A prediction algorithm for time series based on adaptive model selection
Expert Systems with Applications(2009)
摘要
HMM (Hidden Markov model) has been used successfully to analyze various types of time series. To fit time series with HMM, the number of hidden states should be determined before learning other parameters, since it has great impact on the complexity and precision of the fitting HMM. However this becomes too difficult when there is not enough prior knowledge about the observed series, which will lead to the increasing mean error in prediction process. To overcome this shortcoming, a prediction algorithm PAAMS for time series based on adaptive model selection is proposed. In PAAMS, the model can be dynamically updated when the prediction mean error increases. During the update process, an automatic model selection method AMSA is applied to get the best hidden state number and other model parameters. The proposed method AMSA is based on clustering, in which the number of hidden states is considered as the number of clusters. The feasibility and effectiveness of proposed prediction algorithm are explained. Experiments on American stock price data set are done and the results show that the PAAMS algorithm can achieve higher precision than that of previous study on the same data sets based on fixed model techniques.
更多查看译文
关键词
automatic model selection method,time series,prediction algorithm paams,fixed model technique,adaptive model selection,hidden markov model,model parameter,observed series,time series prediction,hidden state,hidden state number,model selection,mean error
AI 理解论文
溯源树
样例
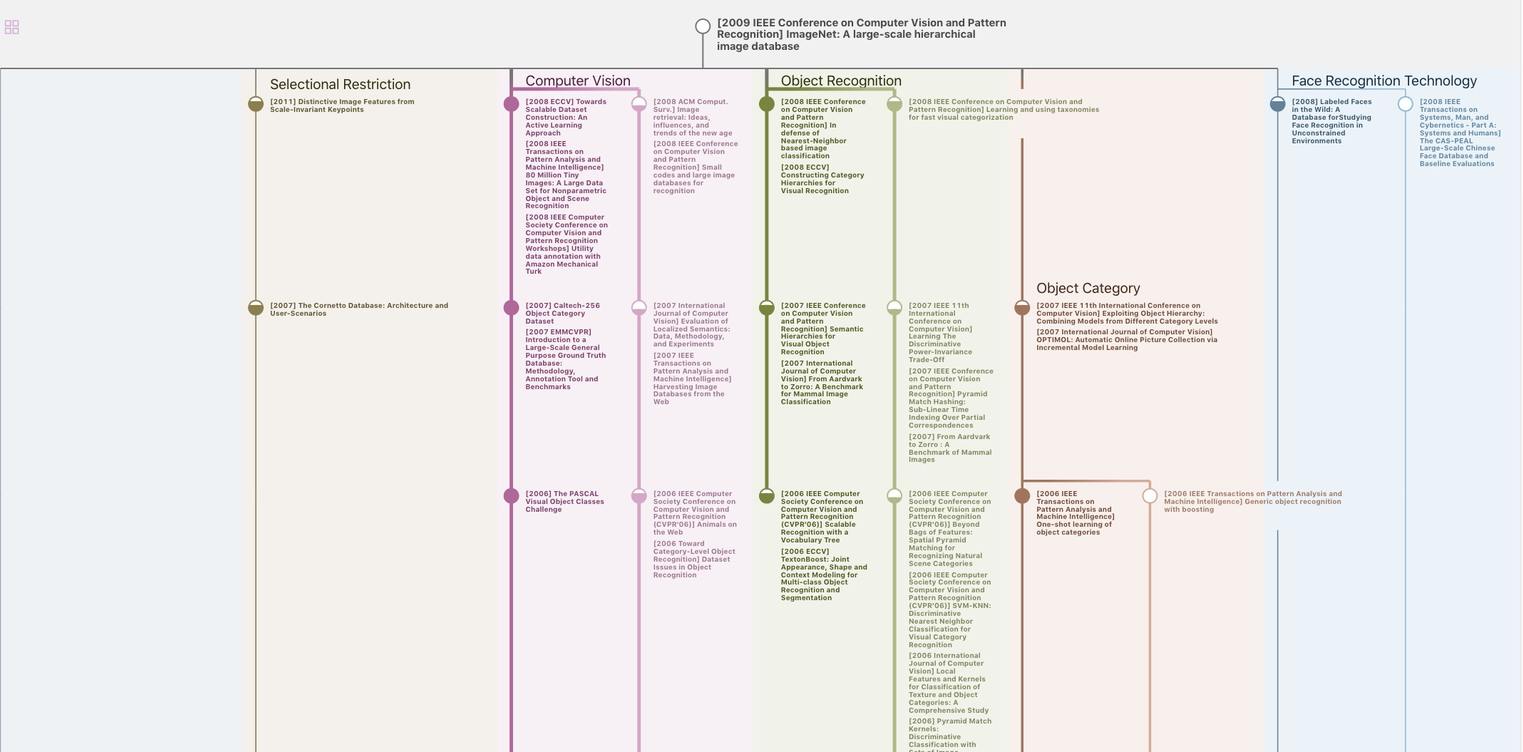
生成溯源树,研究论文发展脉络
Chat Paper
正在生成论文摘要