Exploiting Domain Knowledge by Automated Taxonomy Generation in Recommender Systems
E-COMMERCE AND WEB TECHNOLOGIES, PROCEEDINGS(2009)
摘要
The effectiveness of incorporating domain knowledge into recommender systems to address their sparseness problem and improve their prediction accuracy has been discussed in many research works. However, this technique is usually restrained in practice because of its high computational expense. Although cluster analysis can alleviate the computational complexity of the recommendation procedure, it is not satisfactory in preserving pair-wise item similarities, which would severely impair the recommendation quality. In this paper, we propose an efficient approach based on the technique of Automated Taxonomy Generation to exploit relational domain knowledge in recommender systems so as to achieve high system scalability and prediction accuracy. Based on the domain knowledge, a hierarchical data model is synthesized in an offline phase to preserve the original pairwise item similarities. The model is then used by online recommender systems to facilitate the similarity calculation and keep their recommendation quality comparable to those systems by means of real-time exploiting domain knowledge. Experiments were conducted upon real datasets to evaluate our approach.
更多查看译文
关键词
efficient approach,online recommender system,prediction accuracy,recommender system,relational domain knowledge,hierarchical data model,exploiting domain knowledge,automated taxonomy generation,recommendation quality,recommendation procedure,recommender systems,domain knowledge,computational complexity,hierarchical data,cluster analysis,real time
AI 理解论文
溯源树
样例
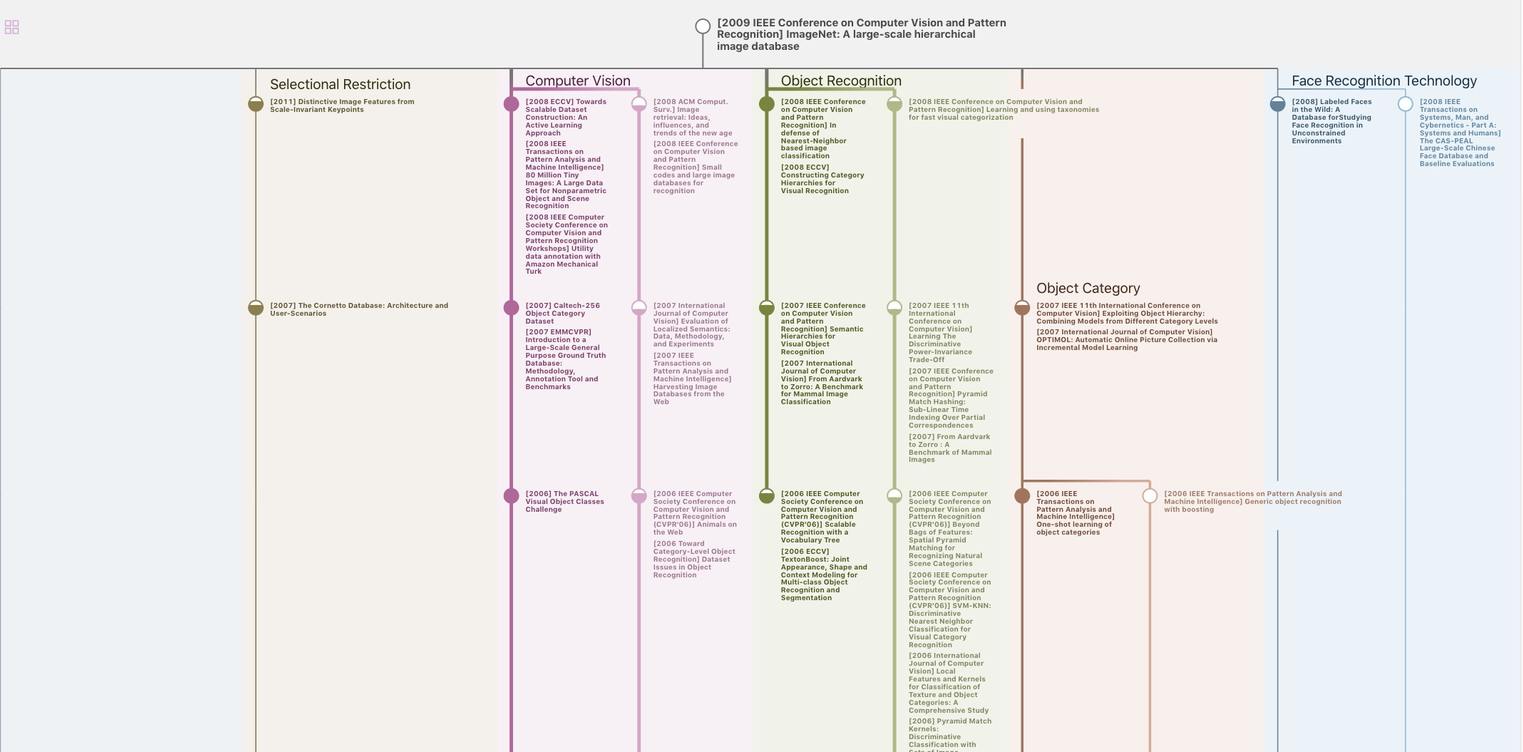
生成溯源树,研究论文发展脉络
Chat Paper
正在生成论文摘要