Nonlinear Embedded Map Projection for Dimensionality Reduction
IMAGE ANALYSIS AND PROCESSING - ICIAP 2009, PROCEEDINGS(2009)
摘要
We describe a dimensionality reduction method used to perform similarity search that is tested on document image retrieval applications. The approach is based on data point projection into a low dimensional space obtained by merging together the layers of a Growing Hierarchical Self Organizing Map (GHSOM) trained to model the distribution of objects to be indexed. The low dimensional space is defined by embedding the GHSOM sub-maps in the space defined by a non-linear mapping of neurons belonging to the first level map. The latter mapping is computed with the Sammon projection algorithm. The dimensionality reduction is used in a similarity search framework whose aim is to efficiently retrieve similar objects on the basis of the distance among projected points corresponding to high dimensional feature vectors describing the indexed objects. We compare the proposed method with other dimensionality reduction techniques by evaluating the retrieval performance on three datasets.
更多查看译文
关键词
dimensionality reduction,dimensionality reduction technique,ghsom sub-maps,latter mapping,sammon projection algorithm,dimensionality reduction method,data point projection,document image retrieval application,map projection,high dimensional feature,low dimensional space,indexation,similarity search,feature vector,image retrieval
AI 理解论文
溯源树
样例
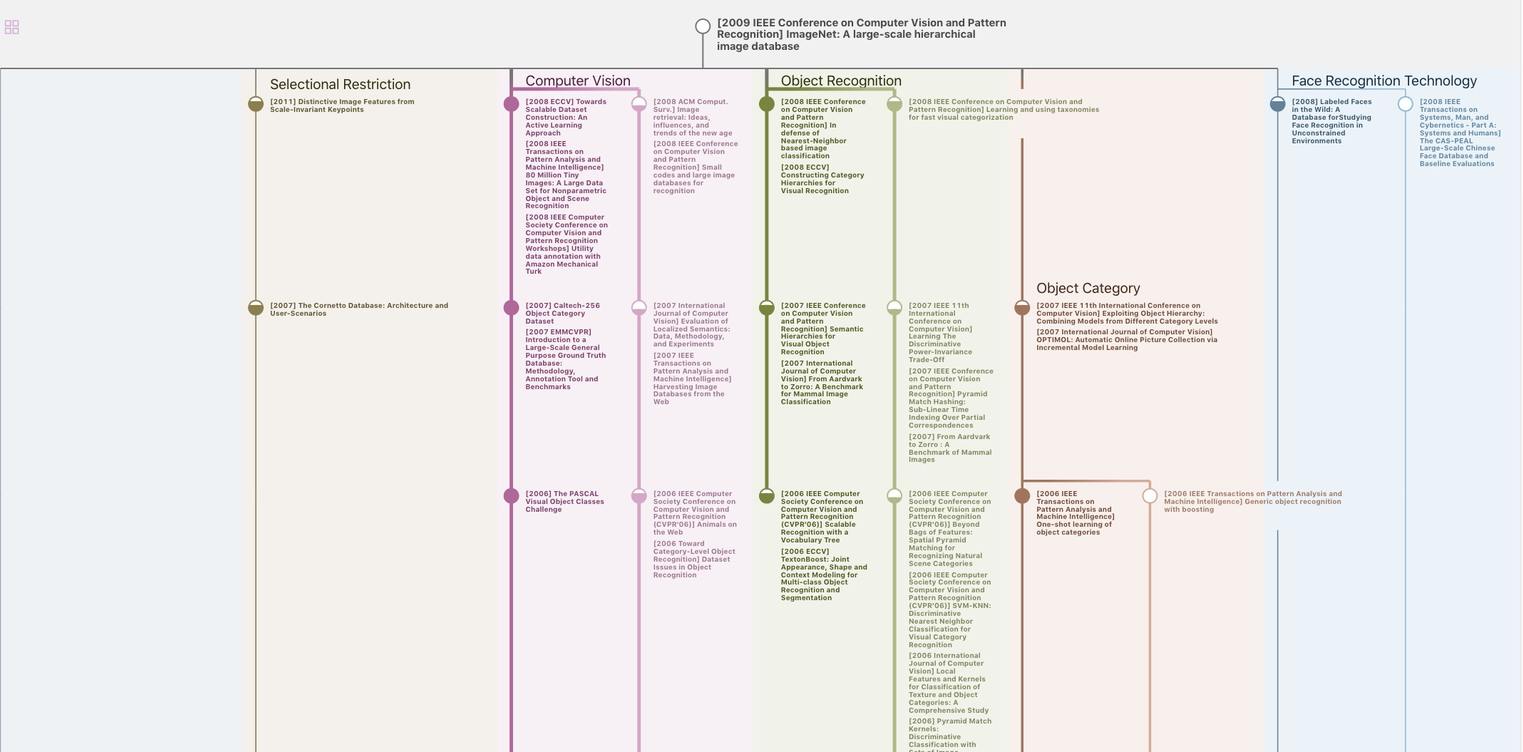
生成溯源树,研究论文发展脉络
Chat Paper
正在生成论文摘要