Alternating Projections for Learning with Expectation Constraints
UAI '09 Proceedings of the Twenty-Fifth Conference on Uncertainty in Artificial Intelligence(2012)
摘要
We present an objective function for learning with unlabeled data that utilizes auxiliary expectation constraints. We optimize this objective function using a procedure that alternates between information and moment projections. Our method provides an alternate interpretation of the posterior regularization framework (Graca et al., 2008), maintains uncertainty during optimization unlike constraint-driven learning (Chang et al., 2007), and is more efficient than generalized expectation criteria (Mann & McCallum, 2008). Applications of this framework include minimally supervised learning, semisupervised learning, and learning with constraints that are more expressive than the underlying model. In experiments, we demonstrate comparable accuracy to generalized expectation criteria for minimally supervised learning, and use expressive structural constraints to guide semi-supervised learning, providing a 3%-6% improvement over stateof-the-art constraint-driven learning.
更多查看译文
关键词
minimally supervised learning,generalized expectation criterion,objective function,constraint-driven learning,semi-supervised learning,state-of-the-art constraint-driven learning,auxiliary expectation constraint,expressive structural constraint,posterior regularization framework,alternate interpretation,Alternating projection
AI 理解论文
溯源树
样例
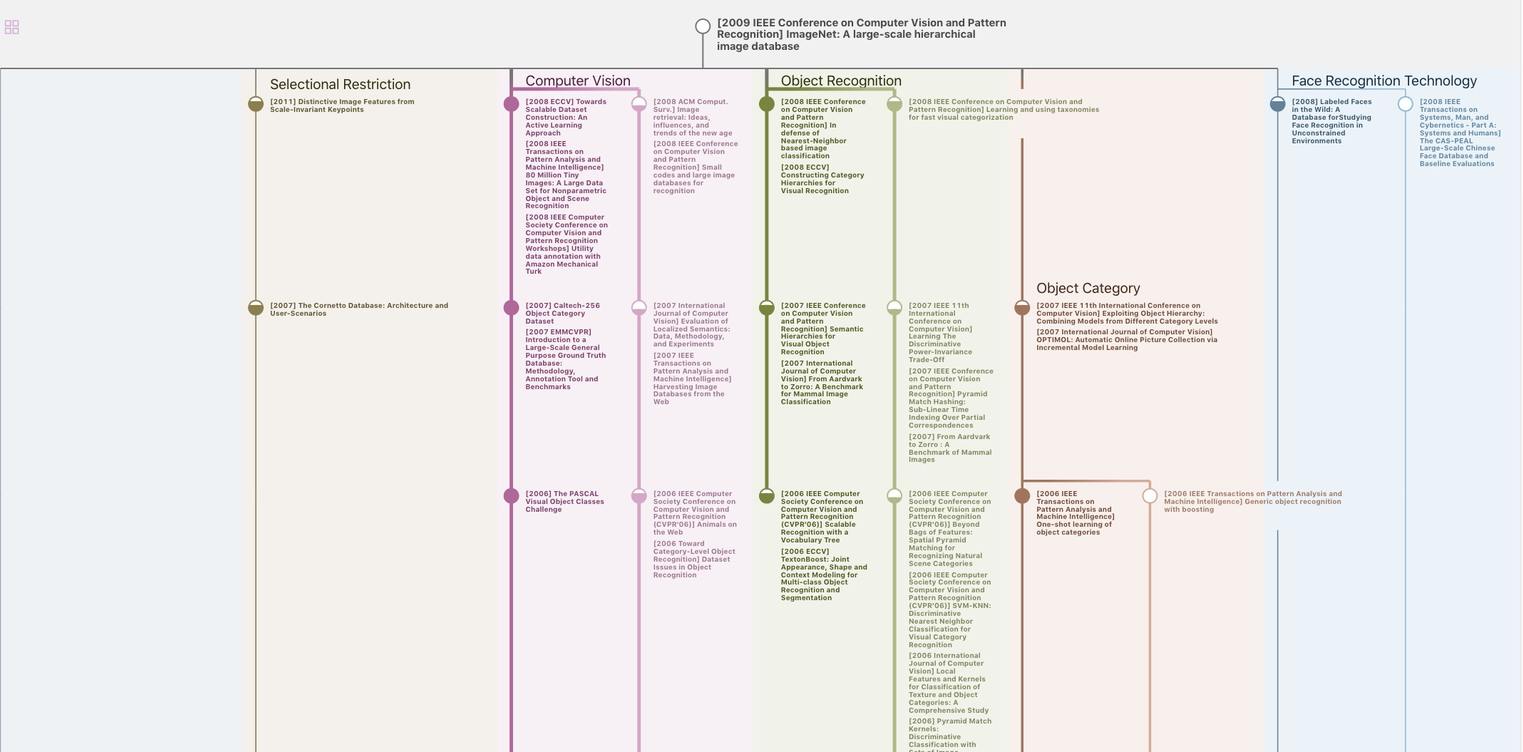
生成溯源树,研究论文发展脉络
Chat Paper
正在生成论文摘要