Learning to recognize features of valid textual entailments
HLT-NAACL '06: Proceedings of the main conference on Human Language Technology Conference of the North American Chapter of the Association of Computational Linguistics(2006)
摘要
This paper advocates a new architecture for textual inference in which finding a good alignment is separated from evaluating entailment. Current approaches to semantic inference in question answering and textual entailment have approximated the entailment problem as that of computing the best alignment of the hypothesis to the text, using a locally decomposable matching score. We argue that there are significant weaknesses in this approach, including flawed assumptions of monotonicity and locality. Instead we propose a pipelined approach where alignment is followed by a classification step, in which we extract features representing high-level characteristics of the entailment problem, and pass the resulting feature vector to a statistical classifier trained on development data. We report results on data from the 2005 Pascal RTE Challenge which surpass previously reported results for alignment-based systems.
更多查看译文
关键词
pascal rte challenge,pipelined approach,best alignment,textual inference,good alignment,entailment problem,alignment-based system,textual entailment,current approach,development data,valid textual entailment,feature vector,natural language,computational linguistics
AI 理解论文
溯源树
样例
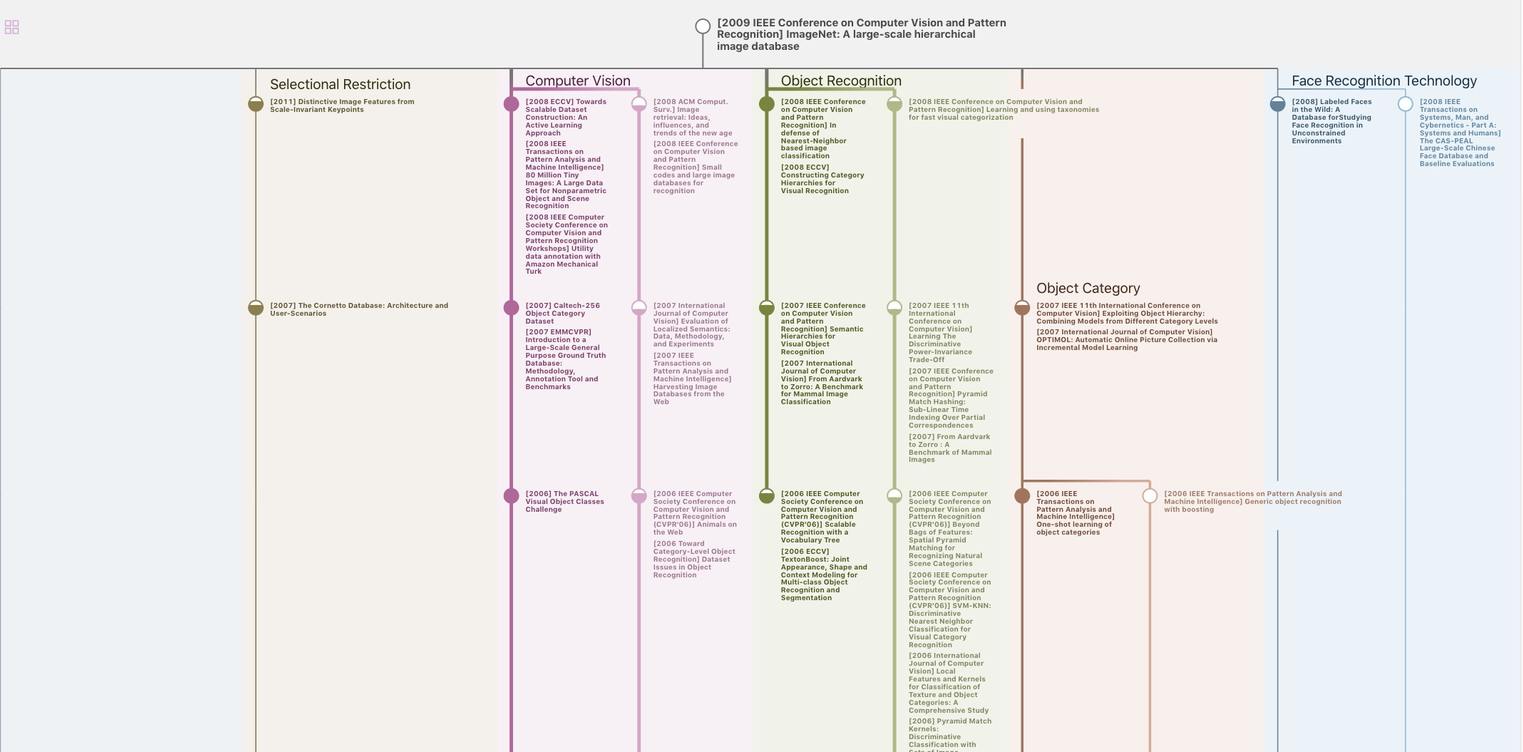
生成溯源树,研究论文发展脉络
Chat Paper
正在生成论文摘要