Diagram Structure Recognition by Bayesian Conditional Random Fields
Computer Vision and Pattern Recognition, 2005. CVPR 2005. IEEE Computer Society Conference(2005)
摘要
Hand-drawn diagrams present a complex recognition problem. Elements of the diagram are often individually ambiguous, and require context to be interpreted. We present a recognition method based on Bayesian conditional random fields (BCRFs) that jointly analyzes all drawing elements in order to incorporate contextual cues. The classification of each object affects the classification of its neighbors. BCRFs allow flexible and correlated features, and take both spatial and temporal information into account. BCRFs estimate the posterior distribution of parameters during training, and average predictions over the posterior for testing. As a result of model averaging, BCRFs avoid the overfitting problems associated with maximum likelihood training. We also incorporate Automatic Relevance Determination (ARD), a Bayesian feature selection technique, into BCRFs. The result is significantly lower error rates compared to ML- and MAP-trained CRFs.
更多查看译文
关键词
context modeling,computer vision,maximum likelihood,parameter estimation,learning artificial intelligence,maximum likelihood estimation,bayesian methods,feature extraction,feature selection,testing,uncertainty,conditional random field,pixel,posterior distribution,image recognition,labeling
AI 理解论文
溯源树
样例
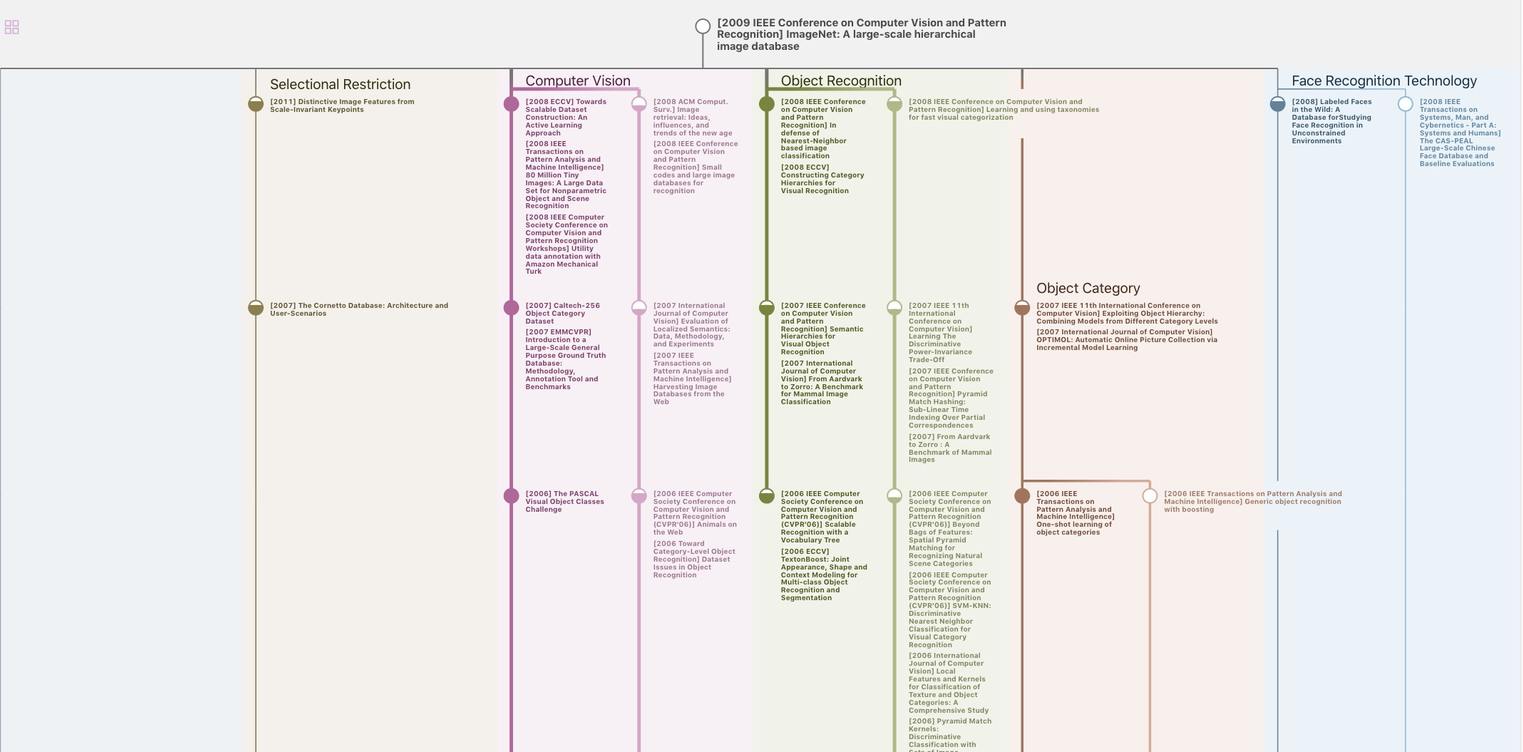
生成溯源树,研究论文发展脉络
Chat Paper
正在生成论文摘要