Combining evidence with a probabilistic framework for answer ranking and answer merging in question answering
Information Processing & Management(2010)
摘要
Question answering (QA) aims at finding exact answers to a user’s question from a large collection of documents. Most QA systems combine information retrieval with extraction techniques to identify a set of likely candidates and then utilize some ranking strategy to generate the final answers. This ranking process can be challenging, as it entails identifying the relevant answers amongst many irrelevant ones. This is more challenging in multi-strategy QA, in which multiple answering agents are used to extract answer candidates. As answer candidates come from different agents with different score distributions, how to merge answer candidates plays an important role in answer ranking. In this paper, we propose a unified probabilistic framework which combines multiple evidence to address challenges in answer ranking and answer merging. The hypotheses of the paper are that: (1) the framework effectively combines multiple evidence for identifying answer relevance and their correlation in answer ranking, (2) the framework supports answer merging on answer candidates returned by multiple extraction techniques, (3) the framework can support list questions as well as factoid questions, (4) the framework can be easily applied to a different QA system, and (5) the framework significantly improves performance of a QA system. An extensive set of experiments was done to support our hypotheses and demonstrate the effectiveness of the framework. All of the work substantially extends the preliminary research in Ko et al. (2007a). A probabilistic framework for answer selection in question answering. In: Proceedings of NAACL/HLT.
更多查看译文
关键词
Answer ranking,Answer merging,Question answering
AI 理解论文
溯源树
样例
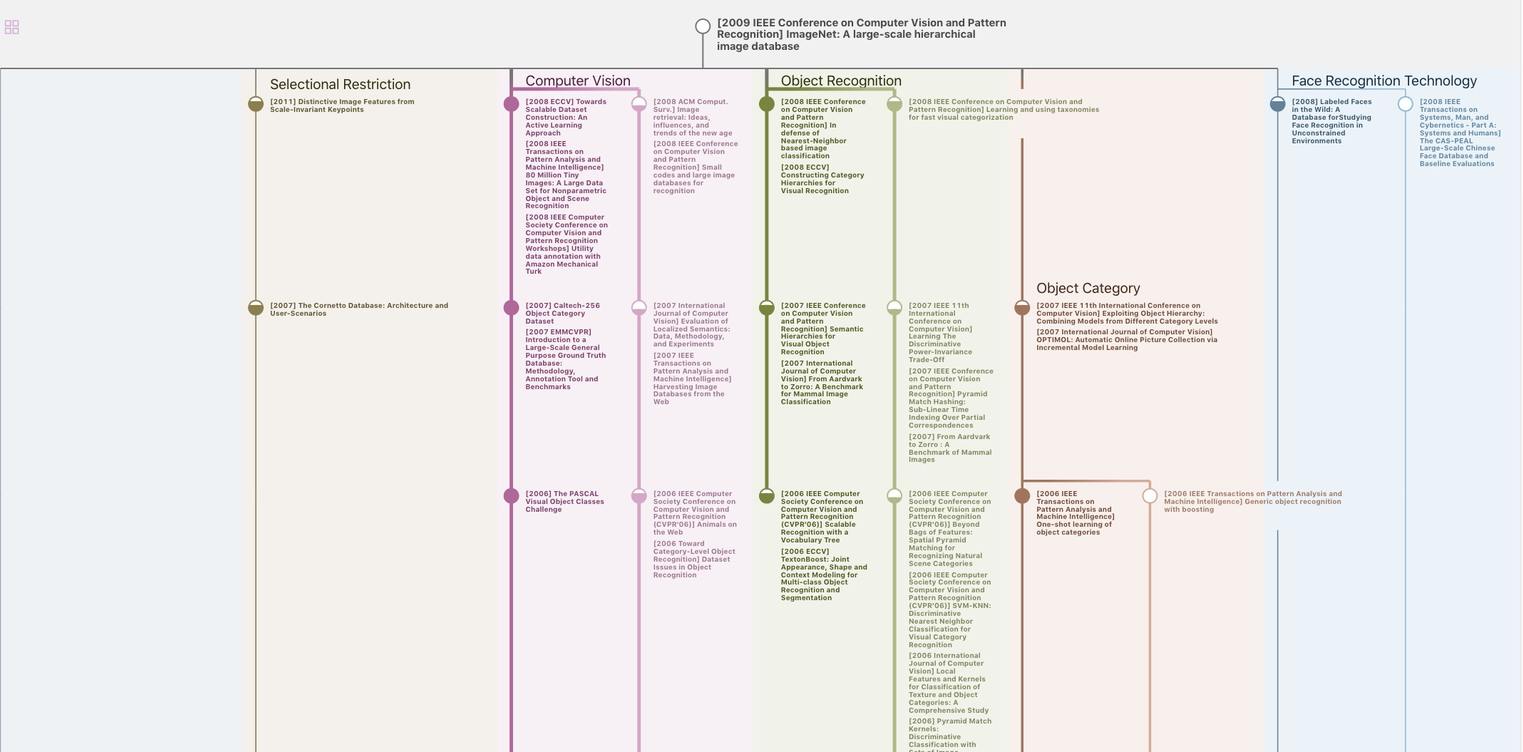
生成溯源树,研究论文发展脉络
Chat Paper
正在生成论文摘要