Discriminative Learning of Markov Random Fields for Segmentation of 3D Scan Data
Computer Vision and Pattern Recognition, 2005. CVPR 2005. IEEE Computer Society Conference(2005)
摘要
We address the problem of segmenting 3D scan data into objects or object classes. Our segmentation framework is based on a subclass of Markov Random Fields (MRFs) which support efficient graph-cut inference. The MRF models incorporate a large set of diverse features and enforce the preference that adjacent scan points have the same classification label. We use a recently proposed maximum-margin framework to discriminatively train the model from a set of labeled scans; as a result we automatically learn the relative importance of the features for the segmentation task. Performing graph-cut inference in the trained MRF can then be used to segment new scenes very efficiently. We test our approach on three large-scale datasets produced by different kinds of 3D sensors, showing its applicability to both outdoor and indoor environments containing diverse objects.
更多查看译文
关键词
markov random fields,trained mrf,segmentation framework,scan data,segmentation task,large set,diverse object,graph-cut inference,mrf model,maximum-margin framework,diverse feature,discriminative learning,efficient graph-cut inference,computer science,markov processes,image segmentation,feature extraction,image classification,learning artificial intelligence,mobile robots,testing,discrimination learning,graph cut,layout
AI 理解论文
溯源树
样例
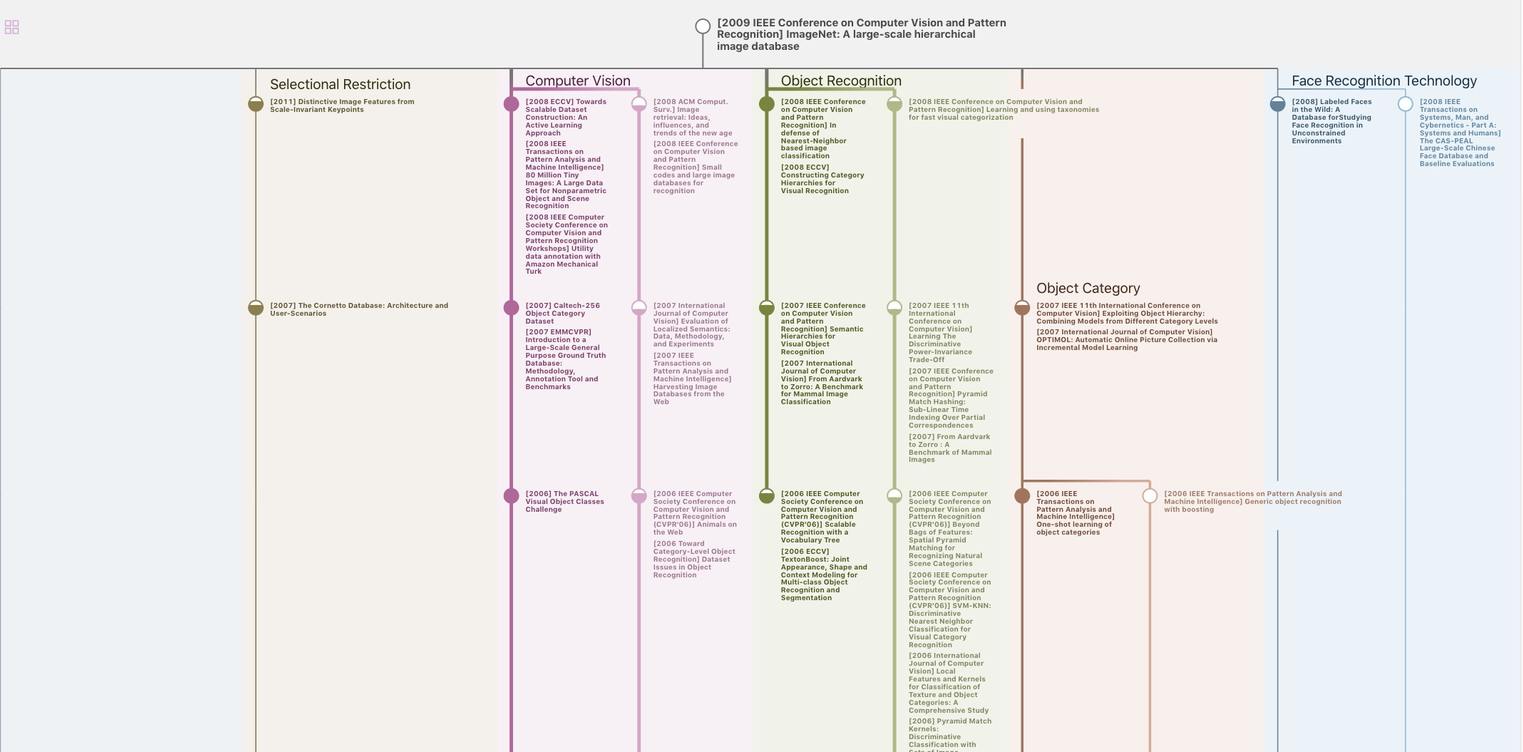
生成溯源树,研究论文发展脉络
Chat Paper
正在生成论文摘要