Combining mixture weight pruning and quantization for small-footprint speech recognition
Taipei(2009)
摘要
Semi-continuous acoustic models, where the output distributions for all Hidden Markov Model states share a common codebook of Gaussian density functions, are a well-known and proven technique for reducing computation in automatic speech recognition. However, the size of the parameter files, and thus their memory footprint at runtime, can be very large. We demonstrate how non-linear quantization can be combined with a mixture weight distribution pruning technique to halve the size of the models with minimal performance overhead and no increase in error rate.
更多查看译文
关键词
hidden Markov models,quantisation (signal),speech recognition,Gaussian density functions,automatic speech recognition,codebook,error rate,hidden Markov model,memory footprint,mixture weight pruning,nonlinear quantization,quantization,semicontinuous acoustic models,small-footprint speech recognition,Data compression,Quantization,Speech recognition
AI 理解论文
溯源树
样例
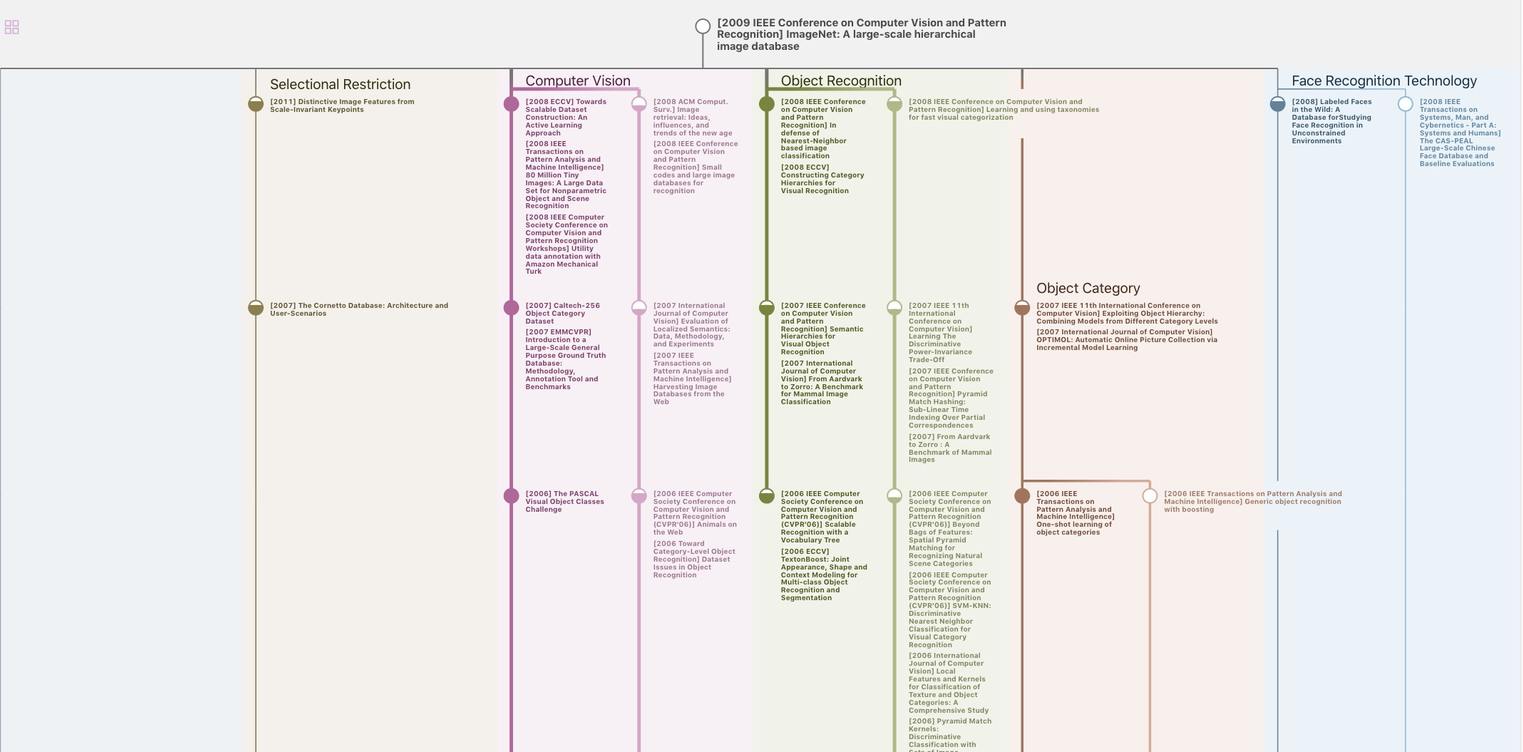
生成溯源树,研究论文发展脉络
Chat Paper
正在生成论文摘要