Nonlinear Dimension Reduction and Activation Detection for fMRI Dataset
CVPR Workshops(2006)
摘要
Functional magnetic resonance imaging (fMRI) has been established as a powerful method for brain mapping. Different physical phenomena contribute to the dynamical changes in the fMRI signal, the task-related hemodynamic responses, non-task-related physiological rhythms, machine and motion artifacts, etc. In this paper, we propose a new approach for fMRI data analysis. Each fMRI time series is viewed as a point in RT . We are interested in learning the organization of the points in high dimensions and extracting useful information for data classification. A nonlinear manifold learning technique is applied to obtain a low dimensional embedding of a dataset. The embedding differentiates time series with different temporal patterns. By assuming that the subset of activated time series forms a low dimensional structure, we partition the dataset and separate subsets of points with low dimensionality. The correspondence between low dimensional subsets and time series that contain task-related responses is verified and the activation maps are generated accordingly. The proposed approach is data-driven. It does not require a model for the hemodynamic response. We have conducted several experiments with synthetic and in-vivo datasets that demonstrate the performance of our approach.
更多查看译文
关键词
activated time series,embedding differentiates time series,fMRI data analysis,fMRI signal,fMRI time series,low dimensional,low dimensional structure,low dimensional subsets,low dimensionality,time series,Activation Detection,Nonlinear Dimension Reduction,fMRI Dataset
AI 理解论文
溯源树
样例
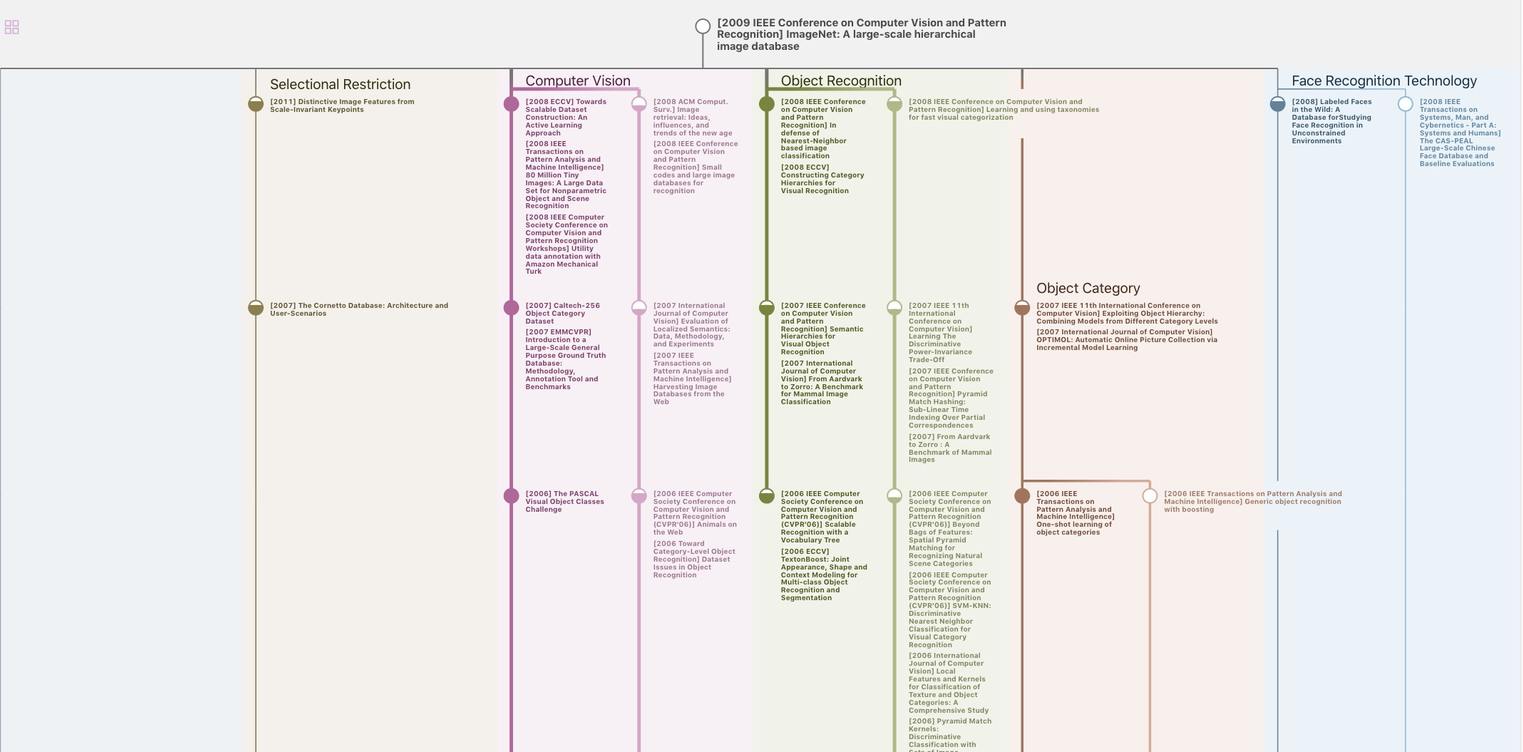
生成溯源树,研究论文发展脉络
Chat Paper
正在生成论文摘要