On-line LDA: Adaptive Topic Models for Mining Text Streams with Applications to Topic Detection and Tracking
Pisa(2008)
摘要
This paper presents Online Topic Model (OLDA), a topic model that automatically captures the thematic patterns and identifies emerging topics of text streams and their changes over time. Our approach allows the topic modeling framework, specifically the Latent Dirichlet Allocation (LDA) model, to work in an online fashion such that it incrementally builds an up-to-date model (mixture of topics per document and mixture of words per topic) when a new document (or a set of documents) appears. A solution based on the Empirical Bayes method is proposed. The idea is to incrementally update the current model according to the information inferred from the new stream of data with no need to access previous data. The dynamics of the proposed approach also provide an efficient mean to track the topics over time and detect the emerging topics in real time. Our method is evaluated both qualitatively and quantitatively using benchmark datasets. In our experiments, the OLDA has discovered interesting patterns by just analyzing a fraction of data at a time. Our tests also prove the ability of OLDA to align the topics across the epochs with which the evolution of the topics over time is captured. The OLDA is also comparable to, and sometimes better than, the original LDA in predicting the likelihood of unseen documents.
更多查看译文
关键词
topic modeling framework,new stream,mining text streams,previous data,real time,up-to-date model,new document,adaptive topic models,on-line lda,original lda,current model,topic model,empirical bayes method,topic detection,latent dirichlet allocation,text analysis,probability density function,data models,data mining,computational modeling,history
AI 理解论文
溯源树
样例
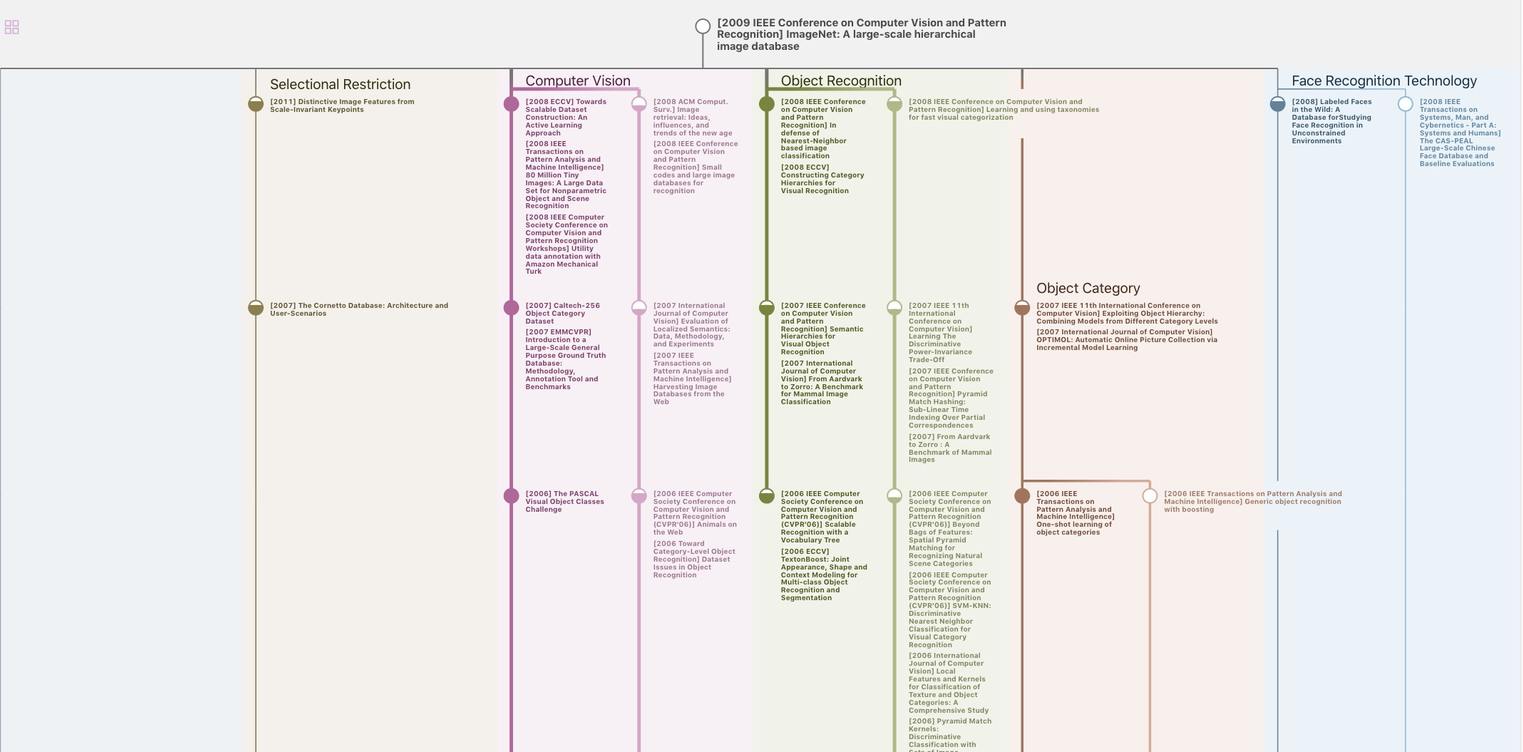
生成溯源树,研究论文发展脉络
Chat Paper
正在生成论文摘要