Nonparametric Monotone Classification with MOCA
Pisa(2008)
摘要
We describe a monotone classification algorithm called MOCA that attempts to minimize the mean absolute prediction error for classification problems with ordered class labels.We first find a monotone classifier with minimum L1 loss on the training sample, and then use a simple interpolation scheme to predict the class labels for attribute vectors not present in the training data.We compare MOCA to the ordinal stochastic dominance learner (OSDL), on artificial as well as real data sets. We show that MOCA often outperforms OSDL with respect to mean absolute prediction error.
更多查看译文
关键词
data mining,interpolation,stochastic processes,MOCA,attribute vectors,interpolation scheme,mean absolute prediction error,minimum L1 loss,nonparametric monotone classification,ordinal stochastic dominance learner,Classification,Monotonicity Constraint
AI 理解论文
溯源树
样例
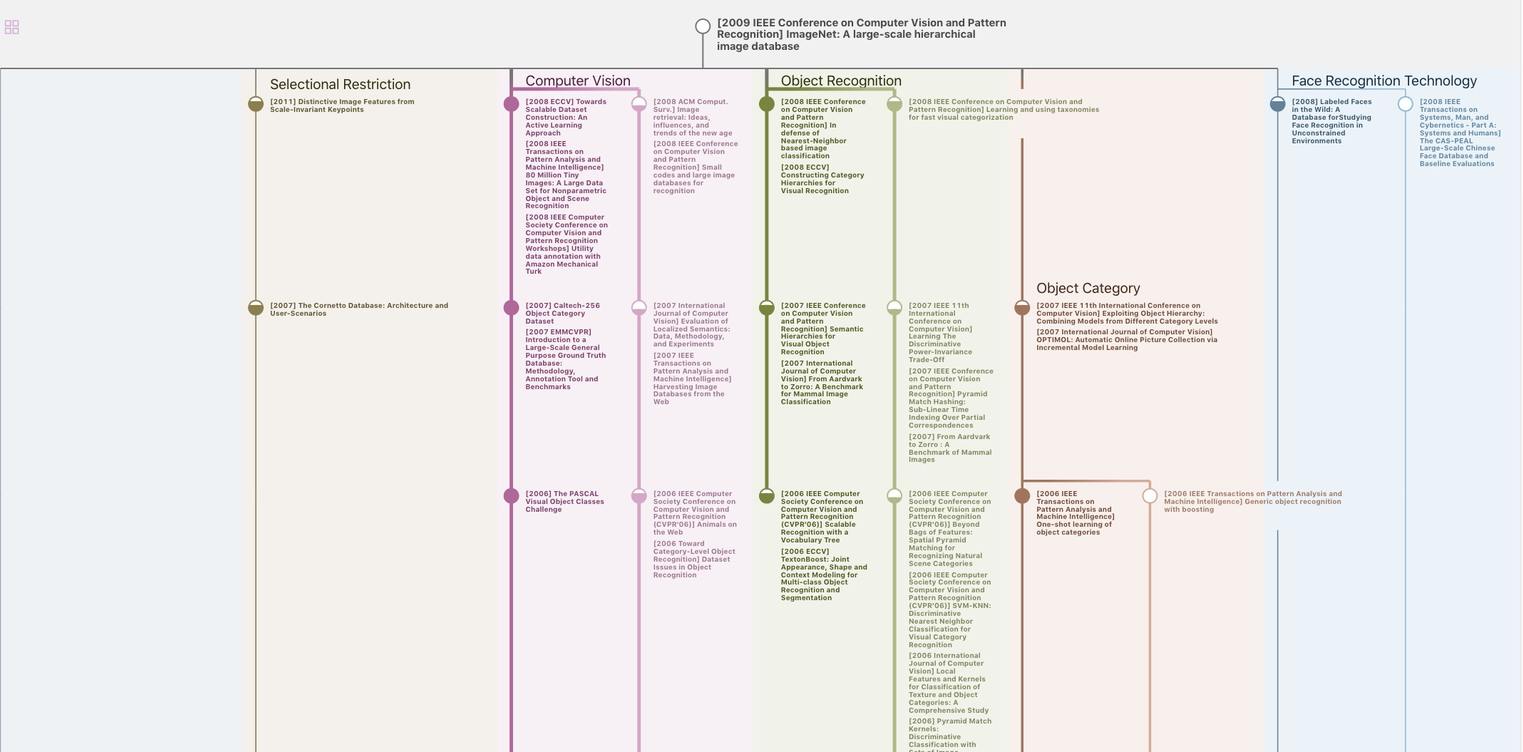
生成溯源树,研究论文发展脉络
Chat Paper
正在生成论文摘要