A Comparative Study Of Unsupervised Machine Learning And Data Mining Techniques For Intrusion Detection
MACHINE LEARNING AND DATA MINING IN PATTERN RECOGNITION, PROCEEDINGS(2007)
摘要
During the past number of years, machine learning and data mining techniques have received considerable attention among the intrusion detection researchers to address the weaknesses of knowledgebase detection techniques. This has led to the application of various supervised and unsupervised techniques for the purpose of intrusion detection. In this paper, we conduct a set of experiments to analyze the performance of unsupervised techniques considering their main design choices. These include the heuristics proposed for distinguishing abnormal data from normal data and the distribution of dataset used for training. We evaluate the performance of the techniques with various distributions of training and test datasets, which are constructed from KDD99 dataset, a widely accepted resource for IDS evaluations. This comparative study is not only a blind comparison between unsupervised techniques, but also gives some guidelines to researchers and practitioners on applying these techniques to the area of intrusion detection.
更多查看译文
关键词
unsupervised technique,intrusion detection,intrusion detection researcher,knowledgebase detection technique,abnormal data,data mining technique,normal data,KDD99 dataset,various distribution,IDS evaluation,Comparative Study,Data Mining Techniques,Intrusion Detection,Unsupervised Machine Learning
AI 理解论文
溯源树
样例
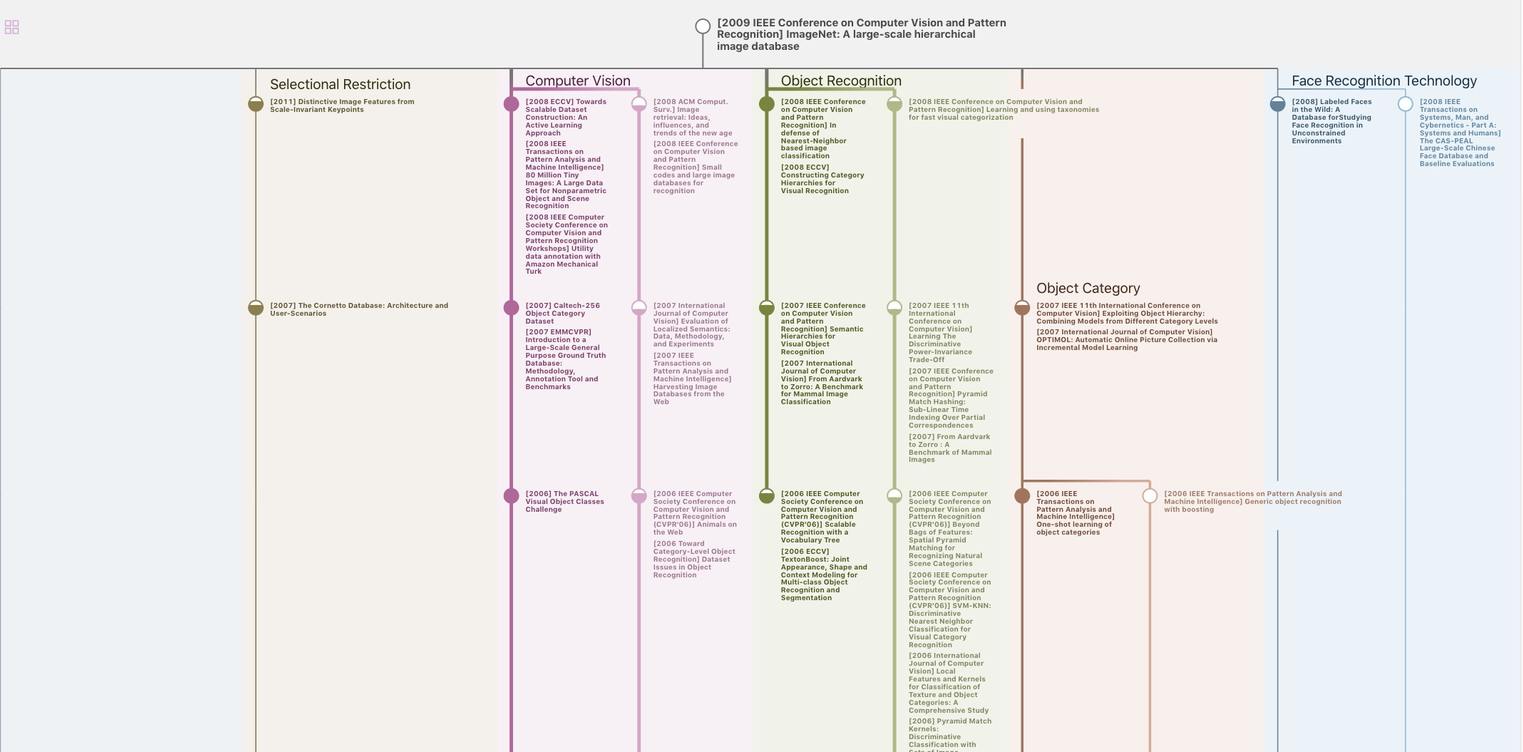
生成溯源树,研究论文发展脉络
Chat Paper
正在生成论文摘要