On the Combination of Logistic Regression and Local Probability Estimates
Gauteng(2009)
摘要
Classifiers based on parametric or non-parametric learning methods have different advantages and disadvantages. To take advantage of the strengths of both methods, we propose an algorithm that combines a parametric model (logistic regression) with a non-parametric classification method (k-nearest neighbors). This combination is based on a measure of appropriateness that uses a heuristic to decide which of the two components should contribute more to the final classification output. We measure the performance of this combination method on two data sets (one from medical informatics, and one consisting of simulated data) in terms of areas under the ROC curves (AUCs). We are able to demonstrate that our method of combining classifiers exceeds the performance of both individual classifiers taken separately.
更多查看译文
关键词
parametric model,logistic regression,local probability estimates,roc curve,simulated data,individual classifier,combination method,k-nearest neighbor,different advantage,final classification output,non-parametric classification method,machine learning
AI 理解论文
溯源树
样例
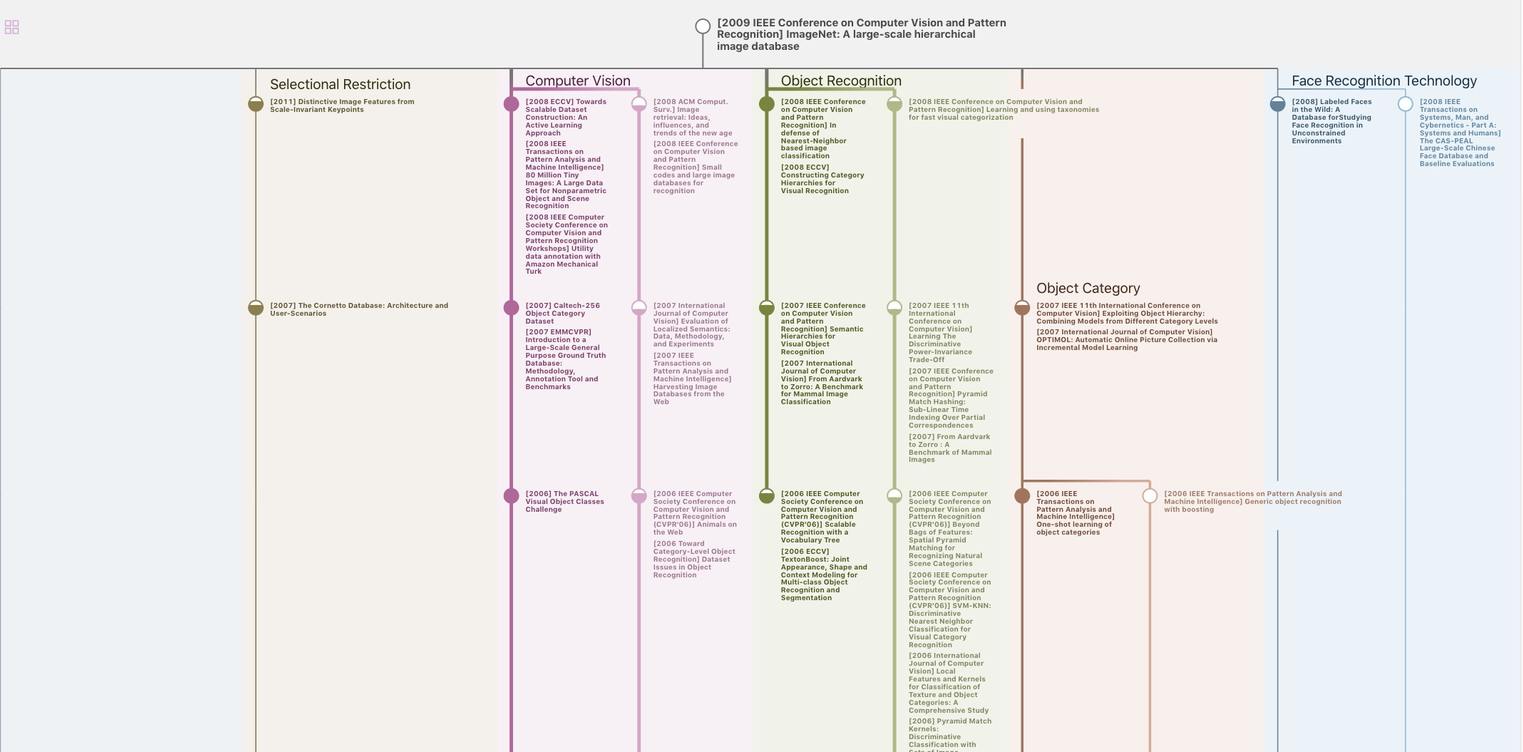
生成溯源树,研究论文发展脉络
Chat Paper
正在生成论文摘要