Topic Significance Ranking Of Lda Generative Models
MACHINE LEARNING AND KNOWLEDGE DISCOVERY IN DATABASES, PT I(2009)
摘要
Topic models, like Latent Dirichlet Allouation (LDA), have been recently used to automatically generate text, corpora topics, and to subdivide the corpus words among those topics. However, not all the estimated topics are of equal importance or correspond to genuine themes of the domain. Some of the topics can be a collection of irrelevant words, or represent insignificant themes. Current approaches to topic modeling perform mannal examination to find meaningful topics. This paper presents the first automated unsupervised analysis of LDA models to identify junk topics from legitimate ones. and to rank the topic significance. Basically, the distance between a topic distribution and three definitions of "junk distribution" is computed using a variety or measures, from which an expressive figure of the topic significance is implemented using 4-phase Weighted Combination approach. Our experiments on synthetic and benchmark datasets show the effectiveness of the proposed approach in ranking the topic significance.
更多查看译文
关键词
topic significance,estimated topic,junk topic,meaningful topic,text corpora topic,topic distribution,topic model,topic modeling,4-phase Weighted Combination approach,LDA model,LDA Generative Models,Topic Significance
AI 理解论文
溯源树
样例
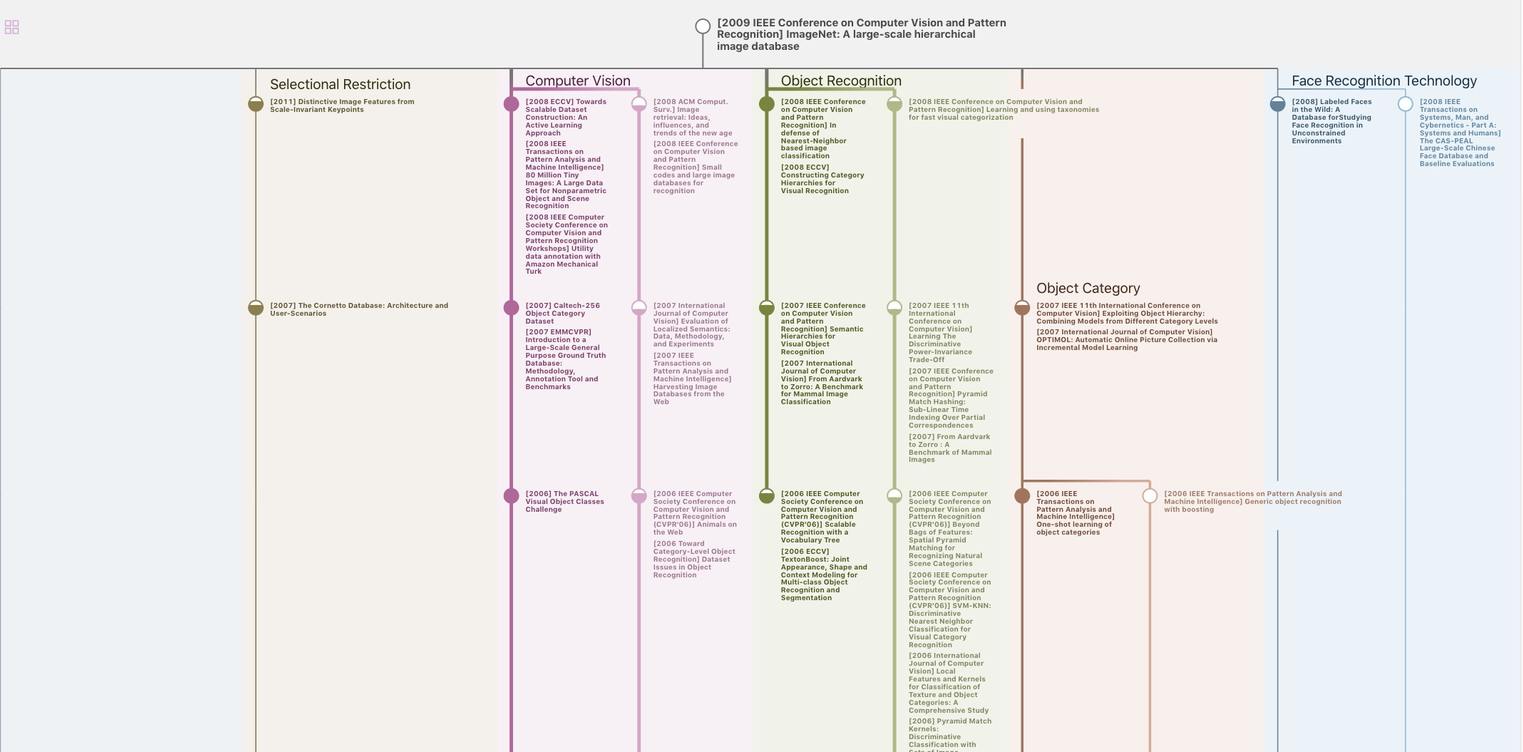
生成溯源树,研究论文发展脉络
Chat Paper
正在生成论文摘要