A constrained EM algorithm for independent component analysis.
Neural Computation(2001)
摘要
We introduce a novel way of performing independent component analysis using a constrained version of the expectation-maximization (EM) algorithm. The source distributions are modeled as D one-dimensional mixtures of gaussians. The observed data are modeled as linear mixtures of the sources with additive, isotropic noise. This generative model is fit to the data using constrained EM. The simpler "soft-switching" approach is introduced, which uses only one parameter to decide on the sub- or supergaussian nature of the sources. We explain how our approach relates to independent factor analysis.
更多查看译文
关键词
supergaussian nature,constrained em algorithm,d one-dimensional mixture,independent factor analysis,independent component analysis,generative model,linear mixture,source distribution,observed data,isotropic noise
AI 理解论文
溯源树
样例
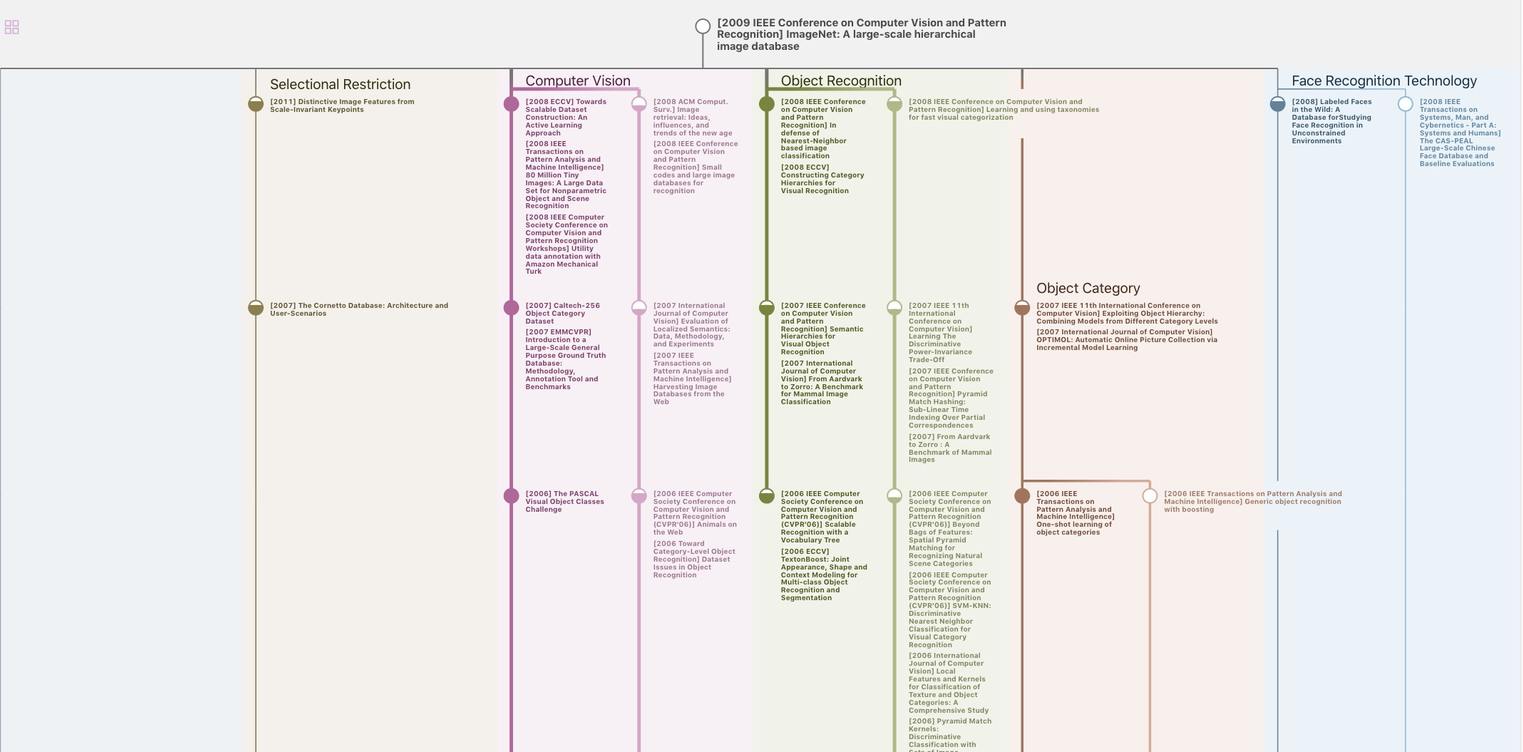
生成溯源树,研究论文发展脉络
Chat Paper
正在生成论文摘要