Bayesian neural network approaches to ovarian cancer identification from high-resolution mass spectrometry data.
BIOINFORMATICS(2005)
摘要
The classification of high-dimensional data is always a challenge to statistical machine learning. We propose a novel method named shallow feature selection that assigns each feature a probability of being selected based on the structure of training data itself. Independent of particular classifiers, the high dimension of biodata can be fleetly reduced to an applicable case for consequential processing. Moreover, to improve both efficiency and performance of classification, these prior probabilities are further used to specify the distributions of top-level hyperparameters in hierarchical models of Bayesian neural network (BNN), as well as the parameters in Gaussian process models.Three BNN approaches were derived and then applied to identify ovarian cancer from NCI's high-resolution mass spectrometry data, which yielded an excellent performance in 1000 independent k-fold cross validations (k = 2,...,10). For instance, indices of average sensitivity and specificity of 98.56 and 98.42%, respectively, were achieved in the 2-fold cross validations. Furthermore, only one control and one cancer were misclassified in the leave-one-out cross validation. Some other popular classifiers were also tested for comparison.The programs implemented in MatLab, R and Neal's fbm.2004-11-10.
更多查看译文
关键词
cancer identification,high-resolution mass spectrometry data,shallow feature selection,independent k-fold cross validation,leave-one-out cross validation,high-dimensional data,2-fold cross validation,excellent performance,bayesian neural network approach,bnn approach,ovarian cancer,training data,cross validation,feature selection,high dimensional data,neural network,mass spectrometry,high resolution,leave one out cross validation,hierarchical model,gaussian process
AI 理解论文
溯源树
样例
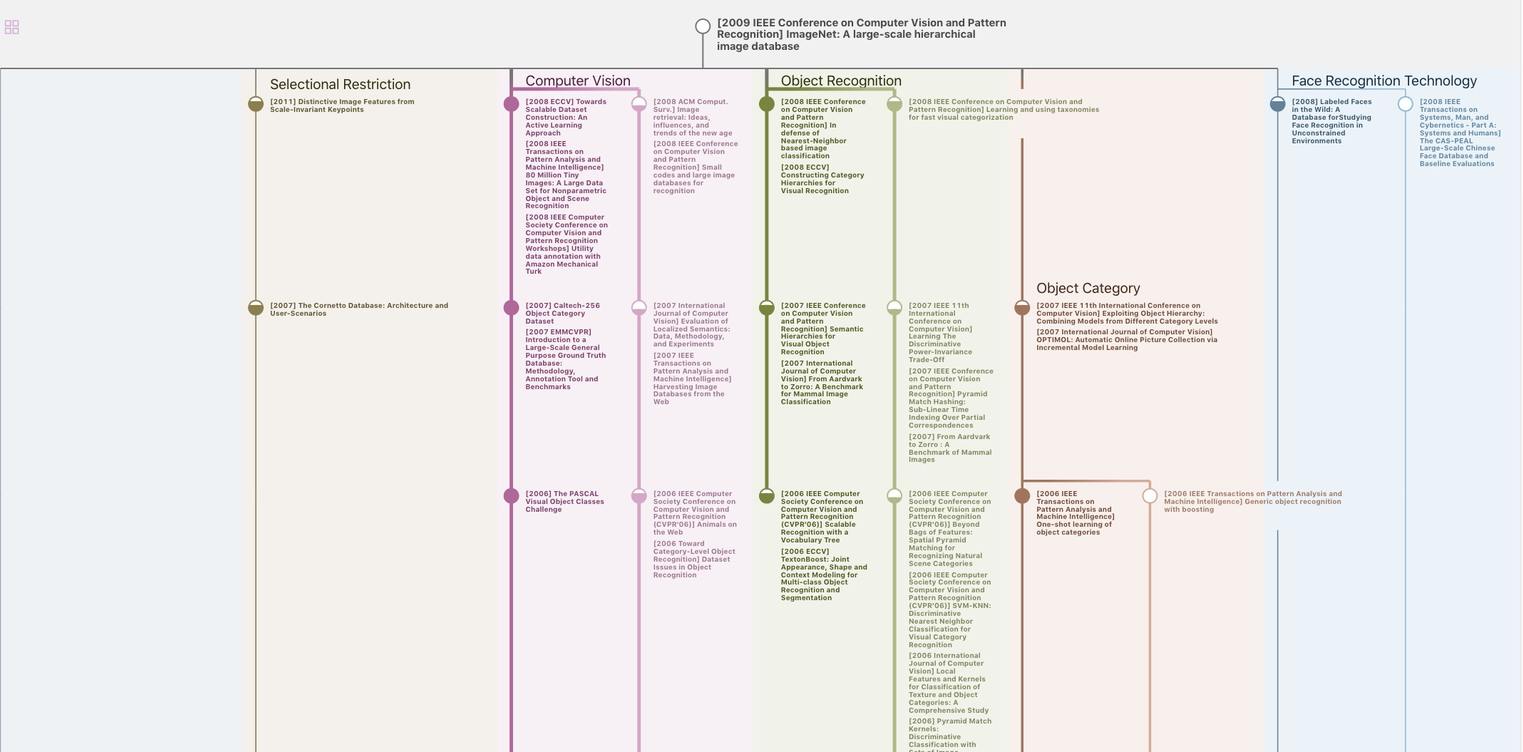
生成溯源树,研究论文发展脉络
Chat Paper
正在生成论文摘要