An On-the-Fly Parameter Dimension Reduction Approach to Fast Second-Order Statistical Static Timing Analysis
IEEE Transactions on Computer-Aided Design of Integrated Circuits and Systems(2009)
摘要
While first-order statistical static timing analysis (SSTA) techniques enjoy good runtime efficiency desired for tackling large industrial designs, more accurate second-order SSTA techniques have been proposed to improve the analysis accuracy, but at the cost of high computational complexity. Although many sources of variations may impact the circuit performance, considering a large number of inter- and intra-die variations in the traditional SSTA is very challenging. In this paper, we address the analysis complexity brought by high parameter dimensionality in SSTA and propose an accurate yet fast second-order SSTA algorithm based on novel on-the-fly parameter dimension reduction techniques. By developing a reduced rank regression (RRR)-based approach and a method of moments (MOM)-based parameter reduction algorithm within the block-based SSTA flow, we demonstrate that accurate second-order SSTA can be extended to a much higher parameter dimensionality than what is possible before. Our experimental results have shown that the proposed parameter reductions can achieve up to 10times parameter dimension reduction and lead to significantly improved second-order SSTA under a large set of process variations.
更多查看译文
关键词
Reduced rank regression,statistical parameter dimension reduction,statistical static timing analysis,second-order statistical static timing analysis,second-order ssta,regression analysis,second-order statistical static timing,on-the-fly parameter dimension reduction,timing,runtime efficiency,computational complexity,novel on-the-fly parameter dimension,parameter dimensionality,parameter reduction algorithm,second-order ssta algorithm,accurate second-order ssta technique,network analysis,circuit performance,reduced rank regression,method of moments,on-the-fly parameter dimension reduction approach,block-based ssta flow,traditional ssta,higher parameter dimensionality,high parameter dimensionality,parameter dimension reduction
AI 理解论文
溯源树
样例
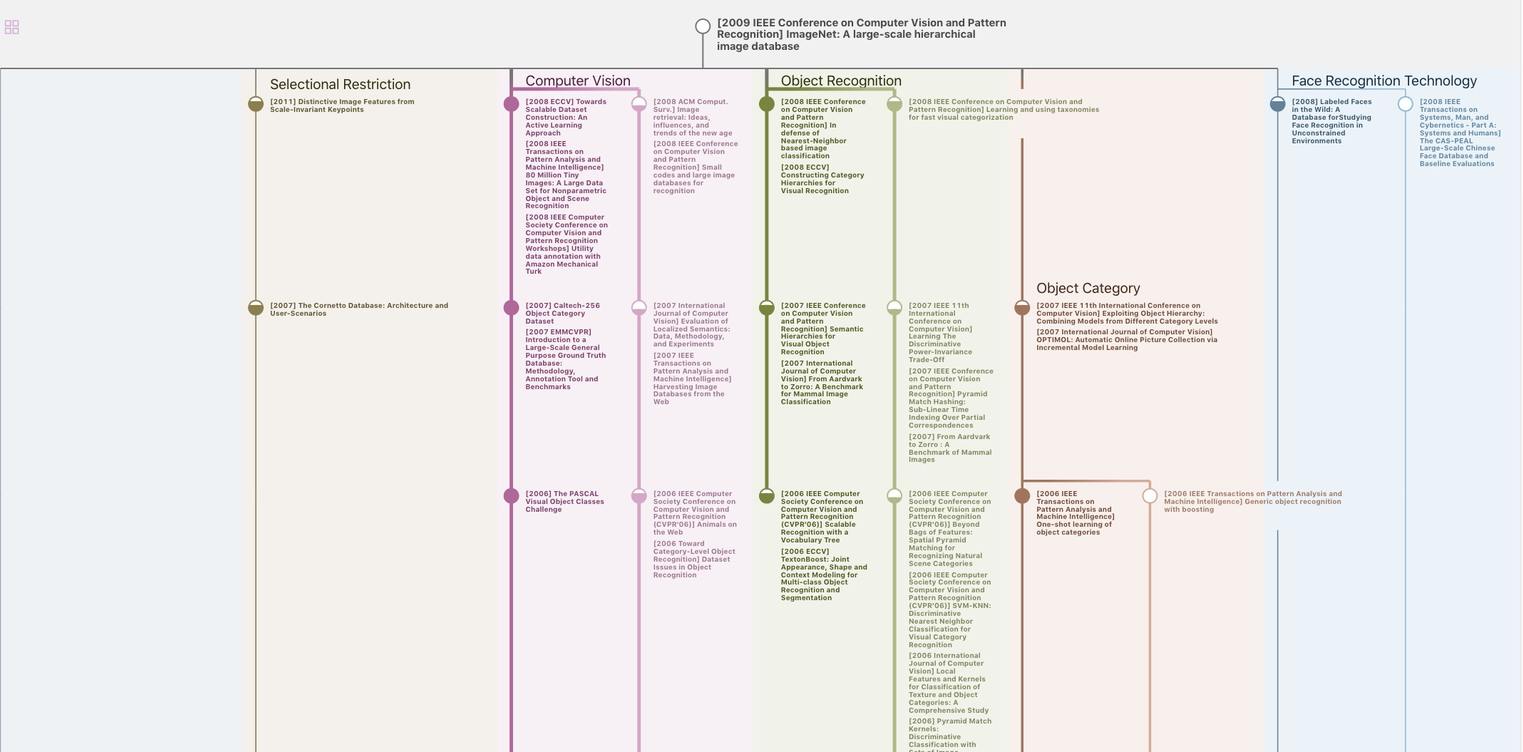
生成溯源树,研究论文发展脉络
Chat Paper
正在生成论文摘要