On The Asymptotic Equivalence Between Differential Hebbian And Temporal Difference Learning
NEURAL COMPUTATION(2009)
摘要
In this theoretical contribution, we provide mathematical proof that two of the most important classes of network learning-correlation-based differential Hebbian learning and reward-based temporal difference learning-are asymptotically equivalent when timing the learning with a modulatory signal. This opens the opportunity to consistently reformulate most of the abstract reinforcement learning framework from a correlation-based perspective more closely related to the biophysics of neurons.
更多查看译文
关键词
differential hebbian learning,mathematical proof,important class,reward-based temporal difference learning,theoretical contribution,correlation-based perspective,modulatory signal,asymptotic equivalence,asymptotically equivalent,abstract reinforcement,temporal difference learning,hebbian learning,reinforcement learning,temporal difference
AI 理解论文
溯源树
样例
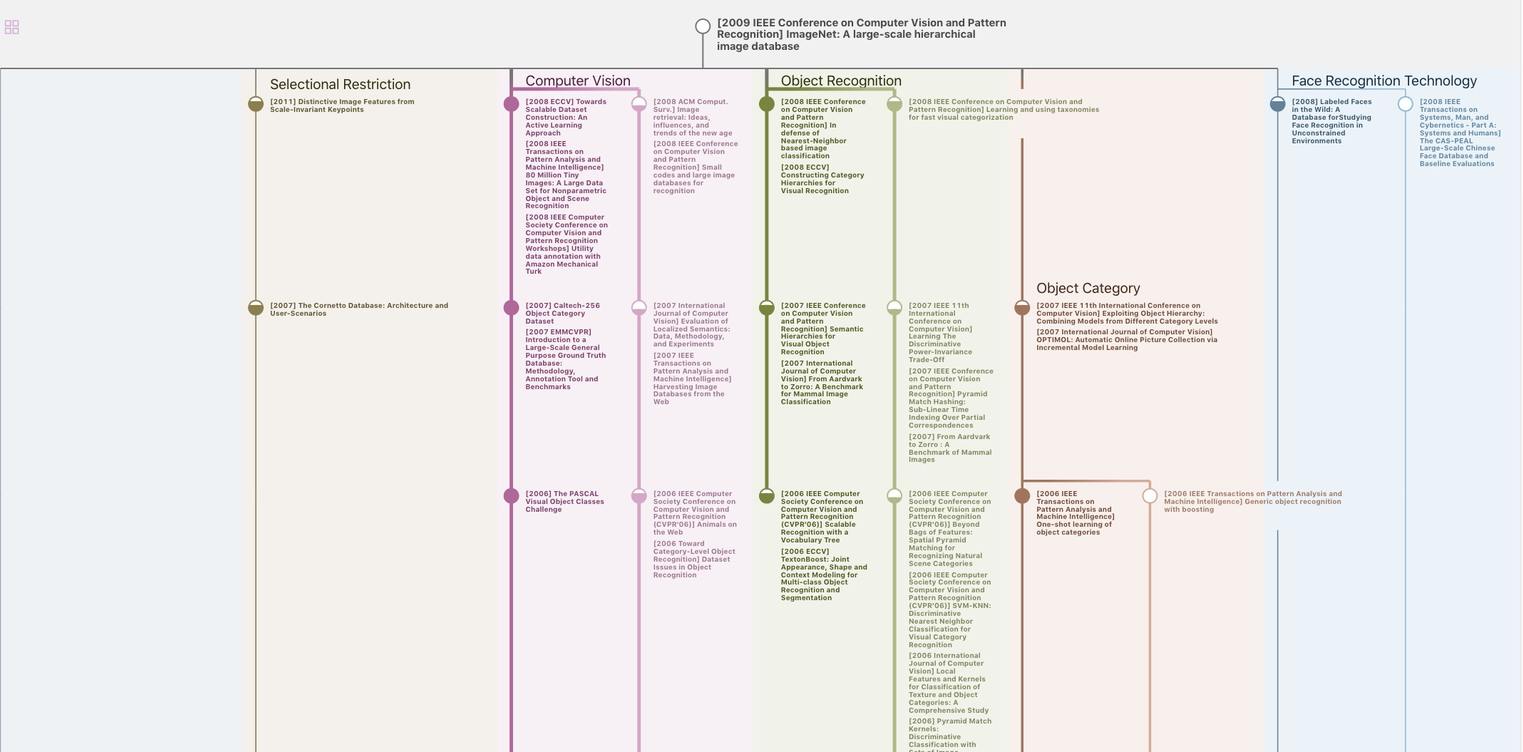
生成溯源树,研究论文发展脉络
Chat Paper
正在生成论文摘要