Nonlinear Function Approximation Based on Least Wilcoxon Takagi-Sugeno Fuzzy Model
Intelligent Systems Design and Applications, 2008. ISDA '08. Eighth International Conference(2008)
摘要
The purpose of this paper is based on radial basis function neural network (RBFN) to develop a self-constructing least Wilcoxon-generalized RBFN fuzzy inference system (LW-GRBFNFIS) and applied to nonlinear function approximation and chaotic time series prediction. As is well known in statistics, the resulting linear function by using the rank-based least Wilcoxon (LW) norm approximate to linear function problems are usually robust against (or insensitive to) outliers. In addition, the Takagi-Sugeno fuzzy model and RBFN techniques have been proved the functional equivalence and successfully applied to modeling nonlinear function approximation problems. This paper introduce proposed method to improve traditional least square GRBFNFIS for nonlinear function approximation and overcome outliers' problem. Nonlinear function approximation and chaotic time series prediction problems used to verify the proposed method. The experiments results show the proposed method can effectively solve outliers' problems.
更多查看译文
关键词
nonlinear function approximation,wilcoxon takagi-sugeno fuzzy model,linear function,fuzzy reasoning,radial basis function networks,radial basis function neural,statistics,chaos,chaotic time series prediction,wilcoxon-generalized rbfn fuzzy inference,fuzzy inference system,radial basis function neural network,function approximation,mathematics computing,least squares approximations,fuzzy systems,nonlinear function approximation problem,nonlinear functions,least wilcoxon takagi-sugeno fuzzy model,rbfn technique,least square method,time series,linear function problem,rbfn,least squares approximation,least square,data models,time series analysis
AI 理解论文
溯源树
样例
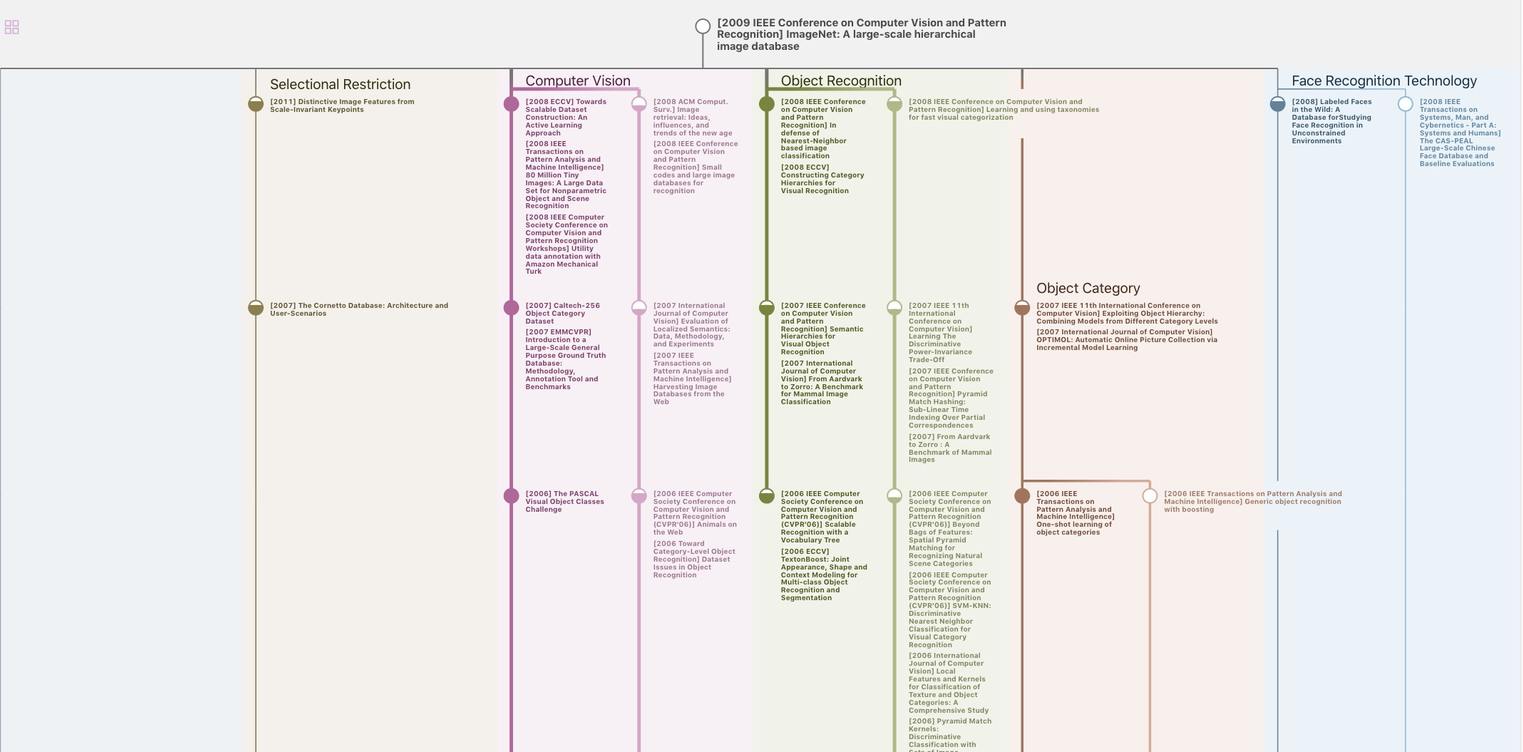
生成溯源树,研究论文发展脉络
Chat Paper
正在生成论文摘要