Resolution-Based Policy Search for Imperfect Information Differential Games
IAT '06 Proceedings of the IEEE/WIC/ACM international conference on Intelligent Agent Technology(2006)
摘要
Differential games (DGs), considered as a typical model of game with continuous states and non-linear dynamics, play an important role in control and optimization. Finding optimal/approximate solutions for these game in the imperfect information setting is currently a challenge for mathematicians and computer scientists. This article presents a multi-agent learning approach to this problem. We hence propose a method called resolution-based policy search, which uses a limited non-uniform discretization of a perfect information game version to parameterize policies to learn. We then study the application of this method to an imperfect information zero-sum pursuit-evasion game (PEG). Experimental results demonstrate strong performance of our method and show that it gives better solutions than those given by traditional analytical methods.
更多查看译文
关键词
perfect information game version,imperfect information,approximate solution,continuous state,differential game,zero-sum pursuit-evasion game,resolution-based policy search,computer scientist,better solution,imperfect information setting,imperfect information differential games,traditional analytical method,discretization,learning artificial intelligence,machine learning,non linear dynamics,multi agent systems
AI 理解论文
溯源树
样例
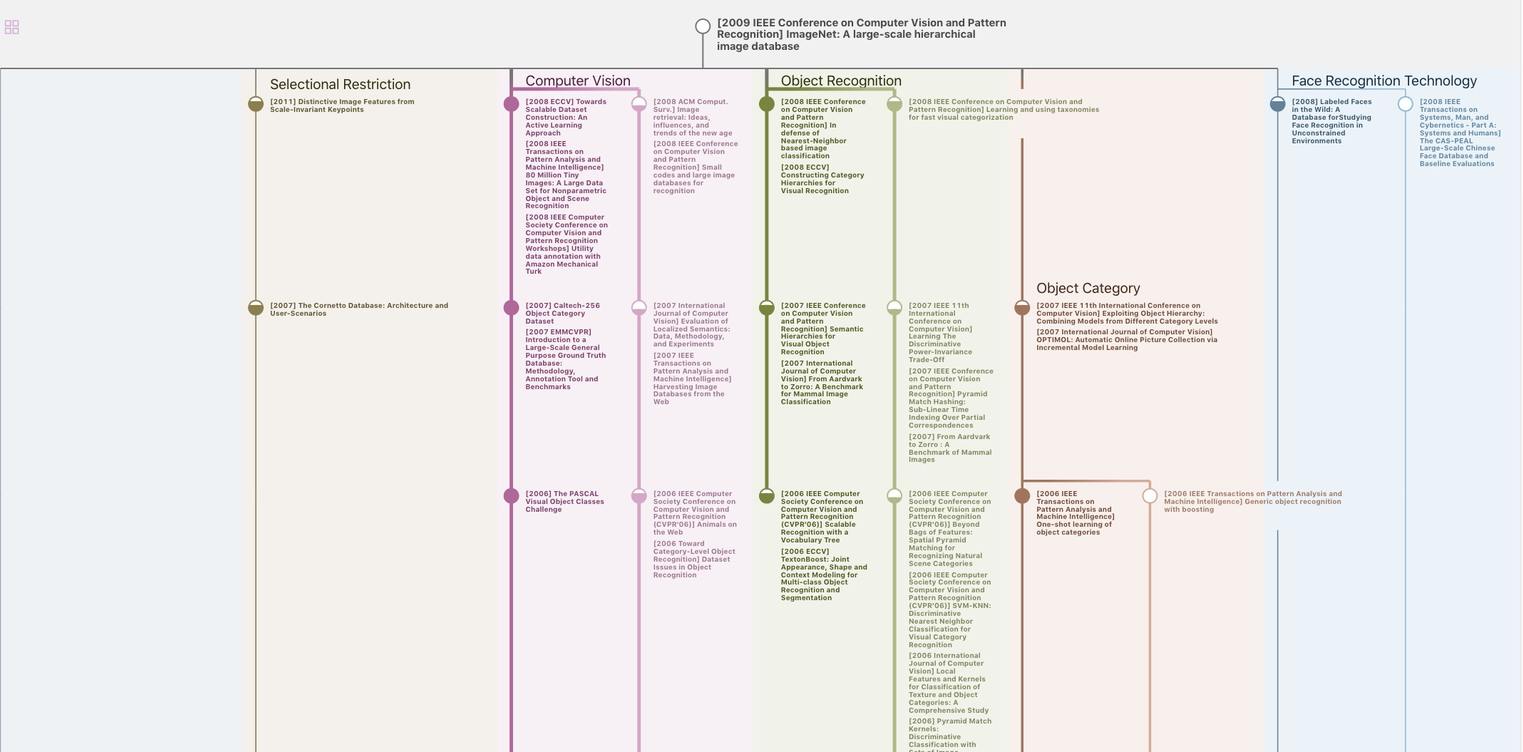
生成溯源树,研究论文发展脉络
Chat Paper
正在生成论文摘要