Log-Linear Convergence and Optimal Bounds for the (1 + 1)-ES
EA'07 Proceedings of the Evolution artificielle, 8th international conference on Artificial evolution(2007)
摘要
The (1 + 1)-ES is modeled by a general stochastic process whose asymptotic behavior is investigated. Under general assumptions, it is shown that the convergence of the related algorithm is sub-log-linear, bounded below by an explicit log-linear rate. For the specific case of spherical functions and scale-invariant algorithm, it is proved using the Law of Large Numbers for orthogonal variables, that the linear convergence holds almost surely and that the best convergence rate is reached. Experimental simulations illustrate the theoretical results.
更多查看译文
关键词
Random Vector,Sphere Function,Multivariate Normal Distribution,Optimal Bound,Positive Random Variable
AI 理解论文
溯源树
样例
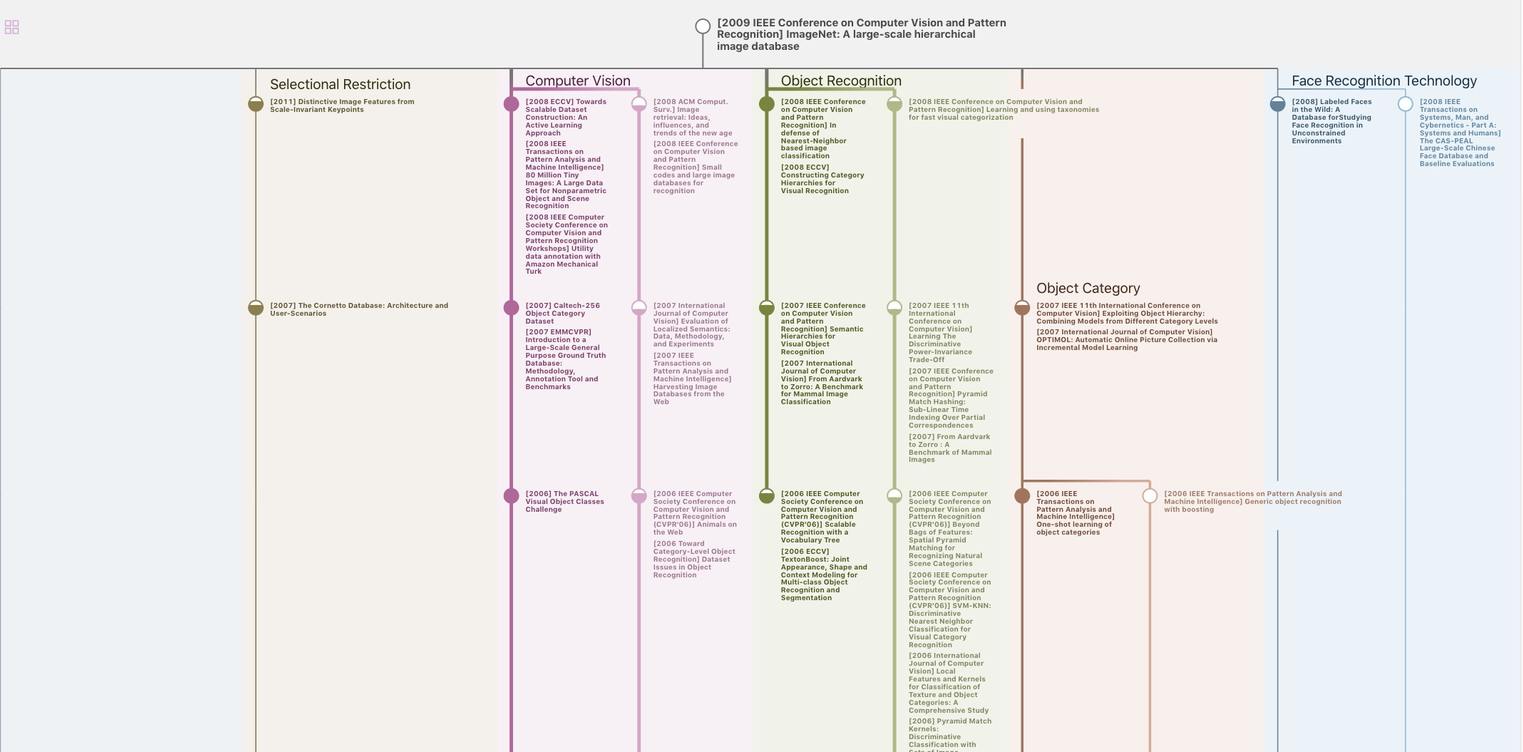
生成溯源树,研究论文发展脉络
Chat Paper
正在生成论文摘要