Robust Bayesian mixture modelling
Neurocomputing(2005)
摘要
Bayesian approaches to density estimation and clustering using mixture distributions allow the automatic determination of the number of components in the mixture. Previous treatments have focussed on mixtures having Gaussian components, but these are well known to be sensitive to outliers, which can lead to excessive sensitivity to small numbers of data points and consequent over-estimates of the number of components. In this paper we develop a Bayesian approach to mixture modelling based on Student-t distributions, which are heavier tailed than Gaussians and hence more robust. By expressing the Student-t distribution as a marginalization over additional latent variables we are able to derive a tractable variational inference algorithm for this model, which includes Gaussian mixtures as a special case. Results on a variety of real data sets demonstrate the improved robustness of our approach.
更多查看译文
关键词
gaussian mixture,student-t distribution,data point,latent variable model,outliers,small number,gaussian component,automatic determination,bayesian approach,mixture distribution,variational inference,robust bayesian mixture modelling,additional latent variable,model selection,density estimation,student t distribution,latent variable
AI 理解论文
溯源树
样例
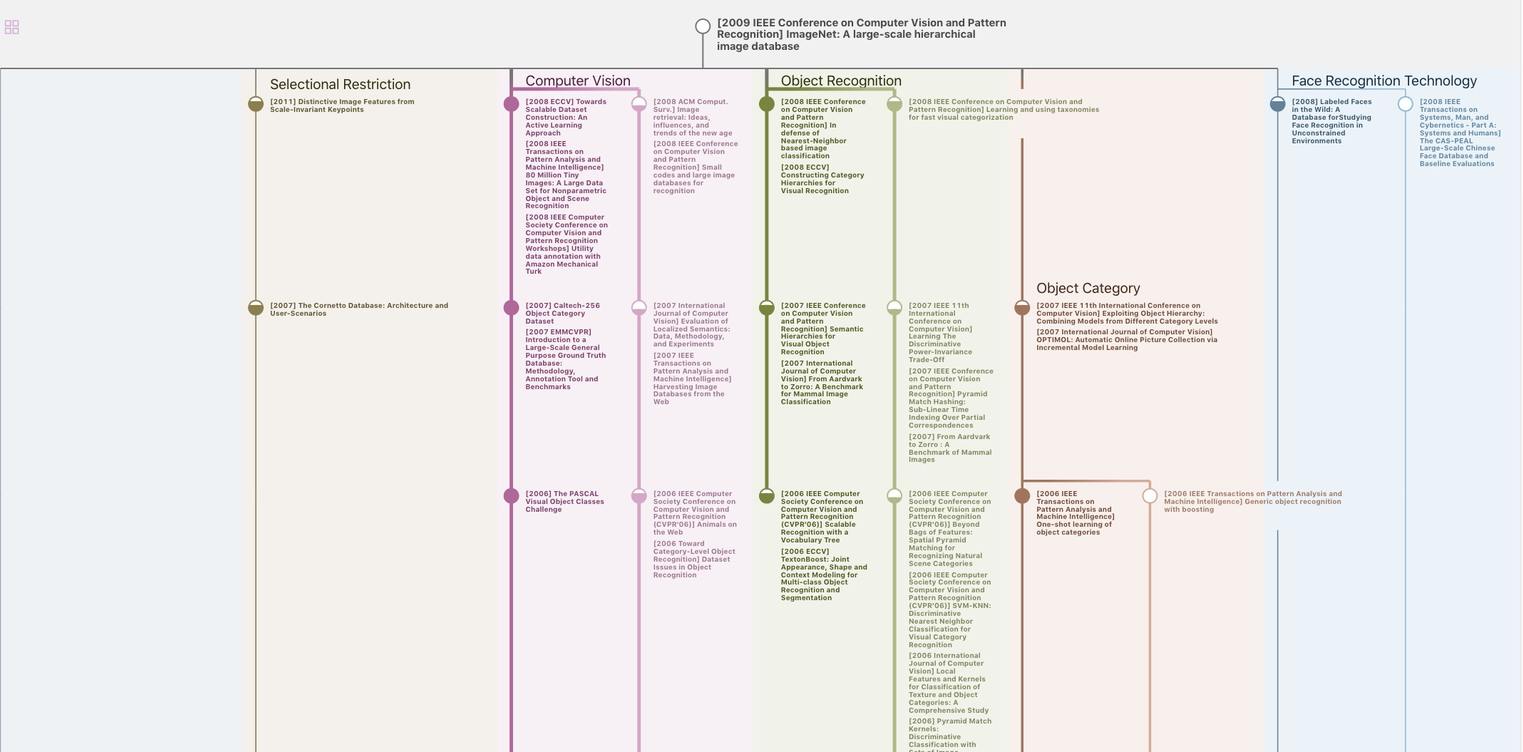
生成溯源树,研究论文发展脉络
Chat Paper
正在生成论文摘要