Independent Component Automatic Clustering And Its Application On Multi-Trails Imaginary Hand Movement Related Eeg
VECIMS'09 Proceedings of the 2009 IEEE international conference on Virtual Environments, Human-Computer Interfaces and Measurement Systems(2009)
摘要
How to extract task-relevant components from spontaneous electroencephalogram background is an open problem in EEG signal analysis. An Independent Component Automatic Clustering (ICAC) method, which combined Independent Component Analysis (ICA) with automatic clustering, is developed in this paper. In ICAC, the ICA decomposed components were grouped into several clusters and sorted automatically. A majority of task-relevant components could be grouped into one cluster and be recognized easily, which can compensate the traditional ICA limitation of component sorting without any task specialized orders. We adopted this method on multi trails EEG signals during imaginary hand movement, results showed that ICAC can automatically extract task-relevant component and increase the Fisher Criterion (FC) separability significantly. Furthermore, we show that the residual mutual information between task-relevant components is not useless as previously regarded but very useful on components recognition.
更多查看译文
关键词
Independent Component Automatic Clustering (ICAC),K-means clustering,imaginary hand movement,mutual information,Fisher Criterion
AI 理解论文
溯源树
样例
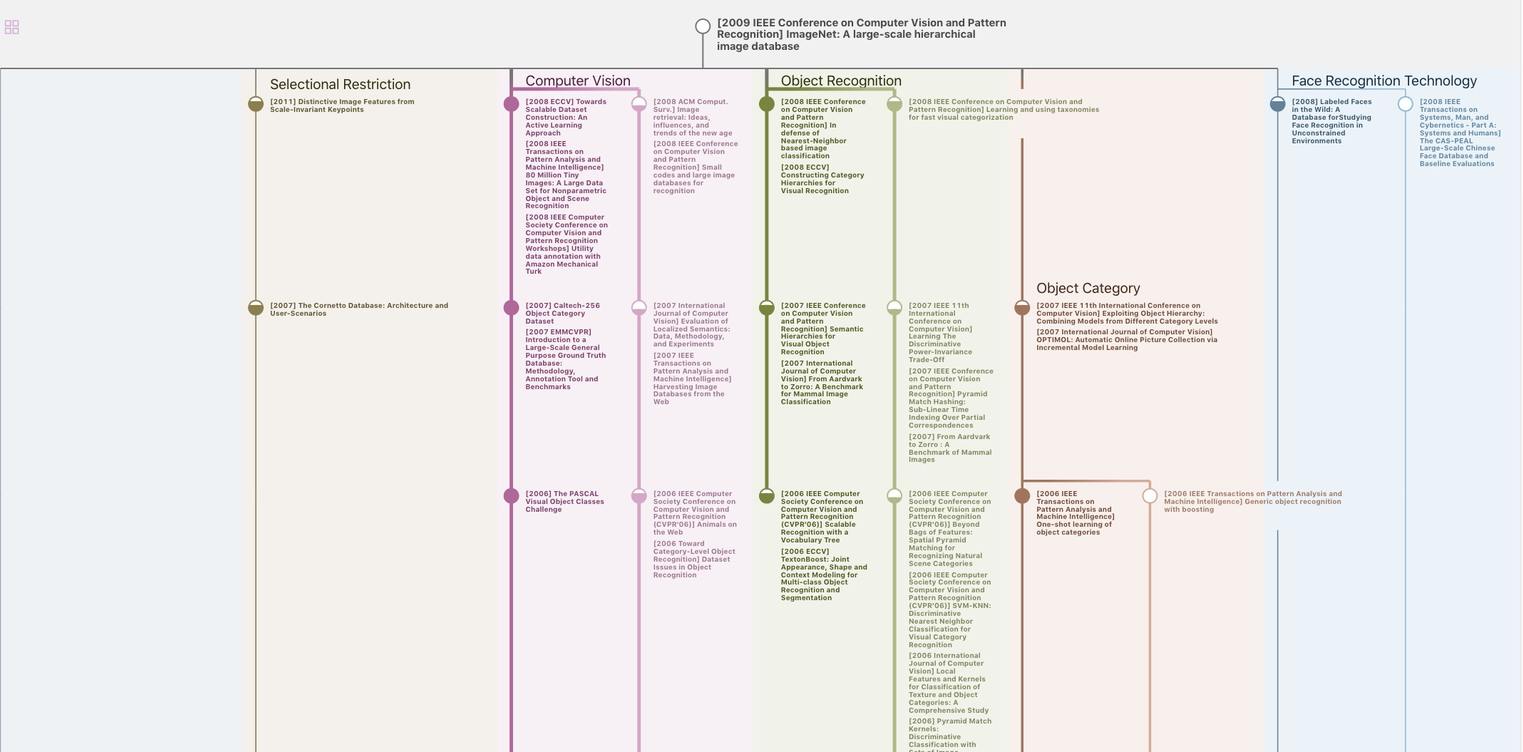
生成溯源树,研究论文发展脉络
Chat Paper
正在生成论文摘要