Meta-learning for imbalanced data and classification ensemble in binary classification
Neurocomputing(2009)
摘要
To conduct binary classification with highly imbalanced data is a very common problem, especially when the examples of interest are relatively rare. In this paper, we proposed the ''Meta Imbalanced Classification Ensemble (MICE)'' algorithm in order to dilute the effect of imbalanced data. In the MICE, the majority group is partitioned based on the transformed features from ''inner product'' to retain the geometric relation between two groups. The empirical results show that the performance of MICE is better than some renowned classification methods in terms of the specificity and the sensitivity.
更多查看译文
关键词
area under roc curve,empirical result,geometric relation,fisher (linear) discriminant analysis,imbalanced data,meta imbalanced classification ensemble,majority group,inner product,common problem,classification ensemble,roc curve,meta-learning,binary classification,renowned classification method
AI 理解论文
溯源树
样例
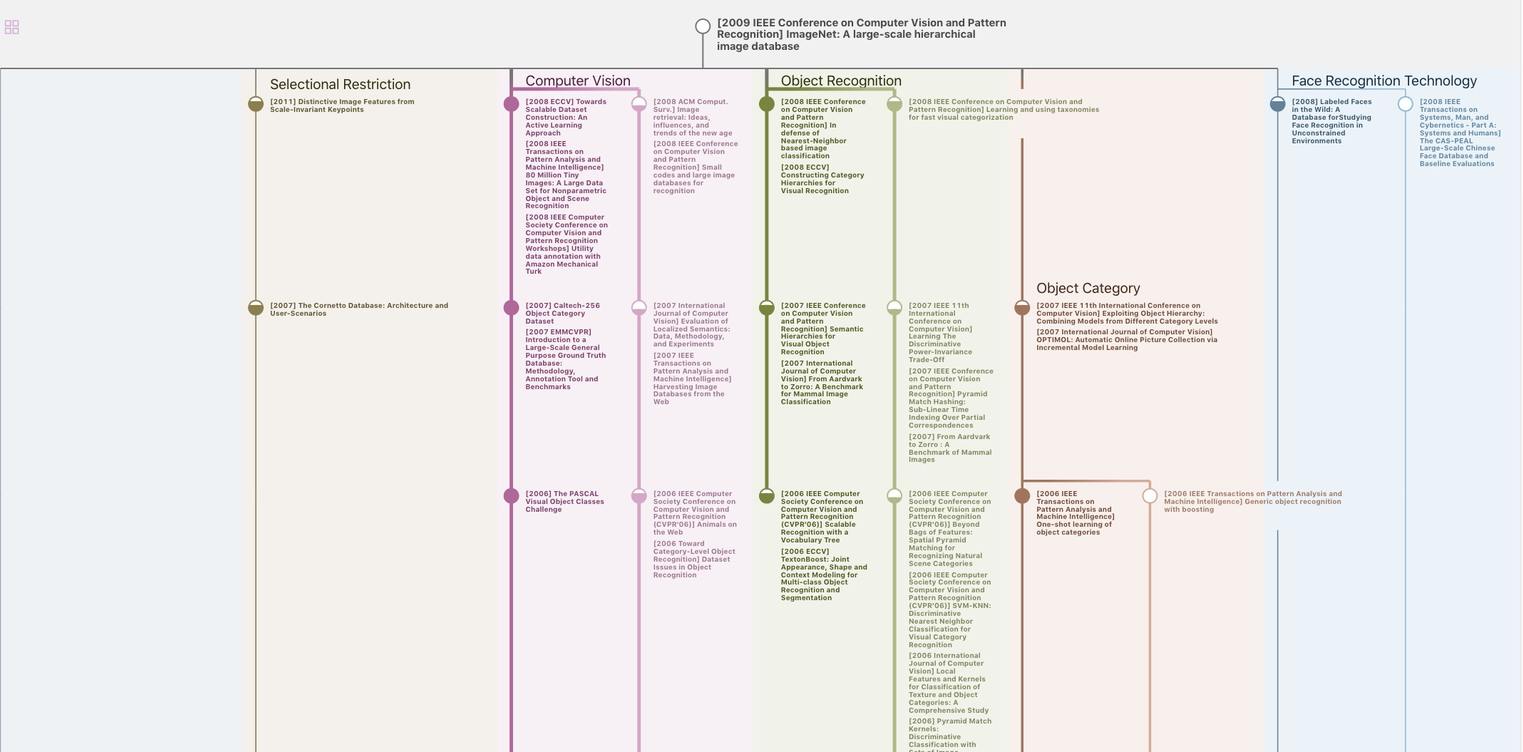
生成溯源树,研究论文发展脉络
Chat Paper
正在生成论文摘要