Interaction-Based Clustering of Multivariate Time Series
Miami, FL(2009)
摘要
In this paper, we present a novel approach to clustering multivariate time series. In contrast to previous approaches, we base our cluster notion on the interactions between the univariate time series within a data object. Our objective is to assign objects with a similar intrinsic interaction pattern to a common cluster. To formalize this idea, we define a cluster by a set of mathematical models describing the cluster-specific interaction pattern. In addition, we propose interaction K-means (IKM), an efficient algorithm for partitioning clustering of multivariate time series. The cluster-specific interaction patterns detected by IKM provide valuable information for interpretation of the cluster content. An extensive experimental evaluation on synthetic and real world data demonstrates the effectiveness and efficiency of our approach.
更多查看译文
关键词
similar intrinsic interaction pattern,multivariate time series,novel approach,cluster-specific interaction pattern,partitioning clustering,interaction k-means,univariate time series,common cluster,cluster notion,interaction-based clustering,cluster content,time series,k means,integrated circuits,data mining,computational modeling,noise,time series analysis,mathematical models,algorithms,mathematical model,clustering algorithms
AI 理解论文
溯源树
样例
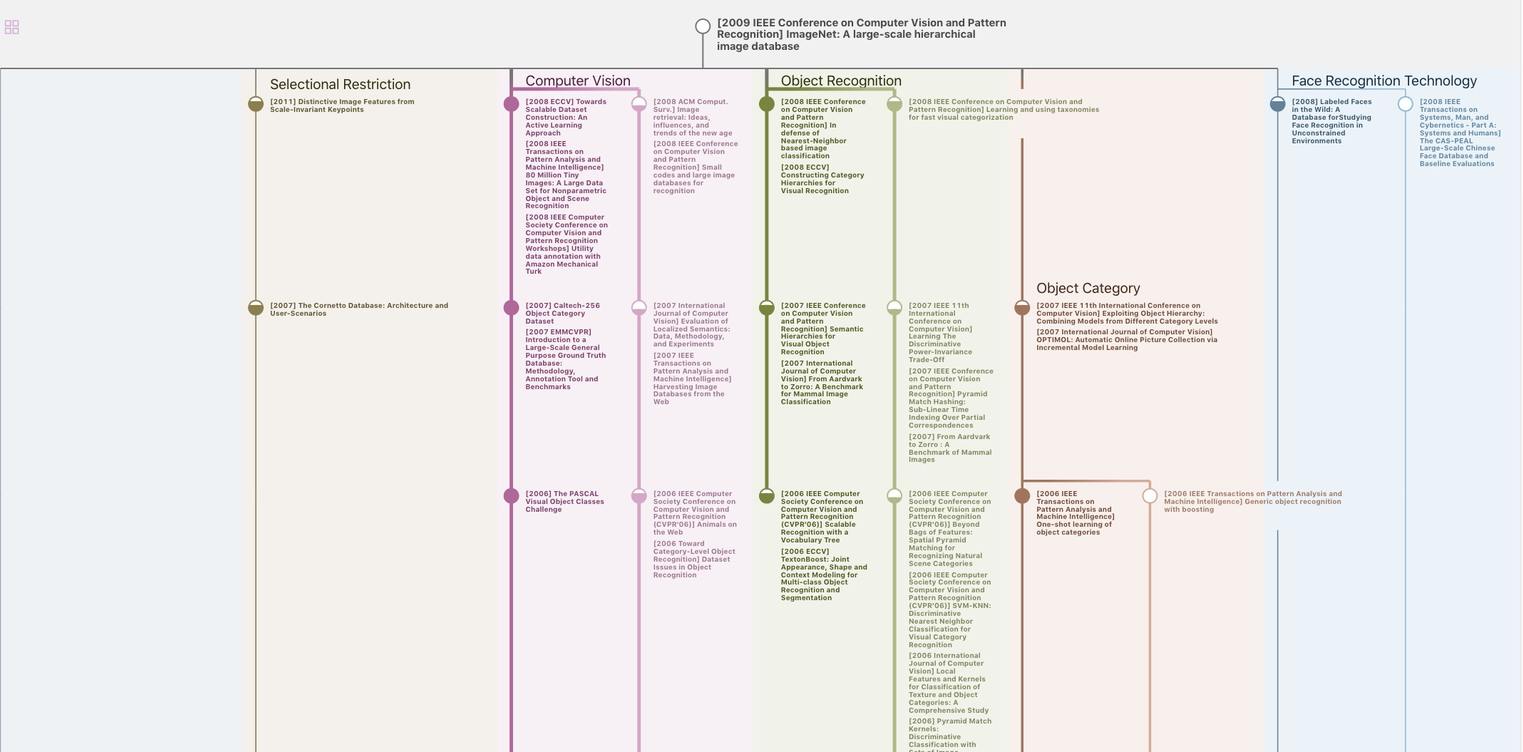
生成溯源树,研究论文发展脉络
Chat Paper
正在生成论文摘要