Model Adaptation via Model Interpolation and Boosting for Web Search Ranking
EMNLP '09 Proceedings of the 2009 Conference on Empirical Methods in Natural Language Processing: Volume 2 - Volume 2(2019)
摘要
This paper explores two classes of model adaptation methods for Web search ranking: Model Interpolation and error-driven learning approaches based on a boosting algorithm. The results show that model interpolation, though simple, achieves the best results on all the open test sets where the test data is very different from the training data. The tree-based boosting algorithm achieves the best performance on most of the closed test sets where the test data and the training data are similar, but its performance drops significantly on the open test sets due to the instability of trees. Several methods are explored to improve the robustness of the algorithm, with limited success.
更多查看译文
关键词
open test,test data,training data,Model Interpolation,closed test,best performance,best result,model adaptation method,Web search ranking,error-driven learning,model interpolation,web search ranking
AI 理解论文
溯源树
样例
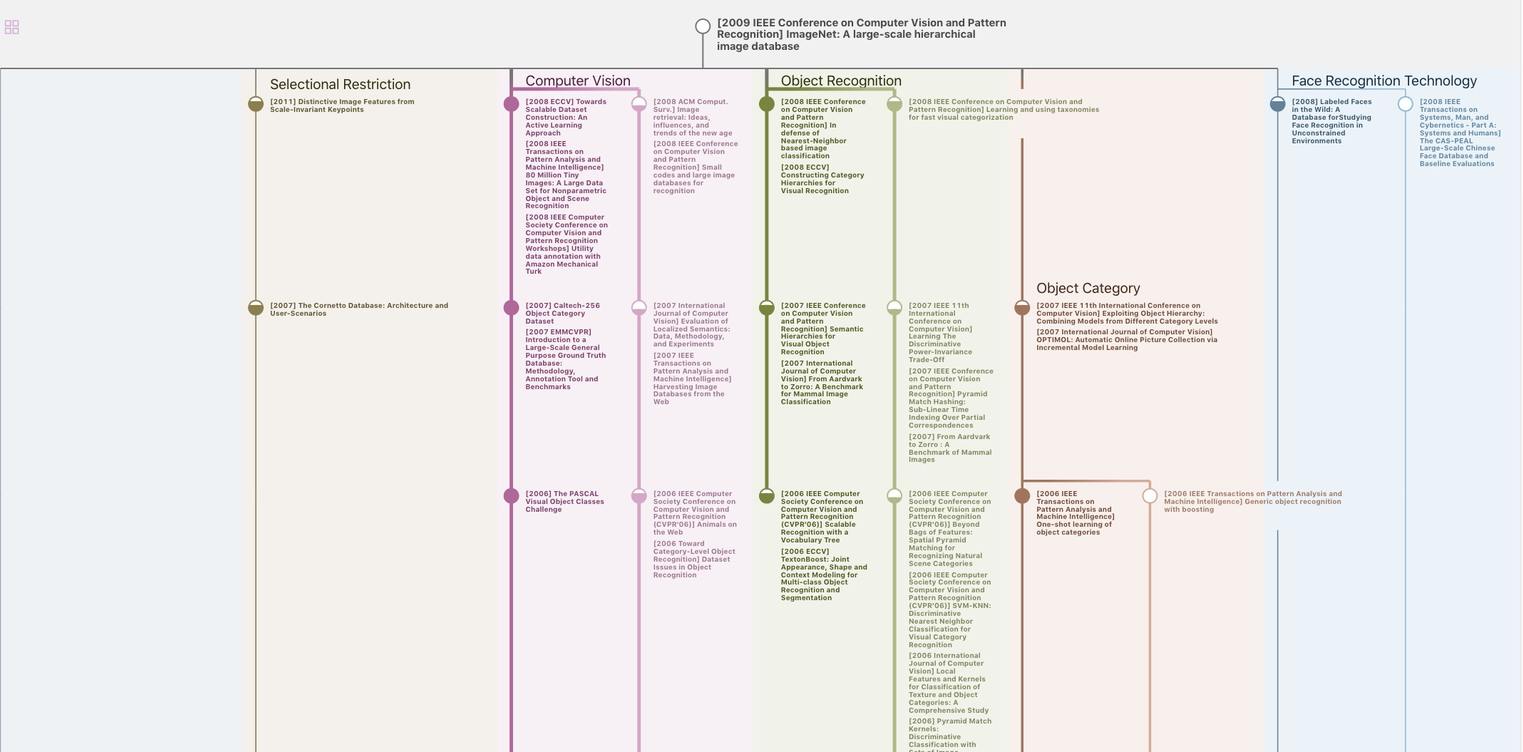
生成溯源树,研究论文发展脉络
Chat Paper
正在生成论文摘要