Experimenting with a global decision tree for state clustering in automatic speech recognition systems
Taipei(2009)
摘要
In modern automatic speech recognition systems, it is standard practice to cluster several logical hidden Markov model states into one physical, clustered state. Typically, the clustering is done such that logical states from different phones or different states can not share the same clustered state. In this paper, we present a collection of experiments that lift this restriction. The results show that, for Aurora 2 and Aurora 3, much smaller models perform as least as well as the standard baseline. On a TIMIT phone recognition task, we analyze the tying structures introduced, and discuss the implications for building better acoustic models.
更多查看译文
关键词
phonetic decision tree,different state,standard baseline,global decision tree,automatic speech recognition system,modern automatic speech recognition,acoustic model,acoustic model- ing,standard practice,timit phone recognition task,logical hidden markov model,logical state,index terms— automatic speech recognition,different phone,smaller model,automatic speech recognition,acoustics,decision tree,concrete,indexing terms,training data,hidden markov models,speech,context modeling,hidden markov model,speech recognition,decision trees,accuracy
AI 理解论文
溯源树
样例
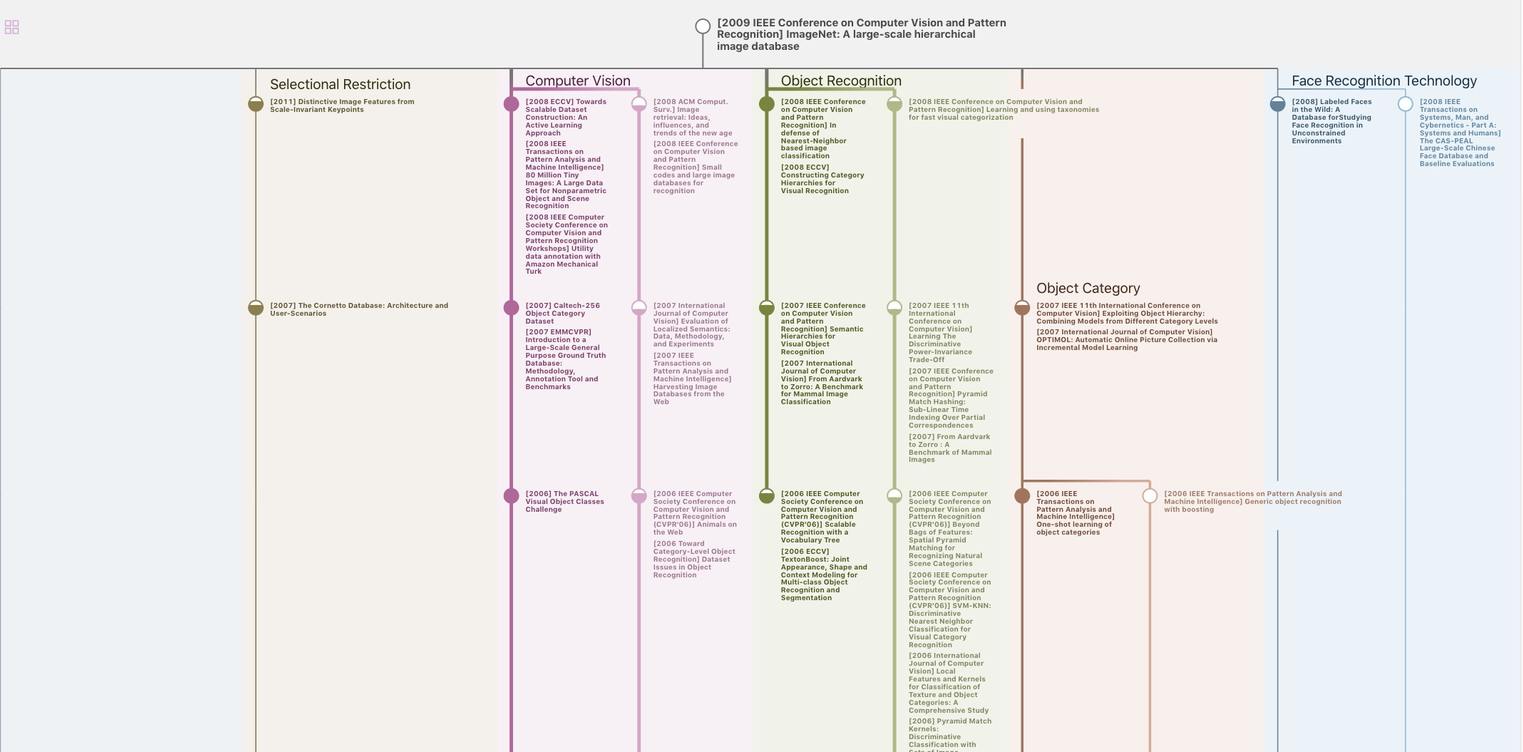
生成溯源树,研究论文发展脉络
Chat Paper
正在生成论文摘要