User variance and its impact on video retrieval benchmarking
CIVR '09: Proceedings of the ACM International Conference on Image and Video Retrieval(2009)
摘要
In this paper, we describe one of the largest multi-site interactive video retrieval experiments conducted in a laboratory setting. Interactive video retrieval performance is difficult to cross-compare as variables exist across users, interfaces and the underlying retrieval engine. Conducted within the framework of TRECVID 2008, we completed a multi-site, multi-interface experiment. Three institutes participated involving 36 users, 12 each from Dublin City University (DCU, Ireland), University of Glasgow (GU, Scotland) and Centrum Wiskunde & Informatica (CWI, the Netherlands). Three user interfaces were developed which all used the same search service. Using a latin squares arrangement, each user completed 12 topics, leading to 6 TRECVID runs per site, 18 in total. This allowed us to isolate the factors of users and interfaces from retrieval performance. In this paper we present an analysis of both the quantitative and qualitative data generated from this experiment, demonstrating that for interactive video retrieval with "novice" users, performance can vary by up to 300% for the same system using different sets of users, whilst differences in performance of interface variants was in comparison not statistically different. Our results have implications for the manner in which interactive video retrieval experiments using non-expert users are evaluated. The primary focus of this paper is in highlighting that non-expert users generate very large performance fluctuations, which may either mask or create system variability. The discussion of why this happened is not covered by this paper.
更多查看译文
关键词
different set,underlying retrieval engine,retrieval performance,non-expert user,largest multi-site interactive video,large performance fluctuation,interactive video retrieval experiment,video retrieval benchmarking,retrieval experiment,interactive video retrieval performance,user variance,interactive video retrieval,information retrieval
AI 理解论文
溯源树
样例
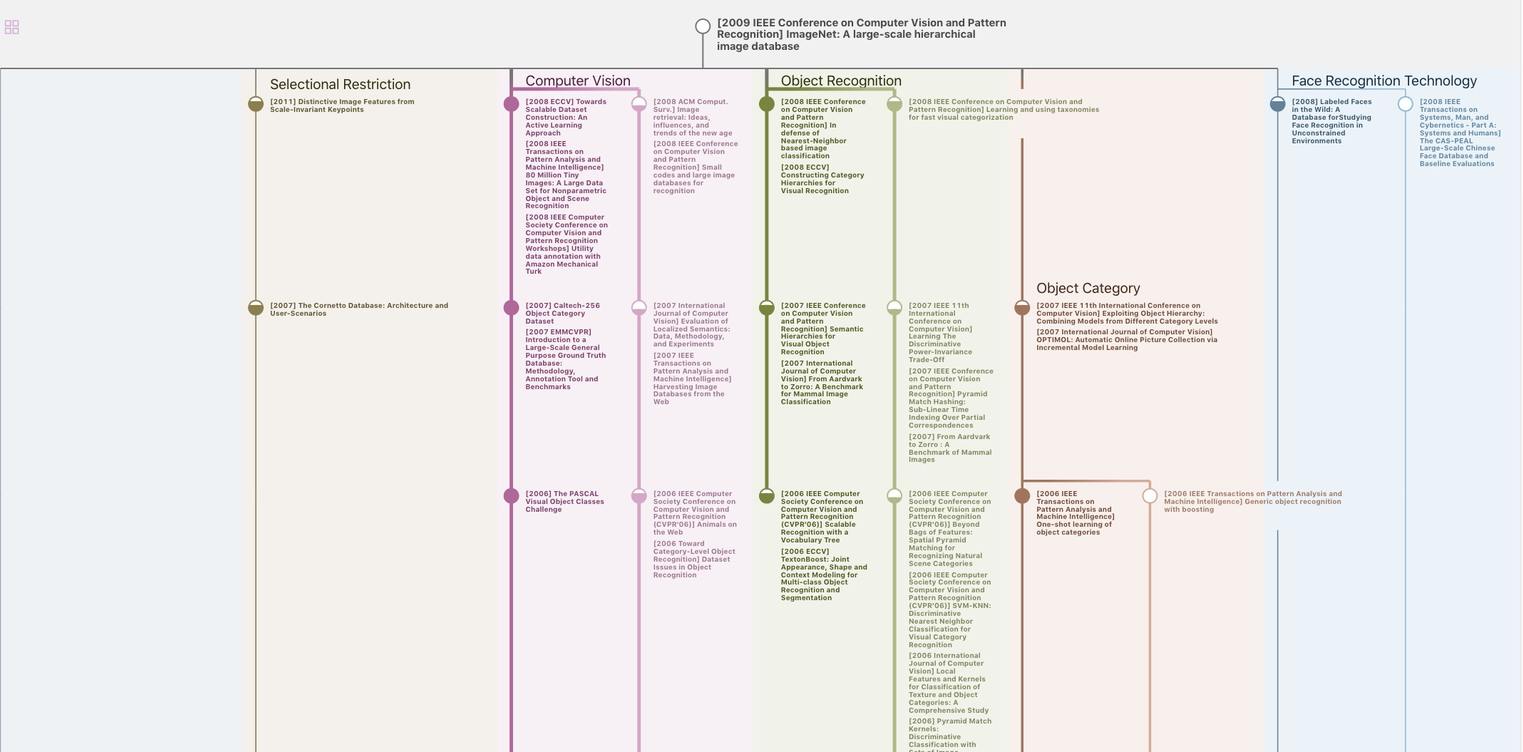
生成溯源树,研究论文发展脉络
Chat Paper
正在生成论文摘要