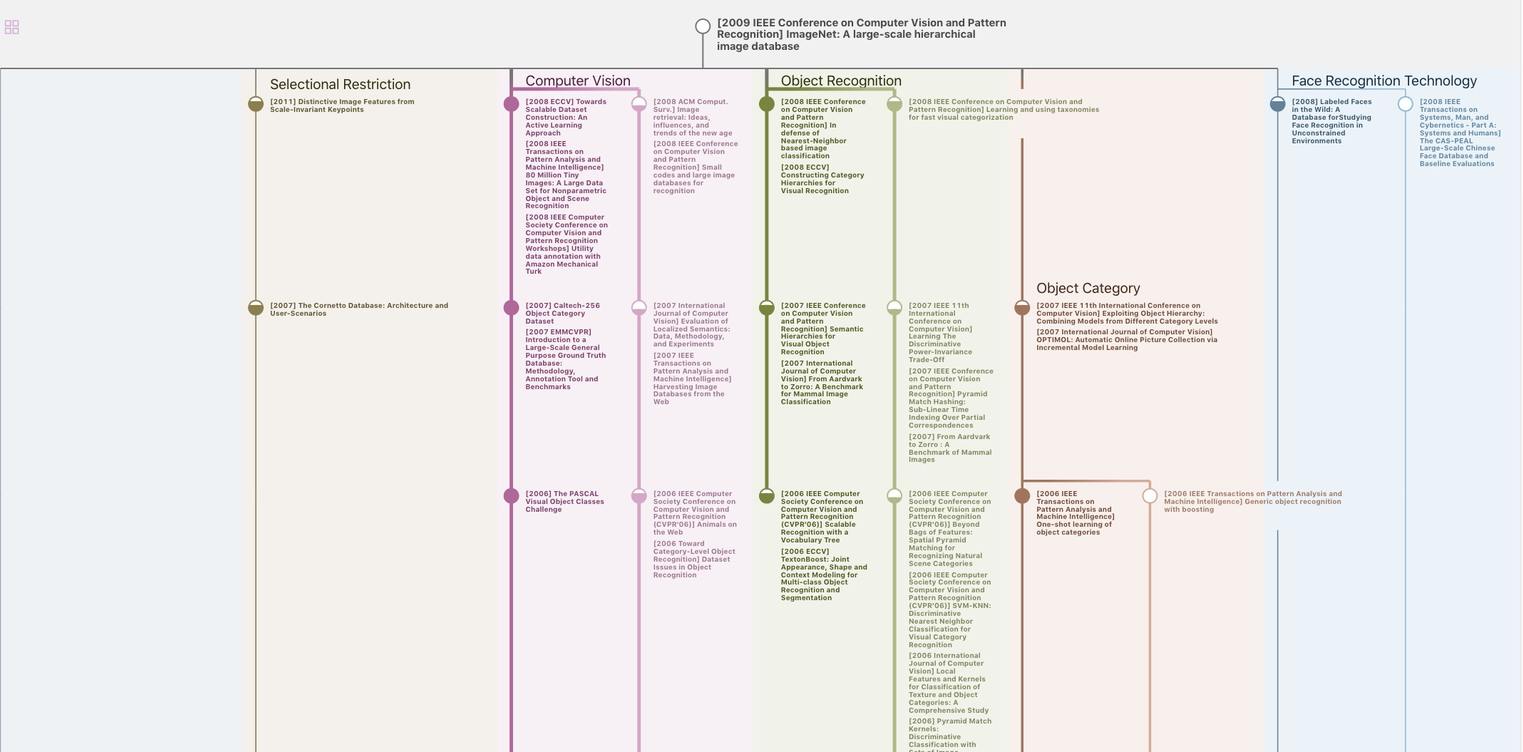
生成溯源树,研究论文发展脉络
Chat Paper
正在生成论文摘要