Hidden process models
ICML '06 Proceedings of the 23rd international conference on Machine learning(2006)
摘要
We introduce Hidden Process Models (HPMs), a class of probabilistic models for multivari- ate time series data. The design of HPMs has been motivated by the challenges of modeling hidden cognitive processes in the brain, given functional Magnetic Resonance Imaging (fMRI) data. fMRI data is sparse, high-dimensional, non-Markovian, and often involves prior knowl- edge of the form "hidden event A occurs n times within the interval (t,t0)." HPMs provide a gen- eralization of the widely used General Linear Model approaches to fMRI analysis, and HPMs can also be viewed as a subclass of Dynamic Bayes Networks.
更多查看译文
关键词
hidden process models,hidden cognitive process,functional magnetic resonance imaging,multivariate time series data,dynamic bayes networks,general linear model,fmri data,prior knowledge,n time,hidden process model,hidden event,probabilistic automata,paramagnetism,stimuli,algorithms,magnetic resonance imaging,monte carlo method,multivariate analysis,bayes theorem,diamagnetism,time series data,tracking,blood circulation,time series analysis,cognitive process,process model,probabilistic model,markov processes,cognition
AI 理解论文
溯源树
样例
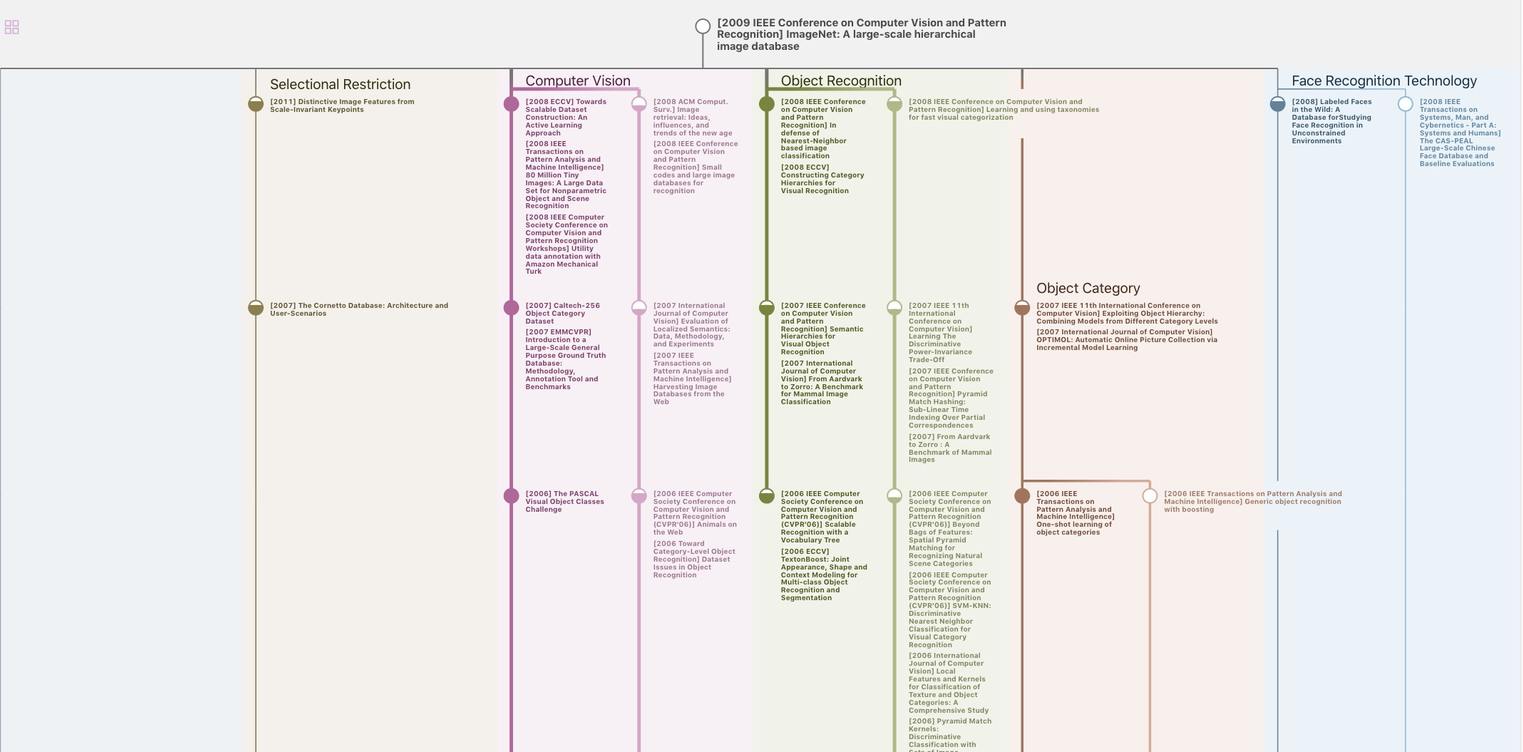
生成溯源树,研究论文发展脉络
Chat Paper
正在生成论文摘要