Network anomaly detection and classification via opportunistic sampling
IEEE Network(2009)
摘要
In this article the emphasis is placed on the evaluation of the impact of intelligent flow sampling techniques on the detection and classification of network anomalies. Based on the observation that for specific-purpose applications such as anomaly detection a large fraction of information is contained in a small fraction of flows, we demonstrate that by using sampling techniques that opportunistically and preferentially sample traffic data, we achieve "magnification" of the appearance of anomalies within the sampled data set and therefore improve their detection. Therefore, the inherently "lossy" sampling process is transformed to an advantageous feature in the anomaly detection case, allowing the revealing of anomalies that would be otherwise untraceable, and thus becoming the vehicle for efficient anomaly detection and classification. The evaluation of the impact of intelligent sampling techniques on the anomaly detection process is based on the application of an entropy-based anomaly detection method on a packet trace with data that has been collected from a real operational university campus network.
更多查看译文
关键词
computer networks,entropy,pattern classification,sampling methods,telecommunication security,telecommunication traffic,entropy-based anomaly detection method,inherently lossy sampling process,intelligent flow sampling technique,network anomaly classification,network anomaly detection,opportunistic sampling technique,sample traffic data
AI 理解论文
溯源树
样例
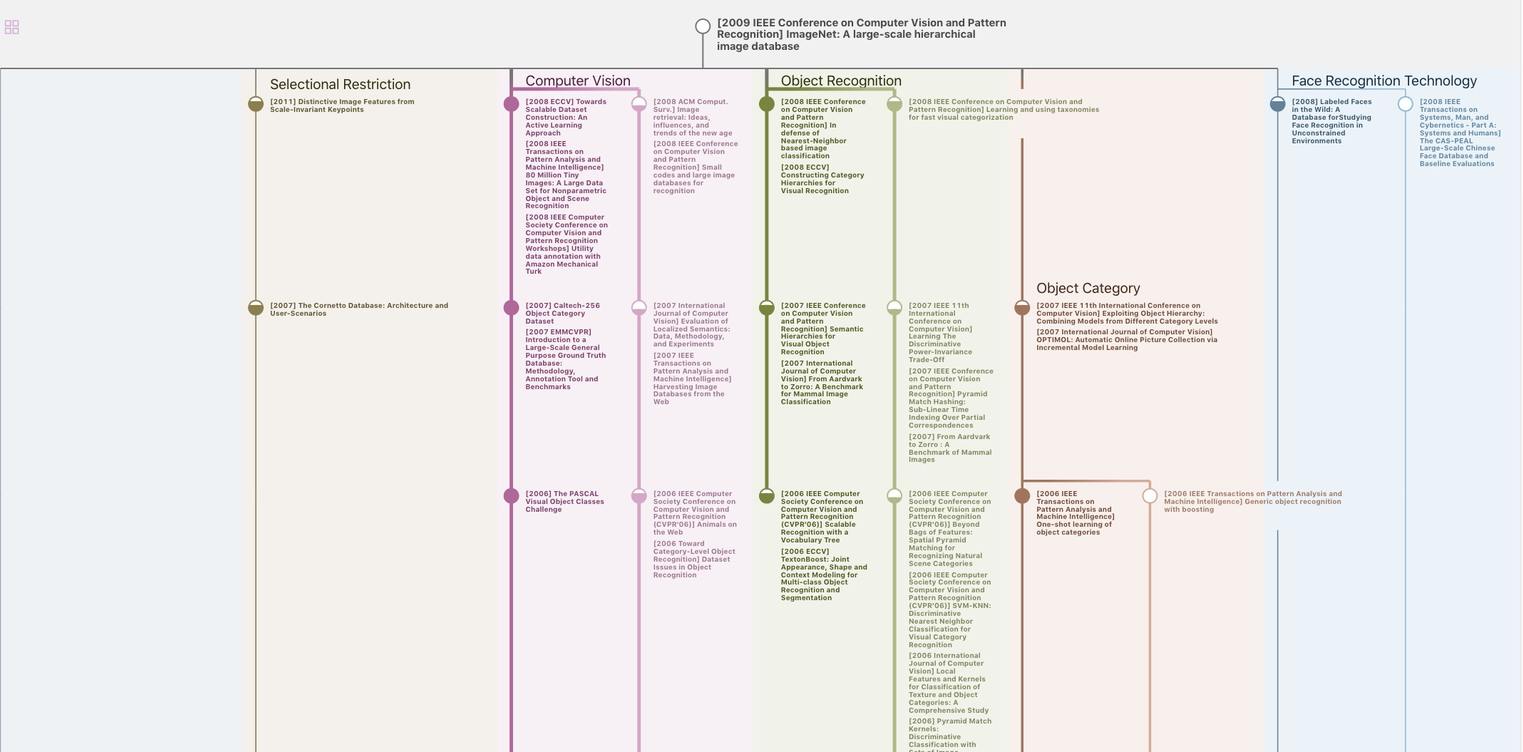
生成溯源树,研究论文发展脉络
Chat Paper
正在生成论文摘要