Finding associations and computing similarity via biased pair sampling
ICDM '09 Proceedings of the 2009 Ninth IEEE International Conference on Data Mining(2012)
摘要
Sampling-based methods have previously been proposed for the problem of finding interesting associations in data, even for low-support items. While these methods do not guarantee precise results, they can be vastly more efficient than approaches that rely on exact counting. However, for many similarity measures no such methods have been known. In this paper, we show how a wide variety of measures can be supported by a simple biased sampling method. The method also extends to find high-confidence association rules. We demonstrate theoretically that our method is superior to exact methods when the threshold for “interesting similarity/confidence” is above the average pairwise similarity/confidence, and the average support is not too low. Our method is particularly advantageous when transactions contain many items. We confirm in experiments on standard association mining benchmarks that we obtain a significant speedup on real data sets. Reductions in computation time of over an order of magnitude, and significant savings in space, are observed.
更多查看译文
关键词
Algorithms,Sampling,Data mining,Association rules
AI 理解论文
溯源树
样例
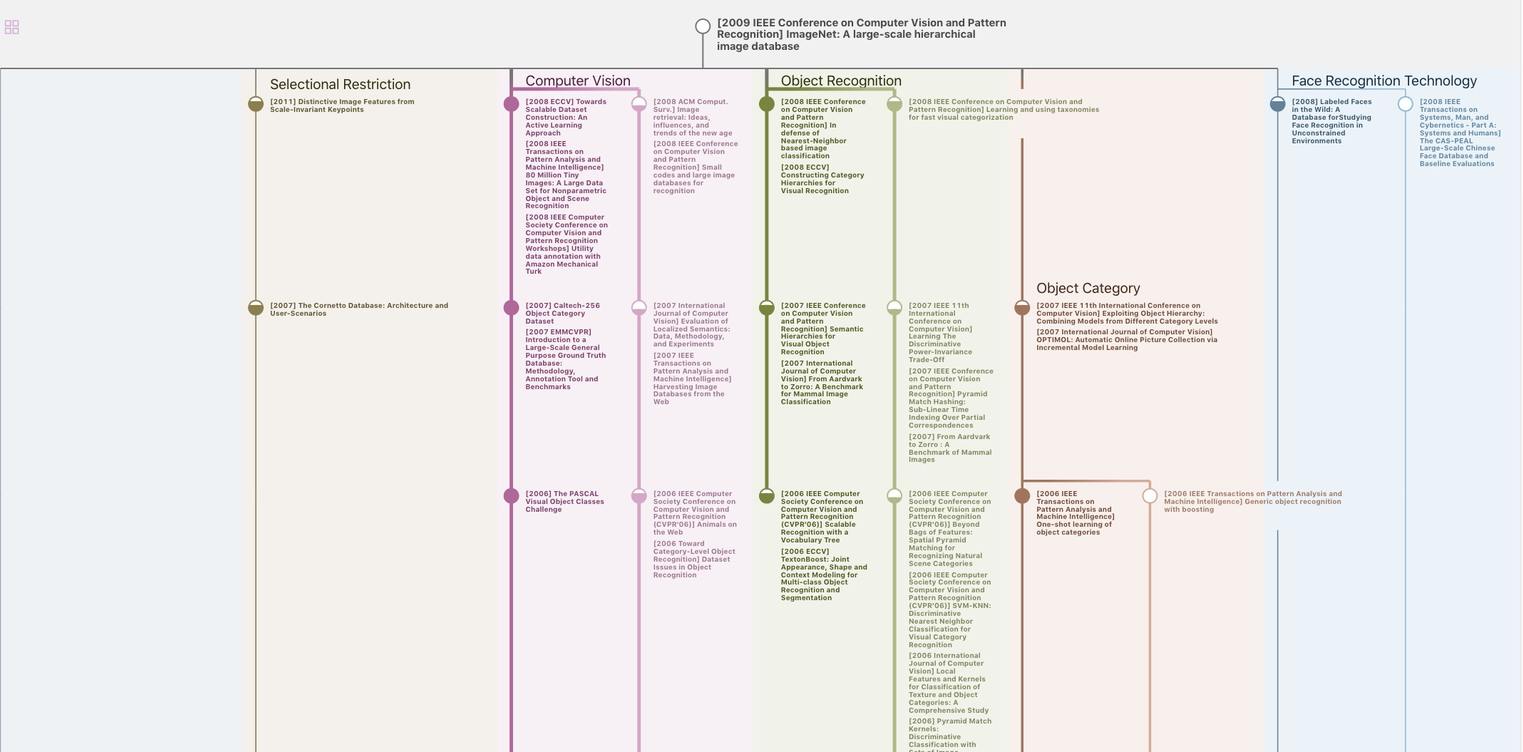
生成溯源树,研究论文发展脉络
Chat Paper
正在生成论文摘要