A Comparison between Neural Network Methods for Learning Aggregate Functions
DISCOVERY SCIENCE, PROCEEDINGS(2008)
摘要
In various application domains, data can be represented as bags of vectors instead of single vectors. Learning aggregate functions from such bags is a challenging problem. In this paper, a number of simple neural network approaches and a combined approach based on cascade-correlation are examined in order to handle this kind of data. Adapted feedforward networks, recurrent networks and networks with special aggregation units integrated in the network can all be used to construct networks that are capable of learning aggregate function. A combination of these three approaches is possible by using cascade-correlation, creating a method that automatically chooses the best of these options. Results on artificial and multi-instance data sets are reported, allowing a comparison between the different approaches.
更多查看译文
关键词
recurrent network,learning aggregate functions,neural network methods,multi-instance data set,aggregate function,different approach,combined approach,challenging problem,feedforward network,special aggregation unit,simple neural network approach,single vector,neural network
AI 理解论文
溯源树
样例
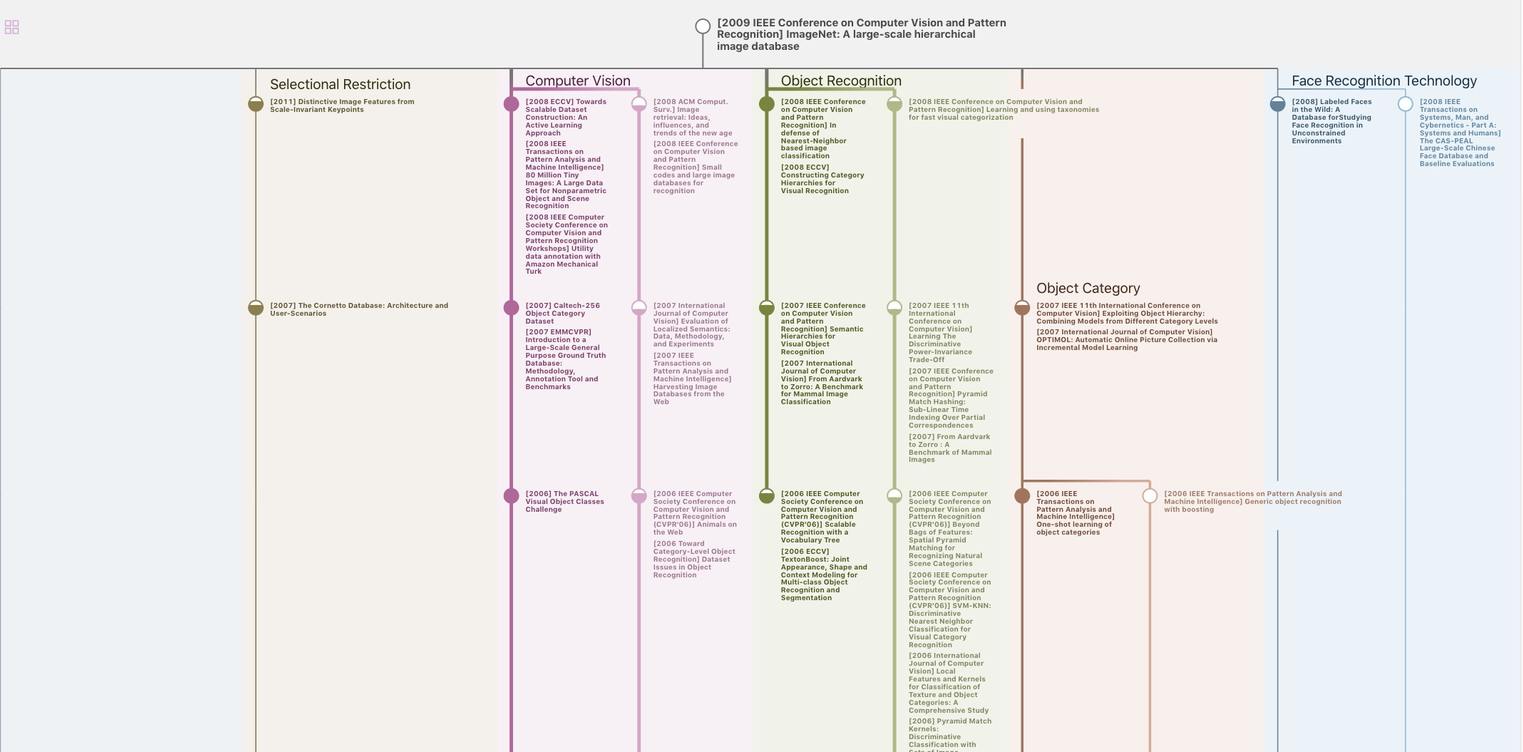
生成溯源树,研究论文发展脉络
Chat Paper
正在生成论文摘要