Transferring Instances For Model-Based Reinforcement Learning
MACHINE LEARNING AND KNOWLEDGE DISCOVERY IN DATABASES, PART II, PROCEEDINGS(2008)
摘要
Reinforcement learning agents typically require a significant amount of data before performing well on complex tasks. Transfer learning methods have made progress reducing sample complexity, but they have primarily been applied to model-free learning methods, not more data-efficient model-based learning methods. This paper introduces TIMBREL, a novel method capable of transferring information effectively into a model-based reinforcement learning algorithm. We demonstrate that TIMBREL can significantly improve the sample efficiency and asymptotic performance of a model-based algorithm when learning in a continuous state space. Additionally, we conduct experiments to test the limits of TMBREL's effectiveness.
更多查看译文
关键词
model-based algorithm,model-based reinforcement,sample complexity,sample efficiency,asymptotic performance,complex task,continuous state space,novel method,significant amount,Model-Based Reinforcement Learning,Transferring Instances
AI 理解论文
溯源树
样例
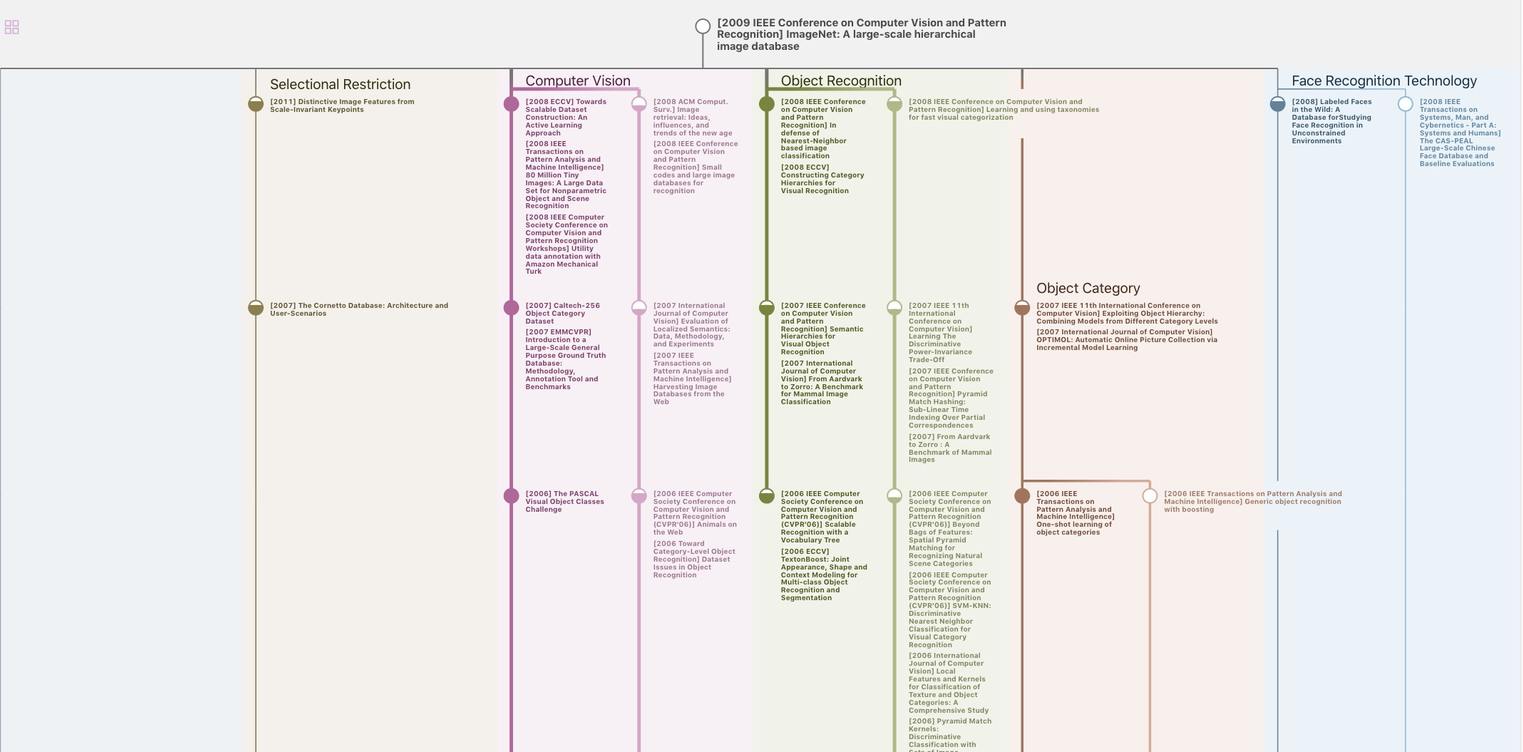
生成溯源树,研究论文发展脉络
Chat Paper
正在生成论文摘要