Periodic step-size adaptation in second-order gradient descent for single-pass on-line structured learning
Machine Learning(2009)
摘要
It has been established that the second-order stochastic gradient descent (SGD) method can potentially achieve generalization performance as well as empirical optimum in a single pass through the training examples. However, second-order SGD requires computing the inverse of the Hessian matrix of the loss function, which is prohibitively expensive for structured prediction problems that usually involve a very high dimensional feature space. This paper presents a new second-order SGD method, called Periodic Step-size Adaptation (PSA). PSA approximates the Jacobian matrix of the mapping function and explores a linear relation between the Jacobian and Hessian to approximate the Hessian, which is proved to be simpler and more effective than directly approximating Hessian in an on-line setting. We tested PSA on a wide variety of models and tasks, including large scale sequence labeling tasks using conditional random fields and large scale classification tasks using linear support vector machines and convolutional neural networks. Experimental results show that single-pass performance of PSA is always very close to empirical optimum.
更多查看译文
关键词
Conditional random fields,Convolutional neural networks,On-line learning,Sequence labeling,Stochastic gradient descent
AI 理解论文
溯源树
样例
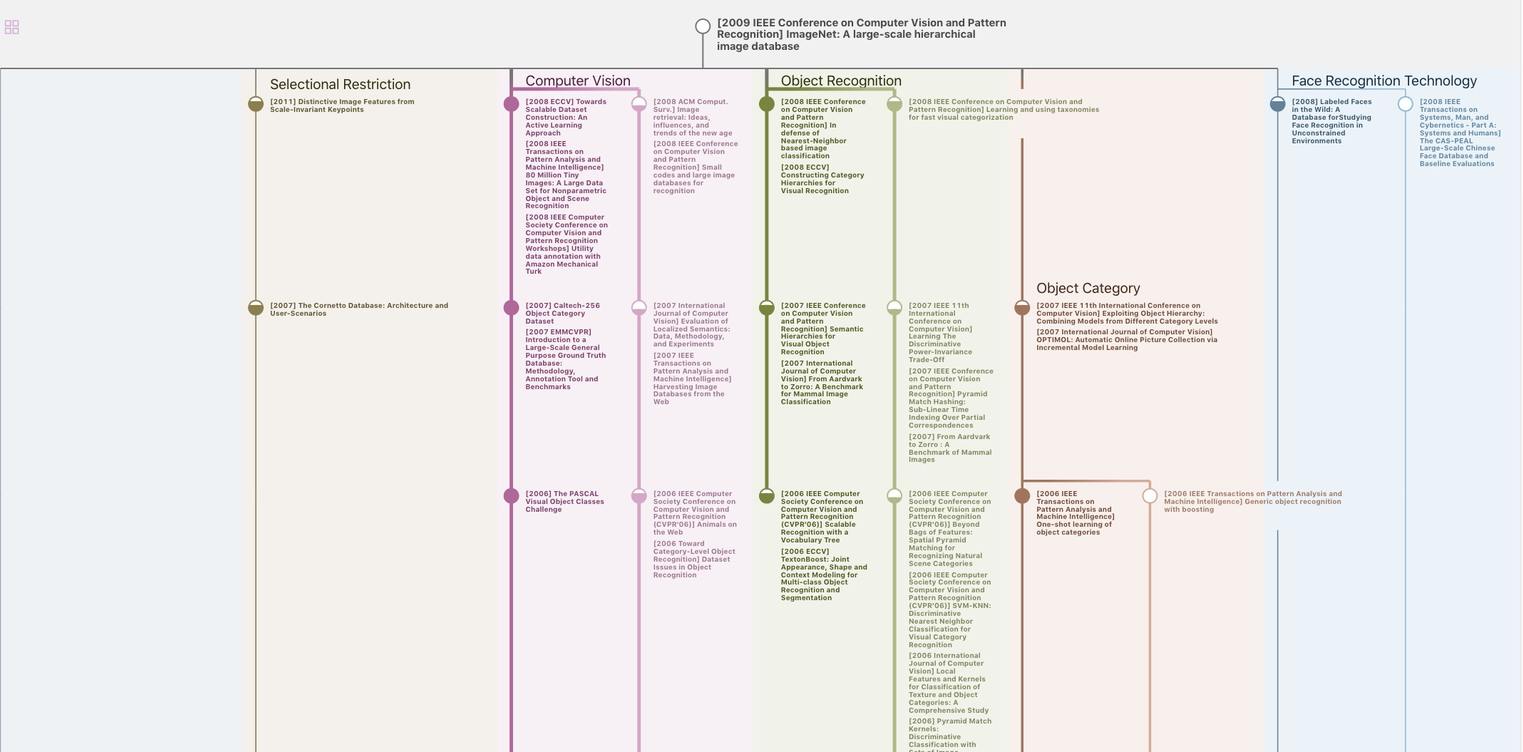
生成溯源树,研究论文发展脉络
Chat Paper
正在生成论文摘要