ISCLEs: Importance Sampled Circuit Learning Ensembles for Trustworthy Analog Circuit Topology Synthesis
EVOLVABLE SYSTEMS: FROM BIOLOGY TO HARDWARE, PROCEEDINGS(2008)
摘要
Importance Sampled Circuit Learning Ensembles (ISCLEs) is a novel analog circuit topology synthesis method that returns designer-trustworthy circuits yet can apply to a broad range of circuit design problems including novel functionality. ISCLEs uses the machine learning technique of boosting, which does importance sampling of “weak learners” to create an overall circuit ensemble. In ISCLEs, the weak learners are circuit topologies with near-minimal transistor sizes. In each boosting round, first a new weak learner topology and sizings are found via genetic programming-based “MOJITO” multi-topology optimization, then it is combined with previous learners into an ensemble, and finally the weak-learning target is updated. Results are shown for the trustworthy synthesis of a sinusoidal function generator, and a 3-bit A/D converter.
更多查看译文
关键词
Genetic Program,Circuit Design,Analog Circuit,Weak Learner,Source Follower
AI 理解论文
溯源树
样例
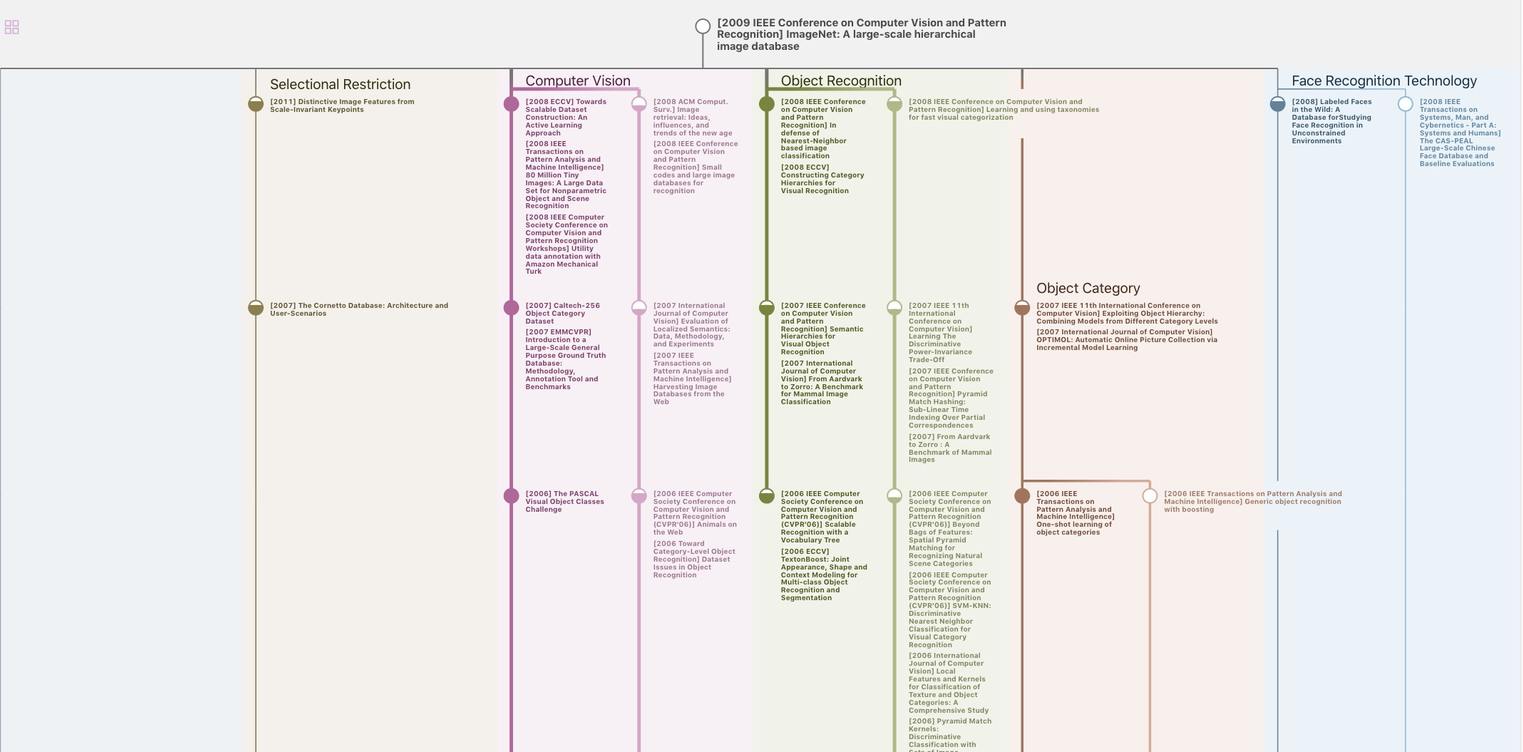
生成溯源树,研究论文发展脉络
Chat Paper
正在生成论文摘要