Generalization And Decision Tree Induction: Efficient Classification In Data Mining
RIDE '97 Proceedings of the 7th International Workshop on Research Issues in Data Engineering (RIDE '97) High Performance Database Management for Large-Scale Applications(1997)
摘要
Efficiency and scalability are fundamental issues concerning data mining in large databases. Although classification has been studied extensively, few of the known methods take serious consideration of efficient induction in large databases and the analysis of data at multiple abstraction levels. This paper addresses the efficiency and scalability issues by proposing a data classification method which integrates attribute-oriented induction, relevance analysis, and the induction of decision trees. Such an integration leads to efficient, high-quality, multiple-level classification of large amounts of data, the relaxation of the requirement of perfect training sets, and the elegant handling of continuous and noisy data.
更多查看译文
关键词
large databases,data classification method,data mining,noisy data,efficient induction,large amount,multiple level classification,multiple abstraction level,relevance analysis,scalability issue,decision tree induction,efficient classification
AI 理解论文
溯源树
样例
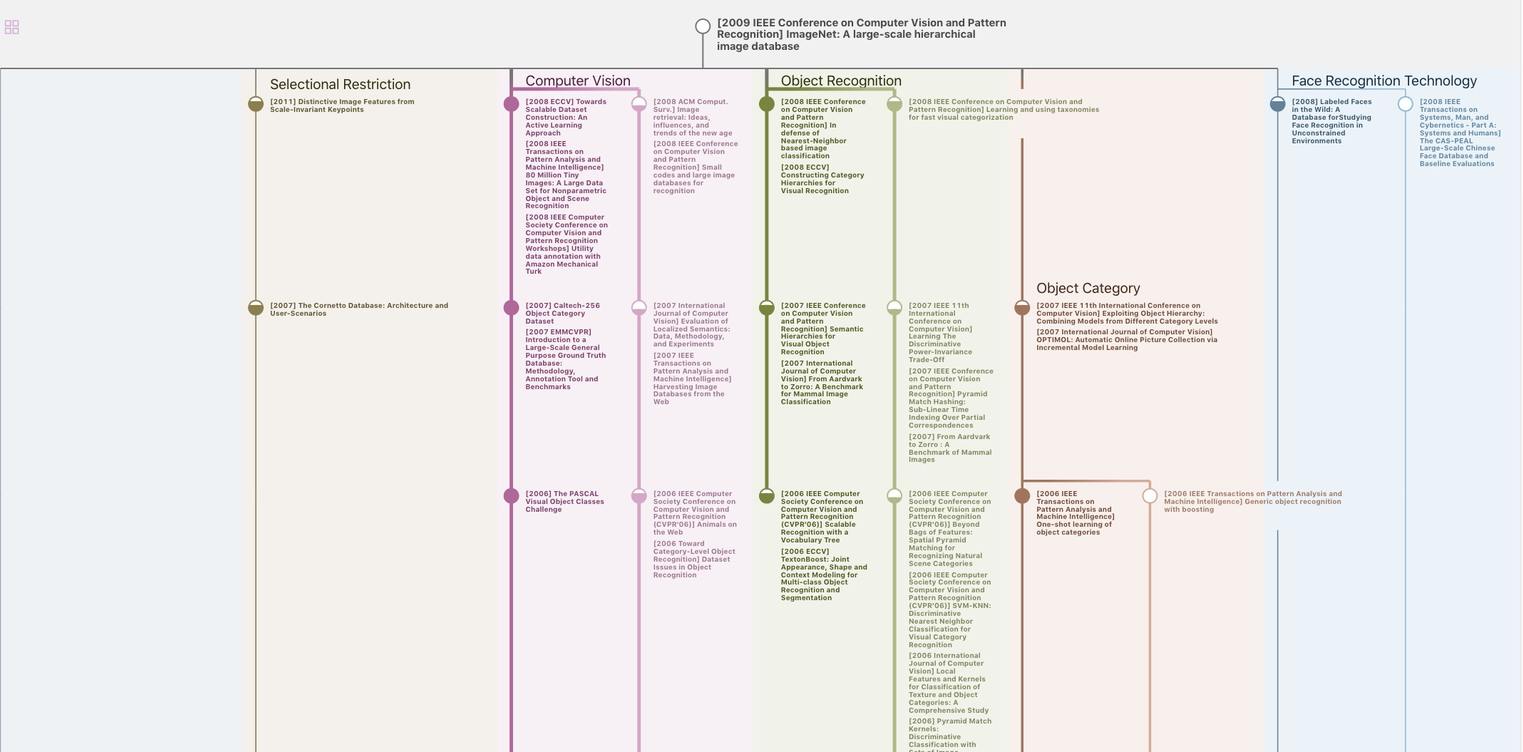
生成溯源树,研究论文发展脉络
Chat Paper
正在生成论文摘要