Predictive linear-Gaussian models of controlled stochastic dynamical systems
ICML(2006)
摘要
We introduce the controlled predictive linear-Gaussian model (cPLG), a model that uses predictive state to model discrete-time dynamical systems with real-valued observations and vector-valued actions. This extends the PLG, an uncontrolled model recently introduced by Rudary et al. (2005). We show that the cPLG subsumes controlled linear dynamical systems (LDS, also called Kalman filter models) of equal dimension, but requires fewer parameters. We also introduce the predictive linear-quadratic Gaussian problem, a cost-minimization problem based on the cPLG that we show is equivalent to linear-quadratic Gaussian problems (LQG, sometimes called LQR). We present an algorithm to estimate cPLG parameters from data, and show that our algorithm is a consistent estimation procedure. Finally, we present empirical results suggesting that our algorithm performs favorably compared to expectation maximization on controlled LDS models.
更多查看译文
关键词
expectation maximization,discrete time,dynamic system,consistent estimator,kalman filter,linear quadratic gaussian,linear dynamical system
AI 理解论文
溯源树
样例
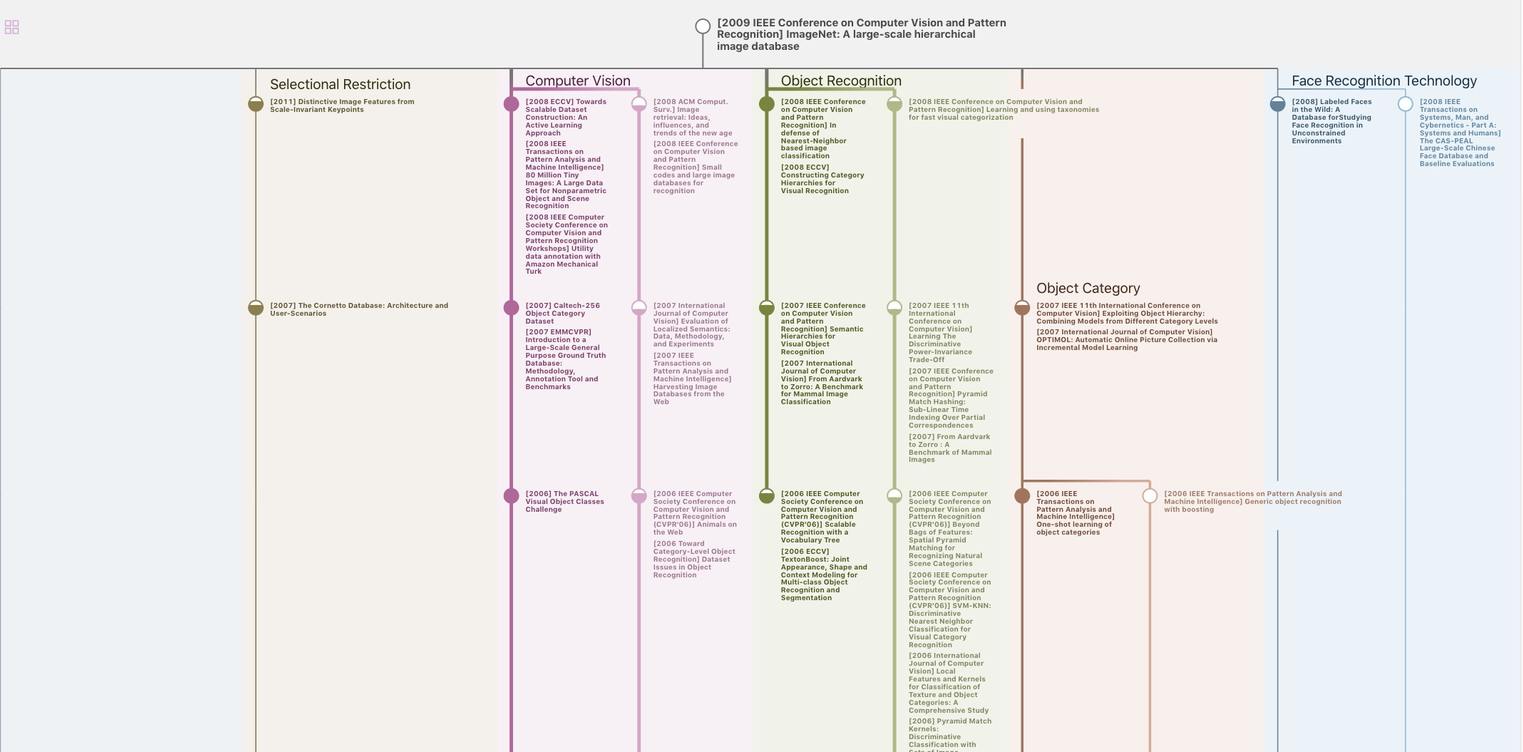
生成溯源树,研究论文发展脉络
Chat Paper
正在生成论文摘要