Semi-Supervised Classification From Discriminative Random Walks
MACHINE LEARNING AND KNOWLEDGE DISCOVERY IN DATABASES, PART I, PROCEEDINGS(2008)
摘要
This paper describes a novel technique, called D-walks, to tackle semi-supervised classification problems in large graphs. We introduce here a betweenness measure based on passage times during random walks of bounded lengths. Such walks are further constrained to start and end in nodes within the same class, defining a distinct betweenness for each class. Unlabeled nodes are classified according to the class showing the highest betweenness. Forward and backward recurrences are derived to efficiently compute the passage times. D-walks can deal with directed or undirected graphs with a linear time complexity with respect to the number of edges, the maximum walk length considered and the number of classes. Experiments on various real-life databases show the D-walks outperforms NetKit[5], the approach of Zhou and Scholkopf[15]and the regularized laplacian kernel[2]. The benefit of D-walks is particularly noticeable when few labeled nodes are available. The computation time of D-walks is also substantially lower in all cases.
更多查看译文
关键词
passage time,betweenness measure,distinct betweenness,highest betweenness,computation time,linear time complexity,Unlabeled node,bounded length,large graph,maximum walk length,Discriminative Random Walks,Semi-supervised Classification
AI 理解论文
溯源树
样例
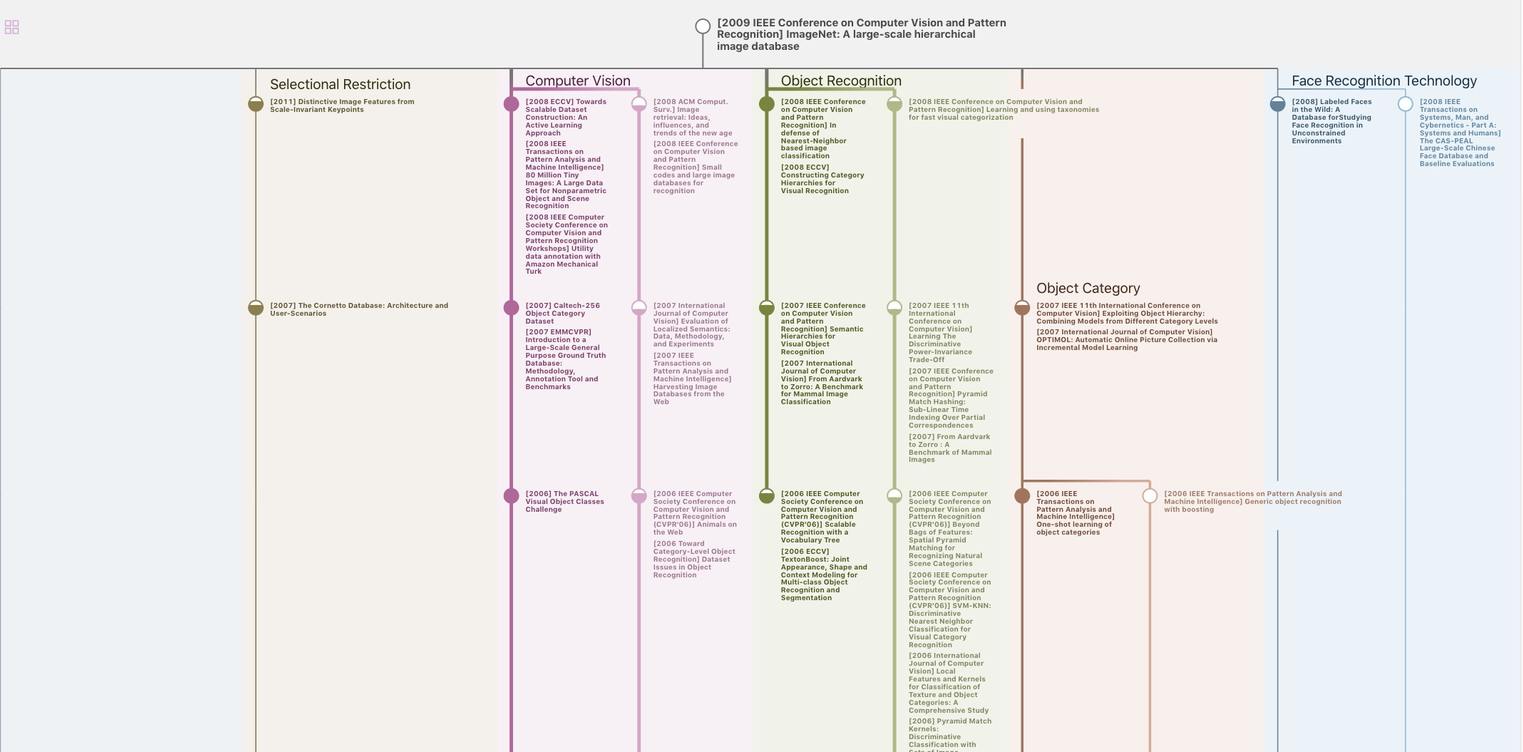
生成溯源树,研究论文发展脉络
Chat Paper
正在生成论文摘要