Correlating burst events on streaming stock market data
Data Mining and Knowledge Discovery(2007)
摘要
We address the problem of monitoring and identification of correlated burst patterns in multi-stream time series databases. We follow a two-step methodology: first we identify the burst sections in our data and subsequently we store them for easy retrieval in an efficient in-memory index. The burst detection scheme imposes a variable threshold on the examined data and takes advantage of the skewed distribution that is typically encountered in many applications. The detected bursts are compacted into burst intervals and stored in an interval index. The index facilitates the identification of correlated bursts by performing very efficient overlap operations on the stored burst regions. We present the merits of the proposed indexing scheme through a thorough analysis of its complexity. We also manifest the real-time response of our burst indexing technique, and demonstrate the usefulness of the approach for correlating surprising volume trading events using historical stock data of the NY stock exchange. While the focus of this work is on financial data, the proposed methods and data-structures can find applications for anomaly or novelty detection in telecommunication, network traffic and medical data.
更多查看译文
关键词
Time-series,Indexing,Burst detection,Correlation
AI 理解论文
溯源树
样例
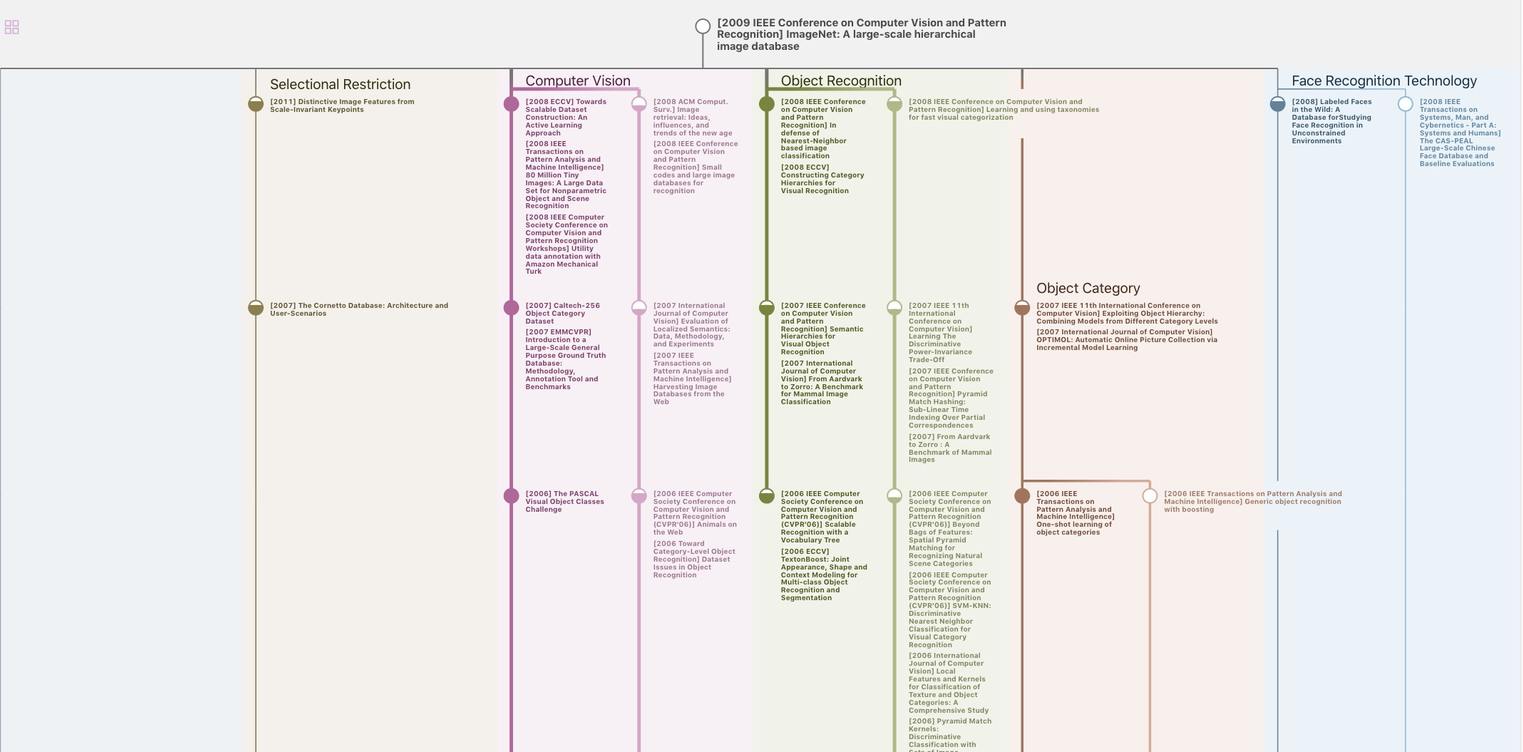
生成溯源树,研究论文发展脉络
Chat Paper
正在生成论文摘要