On AIRS and Clonal Selection for Machine Learning
ARTIFICIAL IMMUNE SYSTEMS, PROCEEDINGS(2009)
摘要
AIRS is an immune-inspired supervised learning algorithm that has been shown to perform competitively on some common datasets. Previous analysis of the algorithm consists almost exclusively of empirical benchmarks and the reason for its success remains somewhat speculative. In this paper, we decouple the statistical and immunological aspects of AIRS and consider their merits individually. This perspective allows us to clarifying why AIRS performs as it does and identify deficiencies that leave AIRS lacking. A comparison with Radial Basis Functions suggests that each may have something to offer the other.
更多查看译文
关键词
empirical benchmarks,previous analysis,common datasets,machine learning,immune-inspired supervised learning algorithm,clonal selection,immunological aspect,radial basis functions,radial basis function,supervised learning
AI 理解论文
溯源树
样例
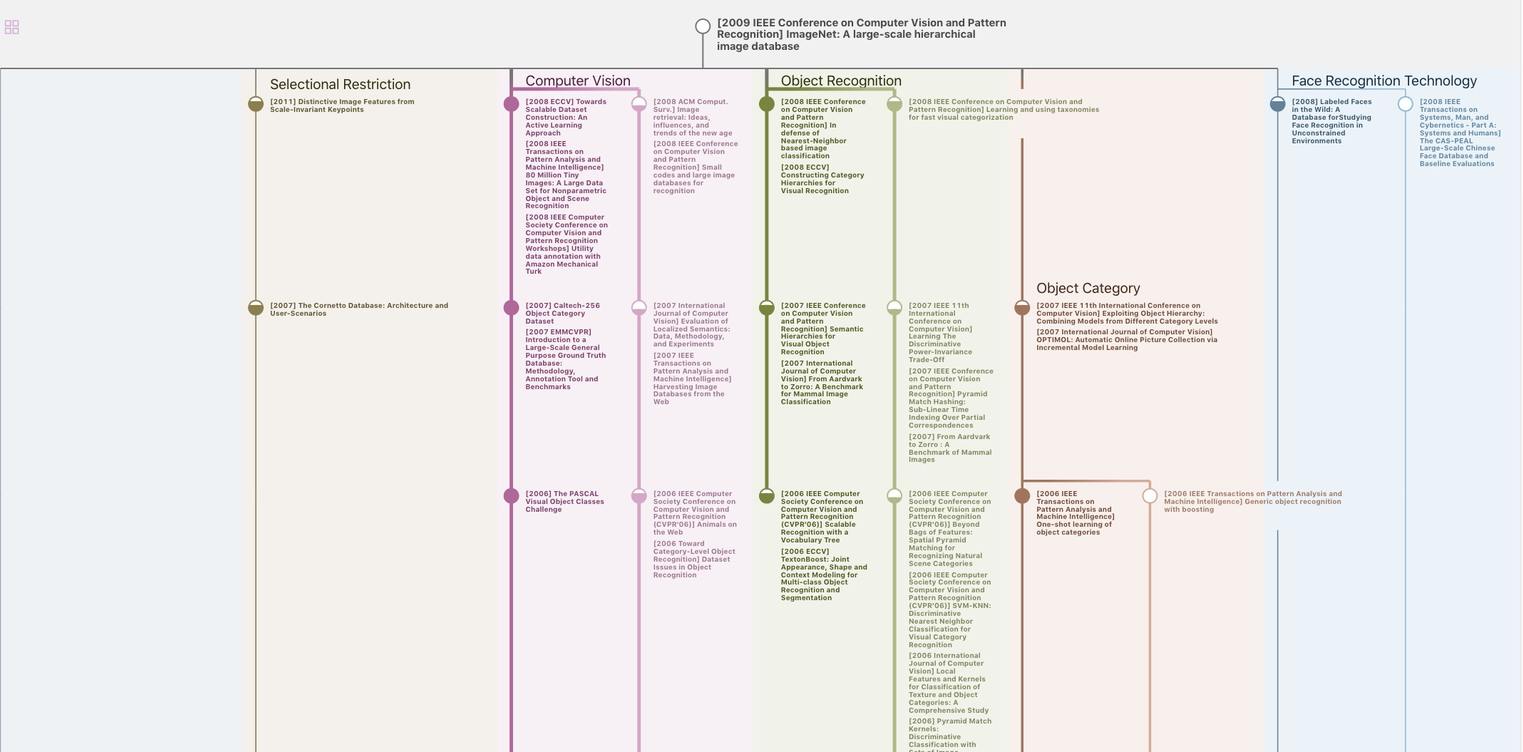
生成溯源树,研究论文发展脉络
Chat Paper
正在生成论文摘要