A kernel-based parametric method for conditional density estimation
Pattern Recognition(2011)
摘要
A conditional density function, which describes the relationship between response and explanatory variables, plays an important role in many analysis problems. In this paper, we propose a new kernel-based parametric method to estimate conditional density. An exponential function is employed to approximate the unknown density, and its parameters are computed from the given explanatory variable via a nonlinear mapping using kernel principal component analysis (KPCA). We develop a new kernel function, which is a variant to polynomial kernels, to be used in KPCA. The proposed method is compared with the Nadaraya-Watson estimator through numerical simulation and practical data. Experimental results show that the proposed method outperforms the Nadaraya-Watson estimator in terms of revised mean integrated squared error (RMISE). Therefore, the proposed method is an effective method for estimating the conditional densities.
更多查看译文
关键词
conditional density,new kernel function,explanatory variable,exponential function,kernel function,conditional density function,conditional density estimation,kernel principal component analysis,nadaraya–watson estimator,effective method,unknown density,new kernel-based parametric method,nadaraya-watson estimator,density estimation,numerical simulation,mean integrated squared error
AI 理解论文
溯源树
样例
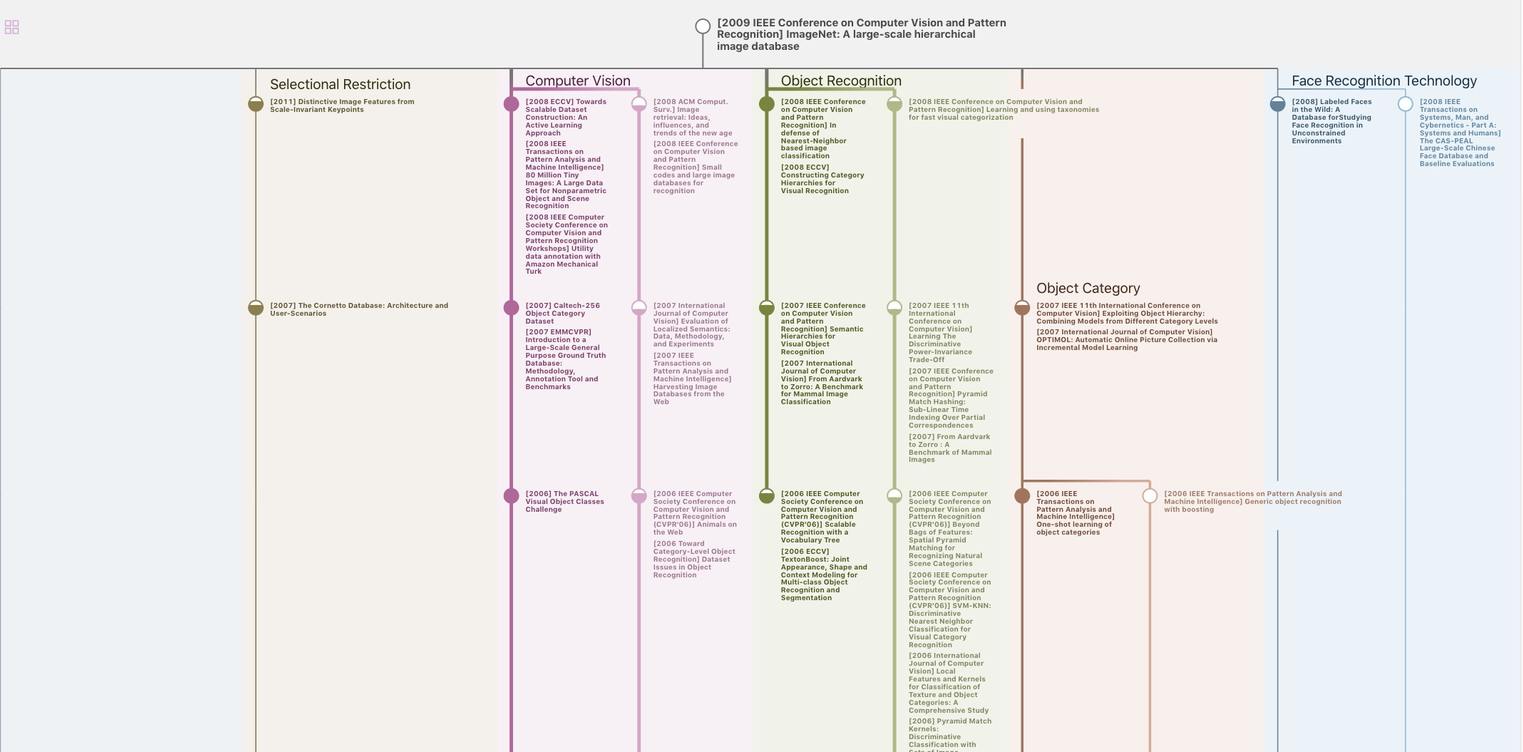
生成溯源树,研究论文发展脉络
Chat Paper
正在生成论文摘要