Noise adaptive training using a vector taylor series approach for noise robust automatic speech recognition
Taipei(2009)
摘要
In traditional methods for noise robust automatic speech recognition, the acoustic models are typically trained using clean speech or using multi-condition data that is processed by the same feature enhancement algorithm expected to be used in decoding. In this paper, we propose a noise adaptive training (NAT) algorithm that can be applied to all training data that normalizes the environmental distortion as part of the model training. In contrast to the feature enhancement methods, NAT estimates the underlying “pseudo-clean” model parameters directly without relying on point estimates of the clean speech features as an intermediate step. The pseudo-clean model parameters learned with NAT are later used with vector Taylor series (VTS) model adaptation for decoding noisy utterances at test time. Experiments performed on the Aurora 2 and Aurora 3 tasks, demonstrate that the proposed NAT method obtain relative improvements of 18.83% and 32.02%, respectively, over VTS model adaptation.
更多查看译文
关键词
proposed nat method,pseudo-clean model parameter,vts model adaptation,noise robust automatic speech,model adaptation,vector taylor series approach,model parameter,noise adaptive training,clean speech,acoustic model,clean speech feature,model training,decoding,nonlinear equations,speech processing,noise measurement,speech,automatic speech recognition,noise,point estimation,data models,indexing terms,taylor series,network address translation,acoustic noise,speech recognition,hidden markov models
AI 理解论文
溯源树
样例
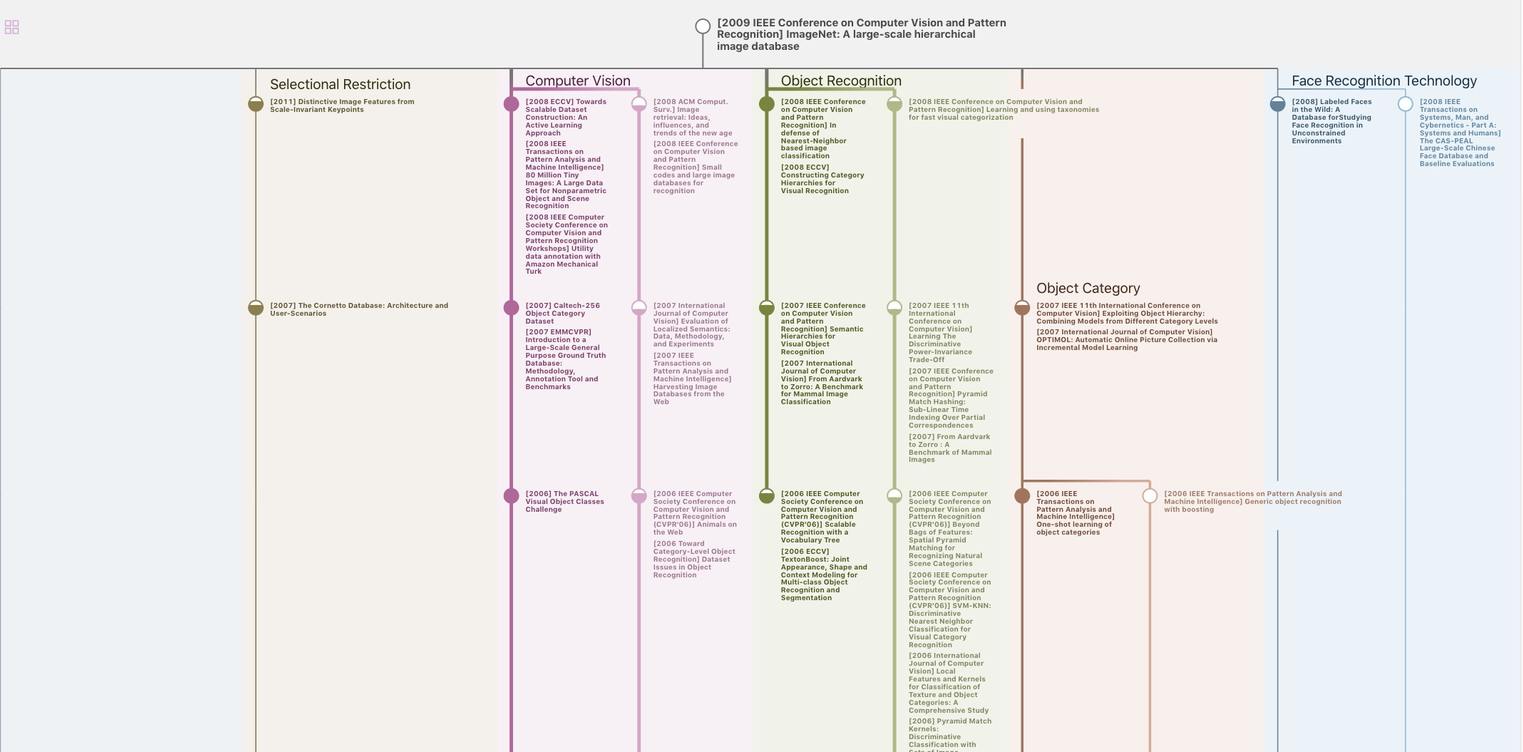
生成溯源树,研究论文发展脉络
Chat Paper
正在生成论文摘要