Kernel least mean square algorithm with constrained growth
Signal Processing(2009)
摘要
The linear least mean squares (LMS) algorithm has been recently extended to a reproducing kernel Hilbert space, resulting in an adaptive filter built from a weighted sum of kernel functions evaluated at each incoming data sample. With time, the size of the filter as well as the computation and memory requirements increase. In this paper, we shall propose a new efficient methodology for constraining the increase in length of a radial basis function (RBF) network resulting from the kernel LMS algorithm without significant sacrifice on performance. The method involves sequential Gaussian elimination steps on the Gram matrix to test the linear dependency of the feature vector corresponding to each new input with all the previous feature vectors. This gives an efficient method of continuing the learning as well as restricting the number of kernel functions used.
更多查看译文
关键词
new efficient methodology,linear dependency,kernel lms algorithm,square algorithm,new input,efficient method,reproducing kernel hilbert space,kernel function,memory requirements increase,feature vector,previous feature vector,adaptive filter,least mean square,radial basis function,lms algorithm,gaussian elimination
AI 理解论文
溯源树
样例
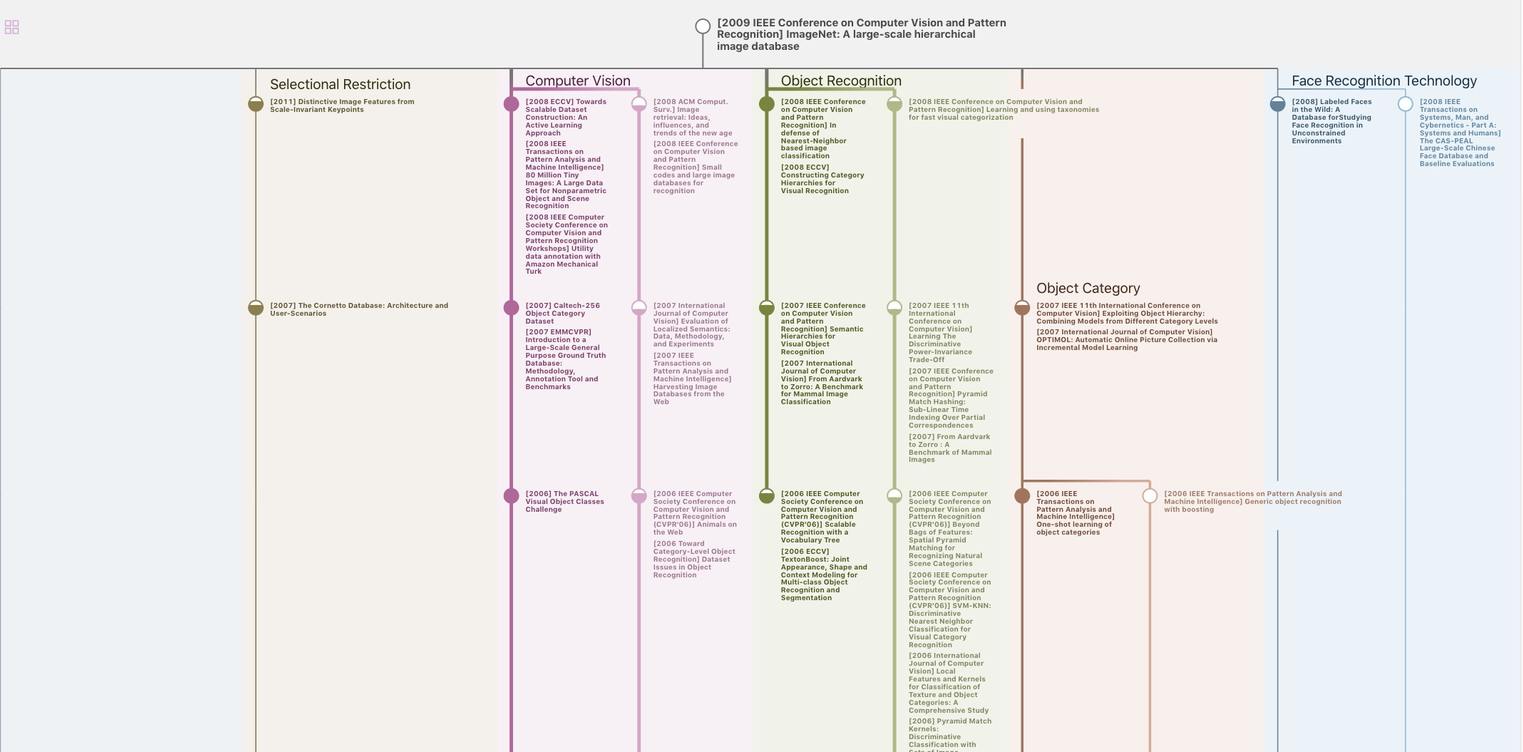
生成溯源树,研究论文发展脉络
Chat Paper
正在生成论文摘要